BAYESIAN AGGREGATION OF AVERAGE DATA By
semanticscholar(2017)
摘要
How can we combine datasets collected under different conditions? This is the well-known problem of meta-analysis, for which Bayesian methods have long been used to achieve partial pooling. Here we consider the challenge when one dataset is given as raw data while the second dataset is given as averages only. In such a situation, common meta-analytic methods can only be applied when the model is sufficiently simple for analytic approaches. When the model is too complex, for example nonlinear, an analytic approach is not possible. The need for meta-analytic methods applied to complex models arises frequently in clinical drug development. Throughout the different phases of a drug development program, randomized trials are used to establish in stages the tolerability, safety, and efficacy of a candidate drug. At each stage one aims to optimize the design of future studies by extrapolation from the available evidence at the time. This includes collected trial data and relevant external data. However, relevant external data are typically available as averages only, for example from trials on alternative treatments reported in the literature. Moreover, realistic models suitable for the desired extrapolation are often complex (longitudinal and nonlinear). We provide a Bayesian solution by using simulation to approximately reconstruct the likelihood of the external summary and allowing the parameters in the model to vary under the different conditions. We first evaluate our approach using fake-data simulations and then demonstrate its application to the problem in drug development that motivated this research, a hierarchical nonlinear model in pharmacometrics, implementing the computation in Stan.
更多查看译文
AI 理解论文
溯源树
样例
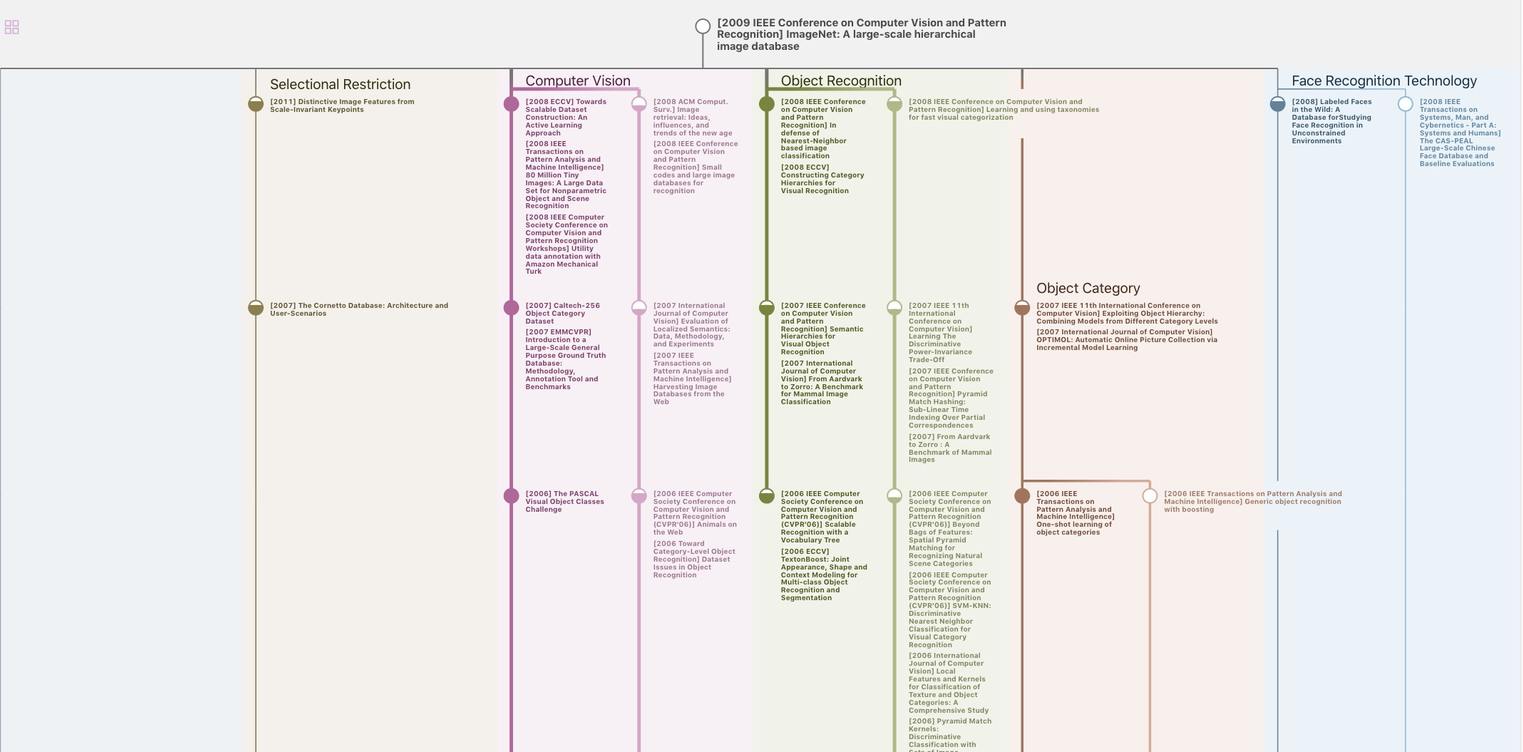
生成溯源树,研究论文发展脉络
Chat Paper
正在生成论文摘要