Collecting High Quality
semanticscholar(2010)
摘要
This paper studies quality of human labels used to train search engines’ rankers. Our specific focus is performance improvements obtained by using overlapping relevance labels, which collecting multiple human judgments for each training sample. The paper explores whether, when, and for which should obtain overlapping training labels, as well as labels per sample are needed. The proposed scheme collects additional labels only for a subset of training samples, specifically for those that are labeled relevant by a Our experiments show that this labeling schem NDCG of two Web search rankers on several real with a low labeling overhead of around 1.4 labels per sample This labeling scheme also outperforms several overlapping labels, such as simple k-overlap, majority vote, the highest labels, etc. Finally, the paper presents a study of how many overlapping labels are needed to get the best in retrieval accuracy.
更多查看译文
AI 理解论文
溯源树
样例
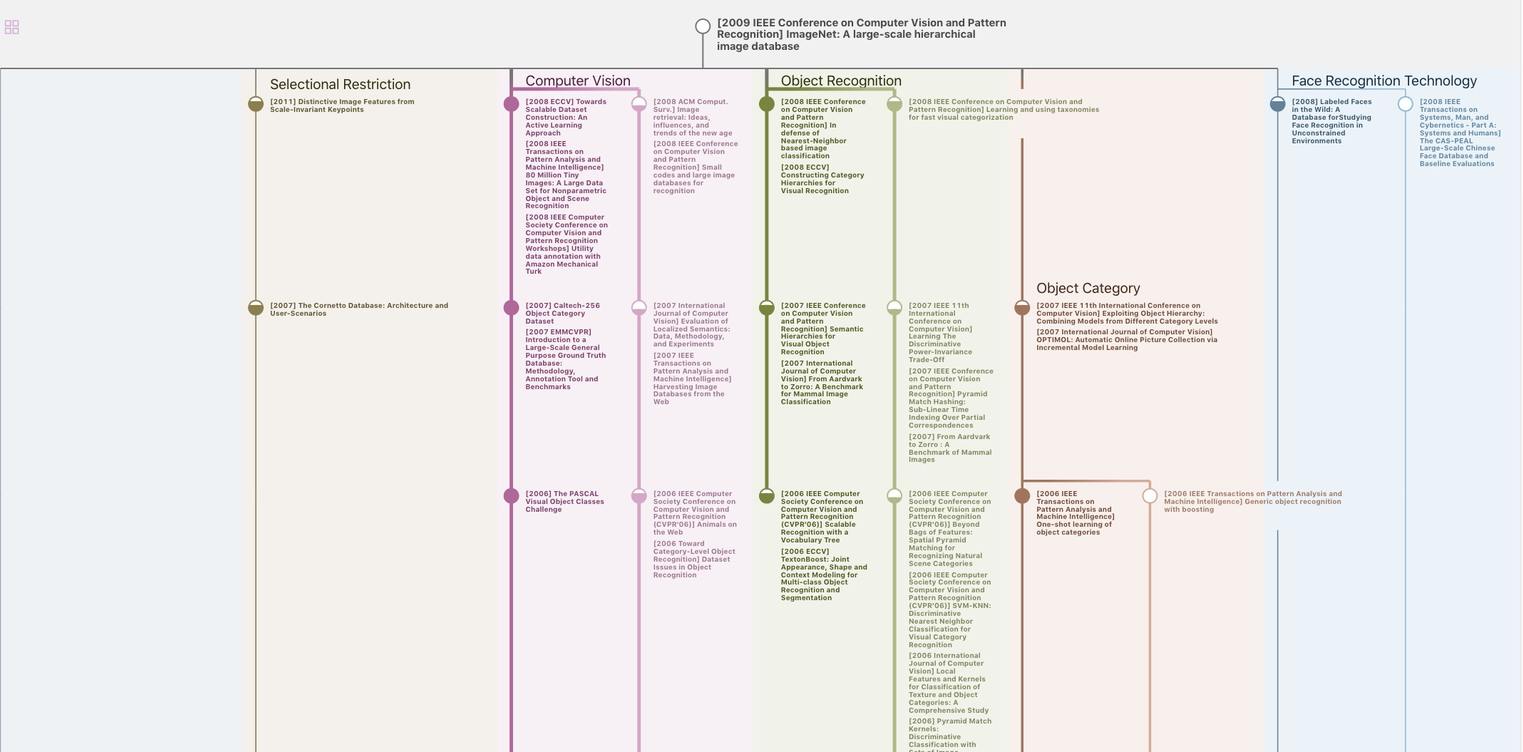
生成溯源树,研究论文发展脉络
Chat Paper
正在生成论文摘要