Drawing Out Model Based Inference : Simulation based plotting methods to discern and display replication from different sources
semanticscholar(2011)
摘要
It would seem prudent to attempt to recognize and visualize distinguishable components of evidence in any statistical analysis. Some methods are developed to facilitate this. One method provides marginal priors and marginal (integrated) likelihoods for a given foci (i.e. parameters of interest) in proper Bayesian analysis using MCMC. Another provides accurate almost individual observation likelihoods for Frequentist (profile and estimated) likelihood analysis. To provide an approximate display for a Bayesian analysis, these almost individual observation profile likelihoods are deformed so that they add up exactly to the integrated likelihood. The motivation is to enable analysts to easily see what is happening in complex Bayesian and or Frequency analyses even those analysts without advanced mathematical skills. In a fully Bayesian analysis the prior and data model (likelihood) evidence can trivially be recognized. Visualization, though, will be diffi cult with more than just a few parameters. Fortunately, these lower dimensional foci (marginal posteriors) can be split into marginal prior and marginal likelihood (Berger, et al 1999). The marginal posterior obtained from integrating the full dimensional posterior is proportional to the marginal (integrated) prior times the marginal (integrated) likelihood. In the Frequentist profile approach, the marginal (profile) likelihoods for foci are obtained by maximizing over the other parameters. This amounts to finding a particular path over the multivariate likelihood surface. This path provides an immediate route for obtaining contributions from individual observations that then can be modified to multiply to the integrated likelihood. They are called almost individual
更多查看译文
AI 理解论文
溯源树
样例
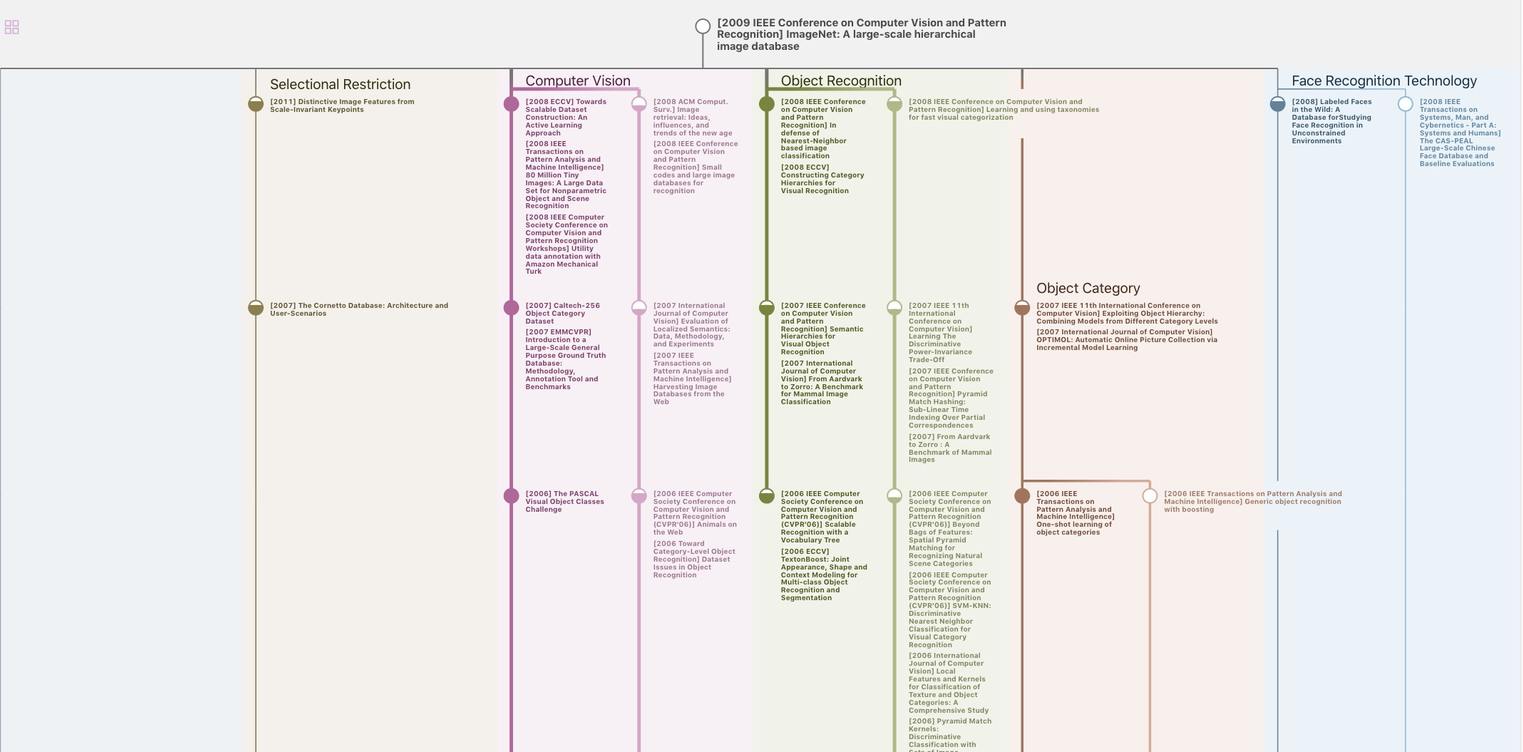
生成溯源树,研究论文发展脉络
Chat Paper
正在生成论文摘要