Know When To Abstain: Calibrating Question Answering System under Domain Shift
semanticscholar(2020)
摘要
No one can be an expert on everything. The same is true for Question Answering (QA) systems. During the interaction with users, it is essential for QA systems to correctly understand when it should refrain from giving the answer when it is likely to err. This motivates the problem of confidence modeling: if we can know when a model is unconfident, we can choose to reject its answer, making the model more robust and practical in real-world settings. In this project, we focus on confidence modeling of QA systems under domain shift. Our experiments show that when facing out-of-domain (OOD) questions, QA models can often be over-confident (i.e.: high softmax probability, which is served as our baseline) on incorrect answers. To tackle this problem, we propose a systematic approach to calibrate the model by augmenting it with a calibrator trained on a small subset of out-of-domain examples. And experiments have demonstrated that our calibrator has better modeling of the confidence score, mitigating the issue of out-of-domain over-confidence.
更多查看译文
AI 理解论文
溯源树
样例
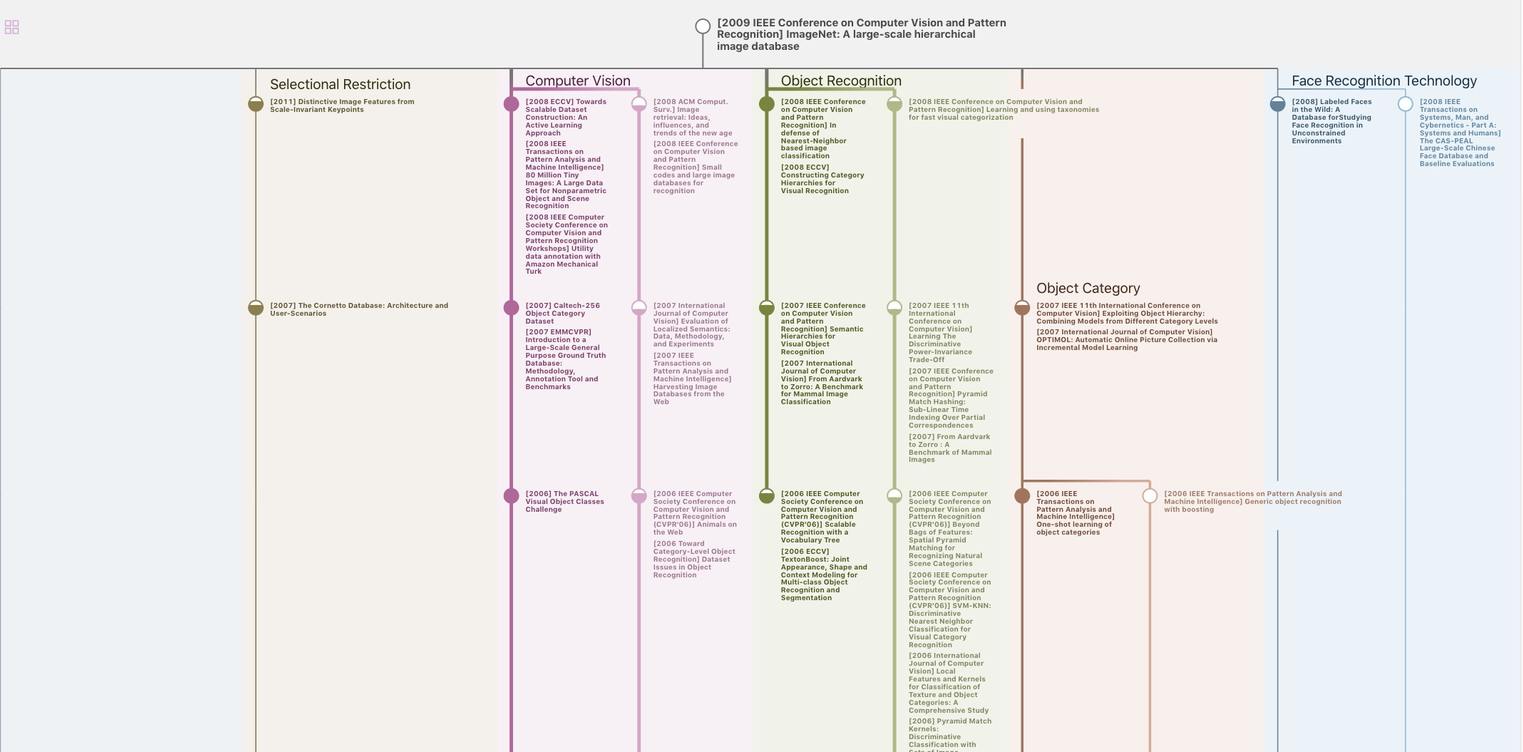
生成溯源树,研究论文发展脉络
Chat Paper
正在生成论文摘要