How to Separate Regular Prices from Promotional Prices ?
semanticscholar(2014)
摘要
Retail price setting is influenced by two distinct factors: the regular price and the promotion price. Together, these factors determine the list price for a specific item at a specific time. These data are often reported only as a singular list price. Separating this one price into two distinct prices is critical for accurate price elasticity modeling in retail. These elasticities are then used to make sales forecasts, manage inventory, and evaluate promotions. This paper describes a new time-series feature extraction utility within SAS Forecast Server that allows for automated separation of promotional and regular prices. INTRODUCTION Given a collection of time series of varying lengths, you can use time series segmentation methods to reduce the time dimension of each series by extracting important features. Time series segmentation aids in the following time series analyses: Decomposing time series: Given a single time series (original series), you can decompose the original series by determining the major (long-term) feature; you can then remove the major feature from the original series to obtain the minor (short-term) features. For example, this analysis is useful for decomposing regular and promotional prices where the original series is the sale price of a product, the major features are regular prices (long-term), and the minor features are the promotional prices (short-term). Customizing discrete time intervals: Given a single time series, you can differentiate time periods that have high and low activity in order to customize a time interval that makes the series easier to identify and easier to model for subsequent forecasting. For example, given a time series of a seasonal product has both in-season (high-activity) and off-season (low-activity) periods, it might beneficial to map the off-season period into a single time period. Analyzing turning points: Given a single time series, you can detect major change points from the time series. These change points might be useful for determining turning points in the series for subsequent turning point analysis. For example, turning point analysis is useful for forecasting the life cycle of products in the marketplace. Comparing time series: Given several time series of varying length, you can use fixed-length segmentation to extract the major features. Comparing fixed-length data vectors is far easier than comparing variablelength data vectors. For example, this analysis is useful for time series search and retrieval, ranking, clustering, and other data mining analyses that are related to time series. Visualizing time series: Given a long time series, it might be difficult to perceive which major features are associated with the time series when you view a typical (detailed) time series plot. If you extract and display only the major features, you might be able to understand the time series when you view it with less detail. SCOPE This paper focuses on (discrete) time series segmentation of time-stamped data that are generated by business and economic activity. In particular, this paper focuses on price series decomposition. The techniques described in this paper are less applicable to voice, image, multimedia, medical, and scientific data or to continuous-time data. BACKGROUND This section provides a brief theoretical background on time series segmentation analysis. It is intended to provide the analyst with motivation, orientation, and references. For an introductory discussion of these topics, see Keogh et al. (2004).
更多查看译文
AI 理解论文
溯源树
样例
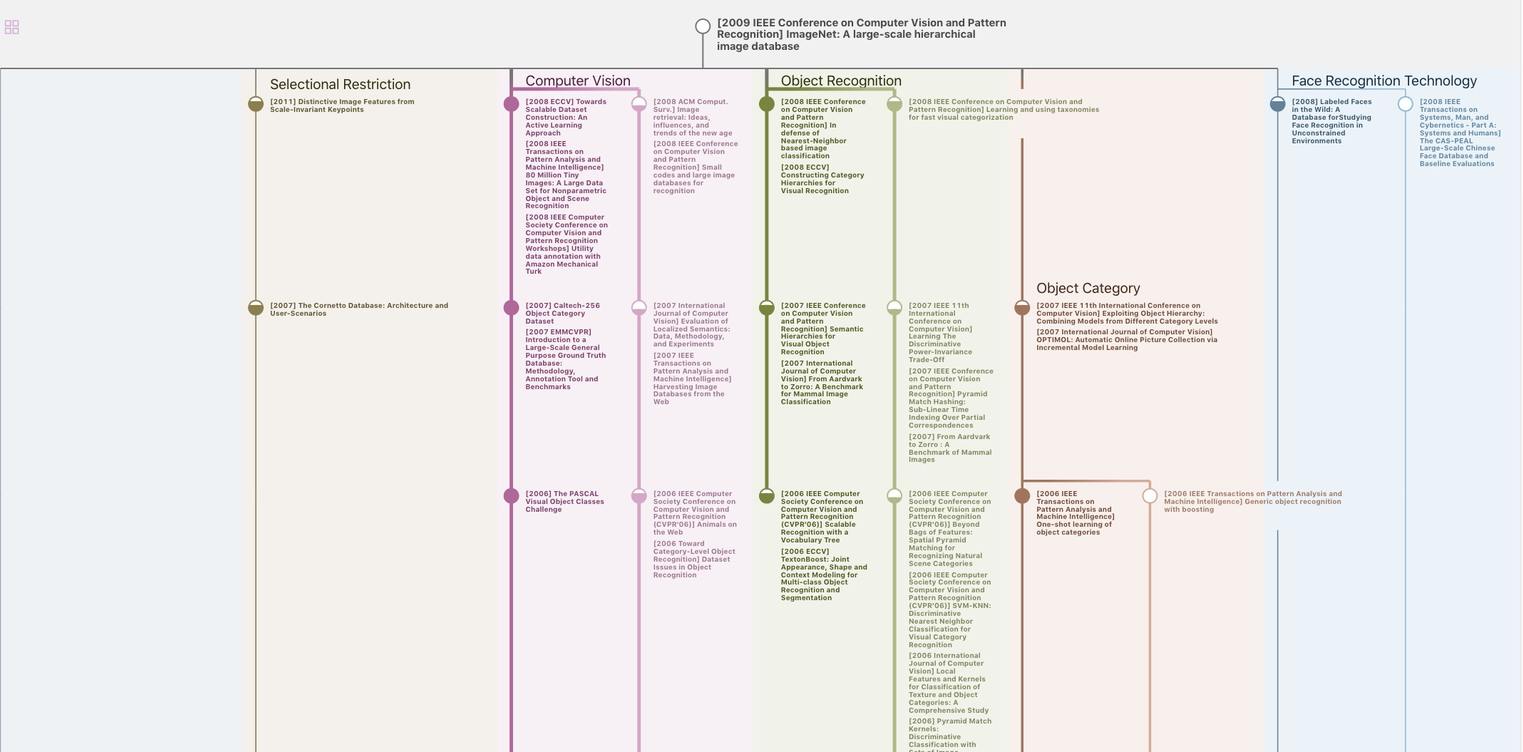
生成溯源树,研究论文发展脉络
Chat Paper
正在生成论文摘要