Poster: Membership Inference Against DNA Methylation Databases: Attacks and Defenses
2020 IEEE European Symposium on Security and Privacy (EuroS&P)(2019)
摘要
Access to biomedical data is key for the advancement of biomedical research. However, biomedical data raises new privacy concerns: membership inference attacks against a biomedical database can leak sensitive information, such as the participants’ health status. In this paper, we study membership inference attacks on DNA methylation data, one of the most important epigenetic elements regulating the human health. We design three different types of attacks exploiting published summary statistics. Two of them are based on statistical tests and the third one on a machine learning model. Moreover, we exploit the dependencies between genome and methylation data to replace knowledge of the target’s methylome by knowledge of the target’s genome, which is currently more accessible. Our extensive evaluation shows that such membership inference attacks are effective. In order to mitigate these privacy risks, we rely on differential privacy and show that such defense is able to provide strong privacy guarantees at the cost of a significantly decreased utility. By restraining the number of released methylation regions to a few hundreds, we can reach an acceptable level of privacy without sacrificing all statistical utility. With the rapidly decreasing costs of molecular profiling, the types of available biomedical data are increasingly diverse and go beyond the genomes of individuals. DNA methylation is one of the most important new types of biomedical data. Being a key regulator of gene transcription, abnormal methylation patterns can lead to severe diseases, such as cancer [2]. Moreover, DNA methylation is also related to environmental cues, such as pollution, exposure to stress or cigarette smoke [8], [9], [1]. Despite being linked with such sensitive information, DNA methylation data is already available on various open research platforms, such as the Gene Expression Omnibus (GEO) [4]. Contrary to genomic data whose privacy has been extensively studied by the security research community [3], [7], [6], the privacy risks stemming from these more recent epigenomic data attracted less attention. One of the most critical attacks in the biomedical research setting is membership inference, popularized by Homer et al.[5]: Given some raw data about a targeted individual, the attacker wants to know whether this individual is member of a dataset (i.e., has contributed his data) by solely relying on aggregated statistics about this dataset. Such a membership inference attack can have serious privacy implications if this dataset contains individuals carrying a specific disease. We aim at evaluating whether DNA methylation databases are also vulnerable to membership inference attacks. DNA methylation data is not only very sensitive as it can unveil severe diseases such as cancer, some regions of our methylation profiles are highly correlated with the genome, thus leakage of such data can indirectly expose family members’ private data. As a consequence, anticipating privacy risks and mitigating them with technical means is of utmost importance. a) Contributions: Specifically, we present multiple attacks against the membership privacy of individuals participating in DNA methylation-based studies. We consider two types of adversarial settings, both relying on mean DNA methylation statistics released about the databases. The first setting assumes the adversary to know its victim’s DNA methylation profile, while the second setting assumes the victim’s genome to be known instead. For both adversarial settings, we design three types of membership inference attacks: one based on the L1 distance, one based on the likelihood-ratio (LR) test and one based on a machine learning classifier trained on distance features. For the genome-based inference, we particularly design our attack to capture the probabilistic dependencies between the two types of biomedical data. We prove that the mean of the conditional distribution of the methylation values given the genomic values is a sufficient statistic for the genome-based attack. We then conduct an extensive evaluation of our attacks on six diverse datasets, containing a total of 1,320 patients. Our results consistently demonstrate the success of this type of attack over different tissues and diseases. While the statistical test based on the LR test exceeds 0.7 AUC and reaches over 0.95 AUC in one case (see Figure 1a), machine-learning increases the AUC to over 0.9 in most cases. Additionally, the attacker’s training data can be distinct from the target data, as our experiments on transferability demonstrate in Figure 1b. Even if the attacker only knows the target’s genome, inference of the methylation values followed by a membership inference attack is possible, as Figure 1c shows. Propelled by these results, we propose a differentially private mechanism. We empirically evaluate its effectiveness on our various datasets. While our mechanism is able to provide strong privacy guarantees, it also negatively affects the utility of the data. If the adversary gets access to the full set of methylation points, we cannot obtain perfect privacy and, at the same time, accurate statistics. However, if only a few hundred methylation values are released, the average amount of noise added decreases and reasonable privacy levels are reached, see Figure 2. GBM PA IBD CD IBD UC BC disease 0.5 0.6 0.7 0.8 0.9 1.0 a v e ra g e A U C AUC L1 test AUC LLR test (a) AUC of the L1 and LLR tests applied to all methylation positions. G B M , PA
更多查看译文
关键词
epigenetics,membership inference
AI 理解论文
溯源树
样例
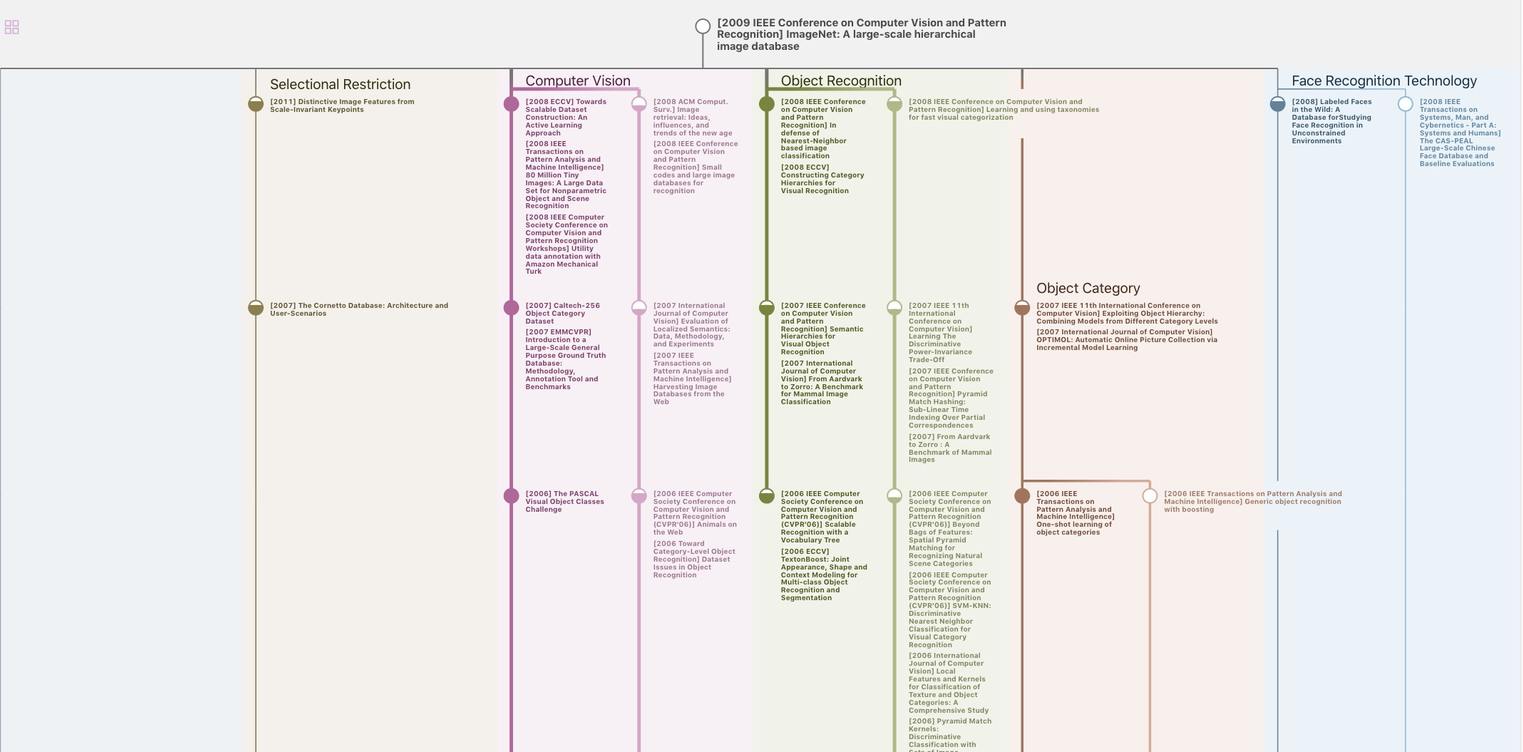
生成溯源树,研究论文发展脉络
Chat Paper
正在生成论文摘要