Robustness using input gradients
semanticscholar(2019)
摘要
This paper addresses the robustness of deep neural networks (DNNs) in respect of the network input. When imposing an input perturbation by an adversarial attack, it is hard to tell which pixels in the input are weak to the adversarial perturbation. We conjecture that the pixels with large expected input gradient are common weak points regardless of the input images. Based on our observation, we propose a simple module referred to Pixel Robustness Manipulator (PRM). By adding a PRM module as the first layer of a base network, the pre-determined (or planned) pixels become general weak points against adversarial attacks. This is done by inducing the adversarial perturbations to the predictable and interpretable locations by PRM, we can easily manage the location, where adversarial perturbations will affect on. Additionally, to show the effectiveness of PRM, we propose a simple defense strategy under a weak attack scenario, where the adversary knows the full parameters while has no information about the defense strategy.
更多查看译文
AI 理解论文
溯源树
样例
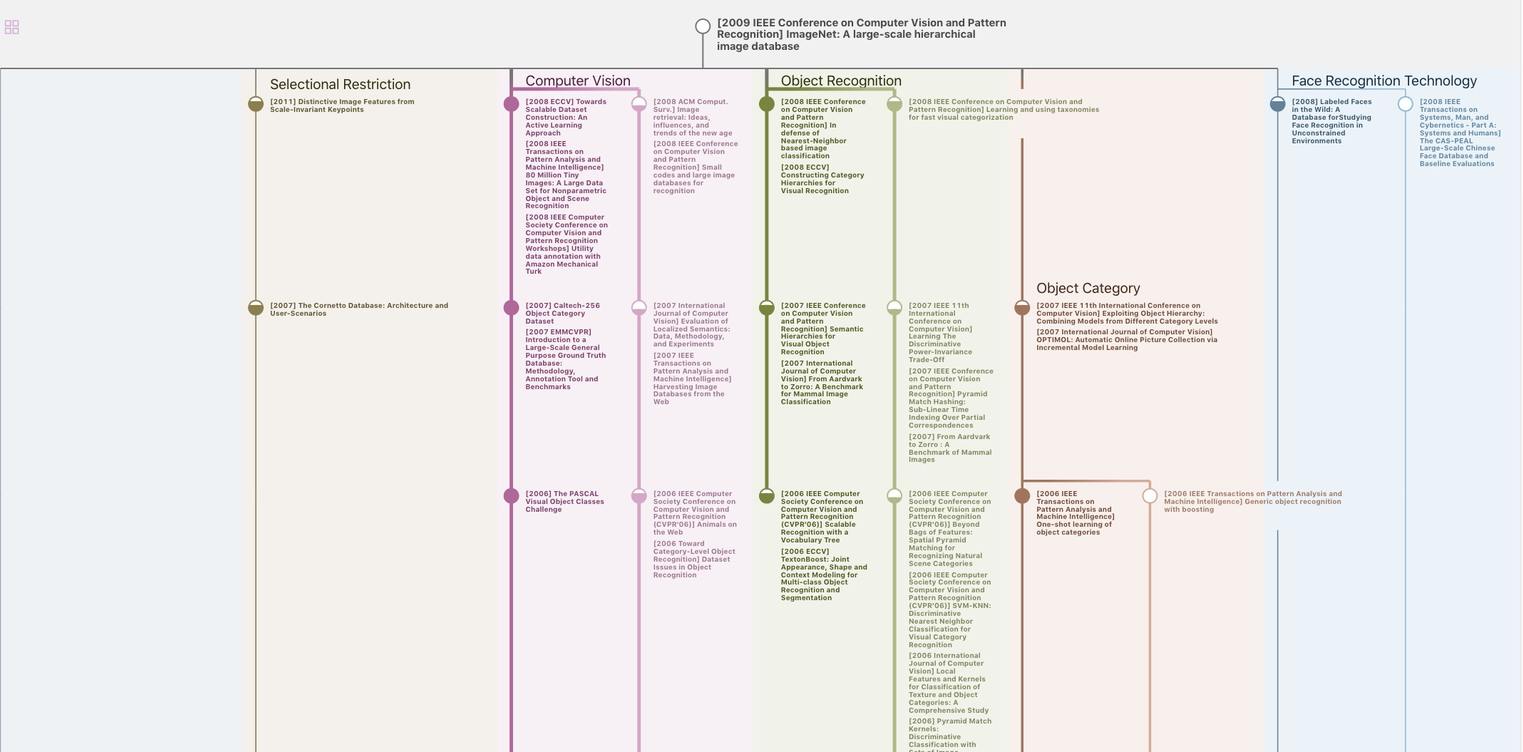
生成溯源树,研究论文发展脉络
Chat Paper
正在生成论文摘要