Scalable Clustering of Correlated Time Series using Expectation Propagation
SIGKDD Workshop on MiLeTS(2016)
摘要
We are interested in finding clusters of time series such that series within a cluster are correlated and series between clusters are independent. Existing Bayesian methods for inferring correlated clusters of time series either: (i) require conditioning on latent variables to decouple time series, but results in slow mixing or (ii) require calculating a collapsed likelihood, but with computation scaling cubically with the number of time series per cluster. To infer the latent cluster assignments efficiently, we consider approximate methods that trade exactness for scalability. Our main contribution is the development of an expectation propagation based approximation for the collapsed likelihood approach. Our empirical results on synthetic data show our methods scale linearly instead of cubically, while maintaining competitive accuracy.
更多查看译文
AI 理解论文
溯源树
样例
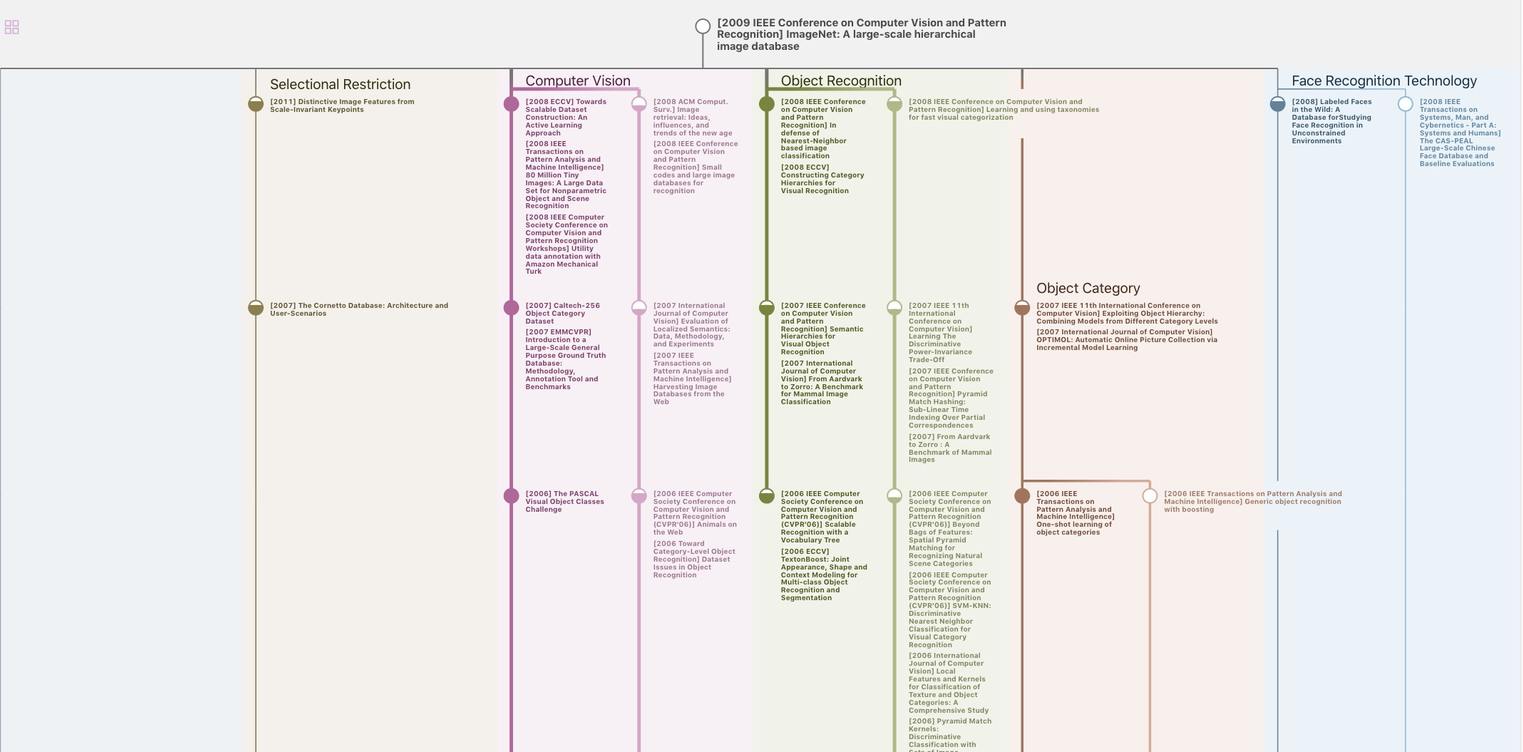
生成溯源树,研究论文发展脉络
Chat Paper
正在生成论文摘要