A Variational Bayes Approach to Robust Principal Component Analysis
semanticscholar(2013)
摘要
We solve the Robust Principal Component Analysis problem: decomposing an observed matrix into a low-rank matrix plus a sparse matrix. Unlike alternative methods that approximate this l0 objective with an l1 objective and solve a convex optimization problem, we develop a corresponding generative model and solve a statistical inference problem. The main advantages of this approach is its ability to incorporate additional prior information when it exists and cope with missing data where it does not. Using a variational Bayes approach, we develop an algorithm the low-rank and sparse matrices. Finally, we test and compare our Bayesian model with alternative approaches on both synthetic and real-world examples.
更多查看译文
AI 理解论文
溯源树
样例
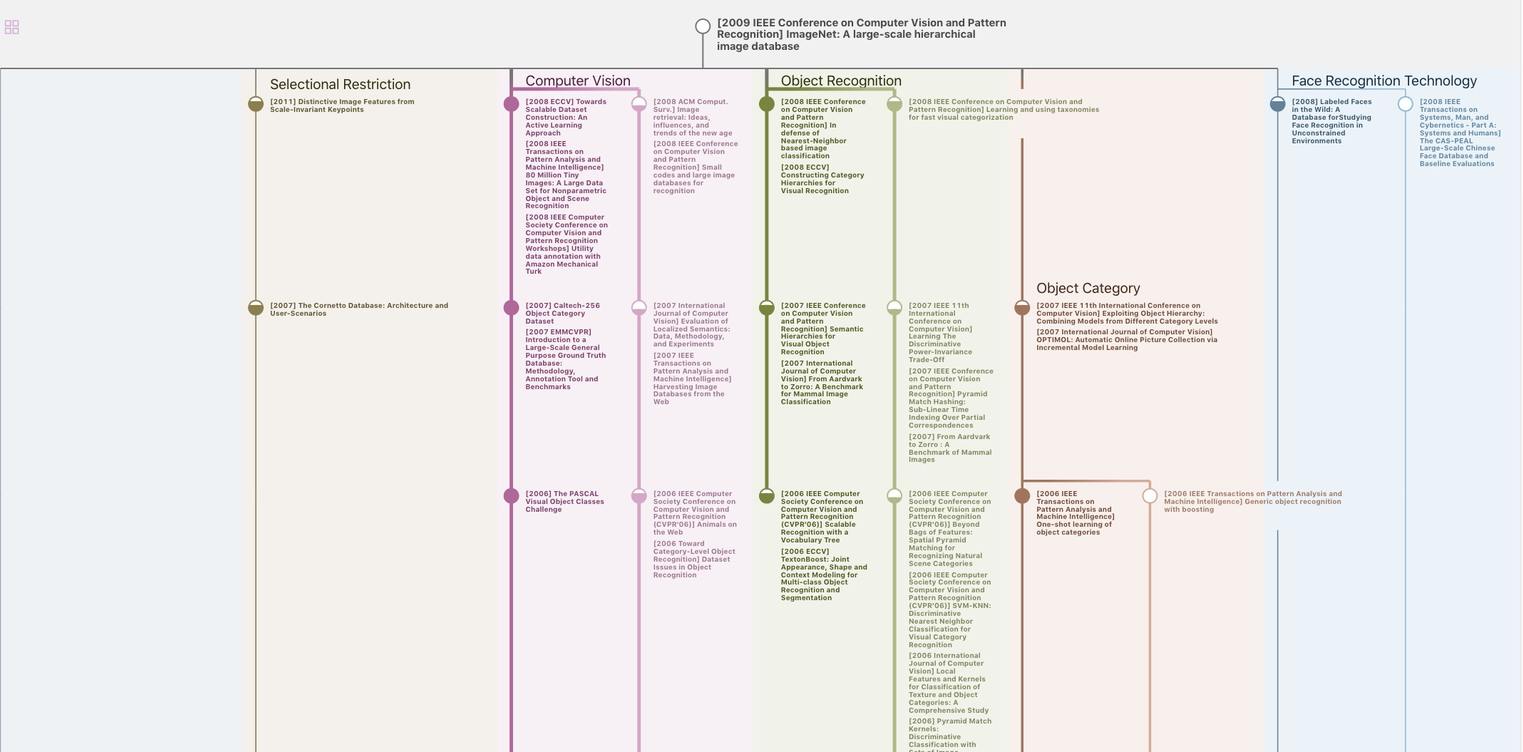
生成溯源树,研究论文发展脉络
Chat Paper
正在生成论文摘要