Visualization of Multivariate Data with Network Constraints using Multi-Objective Optimization
semanticscholar(2017)
摘要
Dimensionality reduction techniques play a key role in data processing and data visualization. Common dimensionality reduction techniques such as MDS, PCA, etc. do a decent job in projecting high-dimensional data into lower dimensions. But in the case of network data, they simply ignore the relationship between nodes which might result in non-planar graphs with many intersecting edges. In this paper, we have tried to model dimensionality reduction for network data as a multi-objective optimization problem. We have tried to draw graph/network in lower dimensions such that planarity is maximized and stress function is minimized simultaneously. We have used two genetic algorithms namely, NSGA-II and NSGA-III. For them, both objectives are equally important and they optimize them together. These techniques return a set of non-dominated solutions represented by pareto-Optimal front. We observed that genetic algorithms outperformed MDS for some cases. In other cases, genetic algorithms gave solutions with significantly lower number of intersections for slight increase in stress value.
更多查看译文
AI 理解论文
溯源树
样例
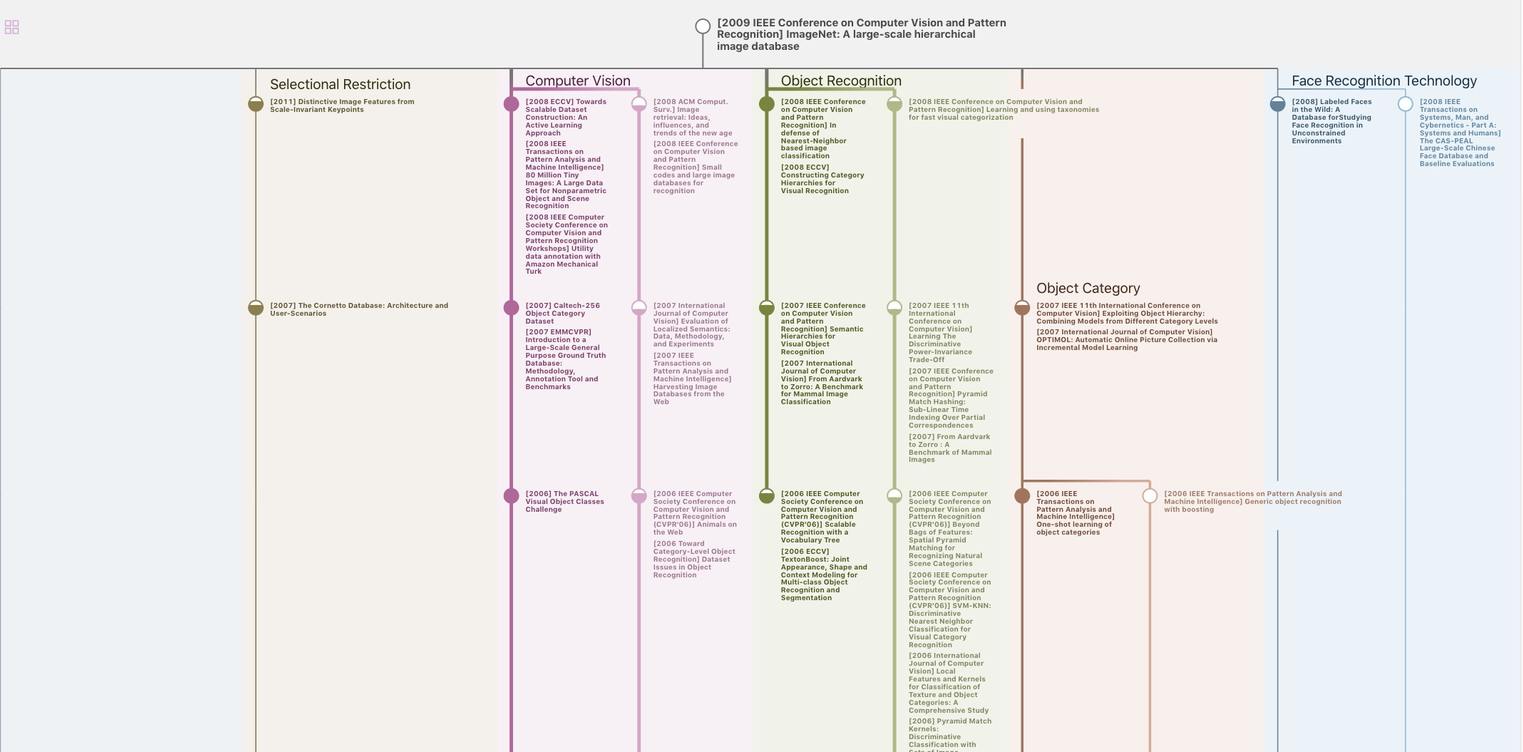
生成溯源树,研究论文发展脉络
Chat Paper
正在生成论文摘要