A SELF-NORMALIZED APPROACH TO SEQUENTIAL CHANGE-POINT DETECTION FOR TIME SERIES
Statistica Sinica(2019)
Abstract
We propose a self-normalization sequential change-point detection method for time series. To test for parameter changes, most traditional sequential monitoring tests use a cumulative sum-based test statistic, which involves a long-run variance estimator. However, such estimators require choosing a bandwidth parameter, which may be sensitive to the performance of the test. Moreover, traditional tests usually suffer from severe size distortion as a result of the slow convergence rate to the limit distribution in the early monitoring stage. We propose self-normalization method to address these issues. We establish the null asymptotic and the consistency of the proposed sequential change-point test under general regularity conditions. Simulation experiments and an applications to railway-bearing temperature data illustrate and verify the proposed method.
MoreTranslated text
Key words
ARMA-GARCH model,on-line detection,pairwise likelihood,quickest detection,sequential monitoring,stochastic volatility model
求助PDF
上传PDF
View via Publisher
AI Read Science
Must-Reading Tree
Example
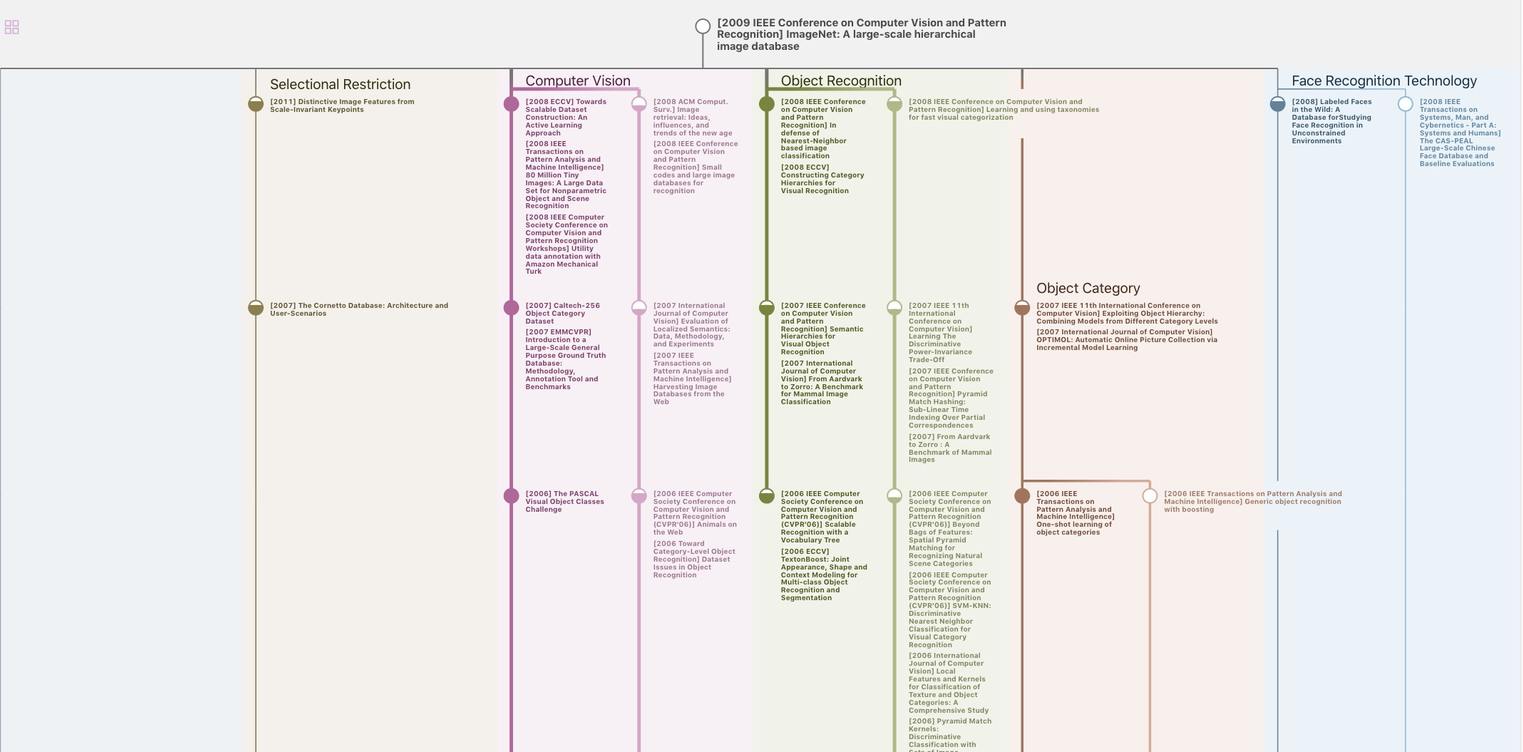
Generate MRT to find the research sequence of this paper
Data Disclaimer
The page data are from open Internet sources, cooperative publishers and automatic analysis results through AI technology. We do not make any commitments and guarantees for the validity, accuracy, correctness, reliability, completeness and timeliness of the page data. If you have any questions, please contact us by email: report@aminer.cn
Chat Paper
Summary is being generated by the instructions you defined