Actor-Critic Instance Segmentation – Supplemental Material –
semanticscholar(2019)
摘要
As we discussed in the main text, previous work suggests that the overall accuracy of a recurrent model is not invariant to the prediction order [20, 37]. To confirm this experimentally for instance segmentation, we decouple the localisation and the segmentation aspect of a recurrent model in an oracle experiment. Reserving the location of the groundtruth segments as the oracle knowledge (e.g., with a bounding box) allows us to control the prediction order and study its role for the quality of the pixelwise prediction. The setup for our oracle experiment is illustrated in Fig. 5. We supply the image and context patches (4 input channels) to a segmentation network in random order, while at the same time accumulating its predictions into the (global) context mask. We repeat this procedure 20 times for every image, with a different random order each. We use the CVPPP train/val split from our ablation study, and report the results on the 25 images in the validation set. The architecture of the network is the same as the segmentation module used by Ren and Zemel [31]. Figure 6a shows the statistic of the results (mean and standard deviation) for each of the 25 images. We observe that there is a tangible difference between the different segmentation orderings. The mean of the maximum and the minimum Dice across the images are 86.5 and 78.1 Dice, respectively, which corresponds to a potential gain of 8.4 mean Dice for the optimal prediction order. Notably, the deviation between the runs varies depending on the image. To investigate this further, we show two examples from the validation set with the highest (index #1, Fig. 6b) and the lowest (index #19, Fig. 6c) deviation. Example #1 appears to contain many more occlusions and more complex segment shapes (e.g., a single stalk) compared to example #19. This aligns well with our expectation: the benefit of the context should be more pronounced in more complex scenes.
更多查看译文
AI 理解论文
溯源树
样例
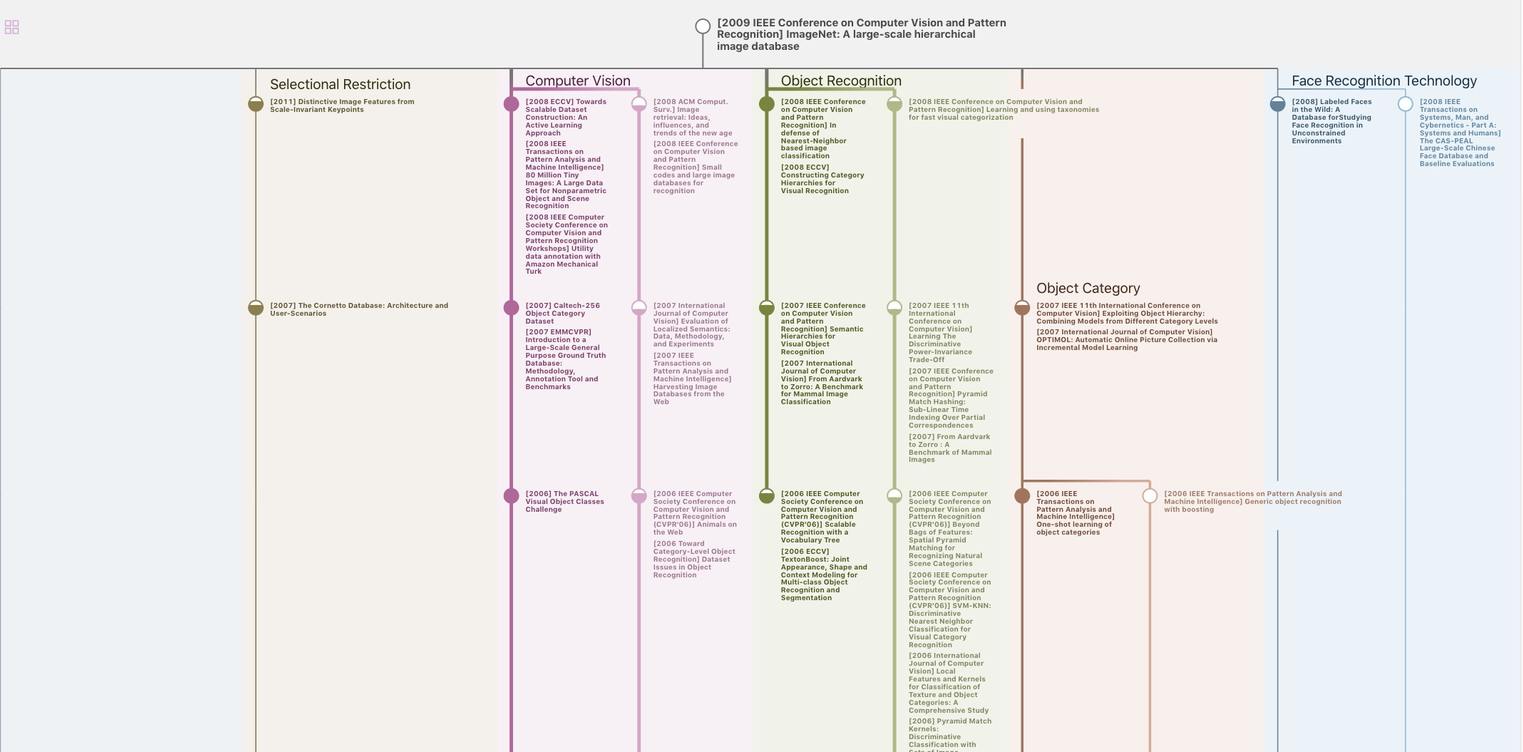
生成溯源树,研究论文发展脉络
Chat Paper
正在生成论文摘要