Stochastic inversion under functional uncertainties
semanticscholar(2018)
摘要
In this present work we propose a new efficient method for solving an inversion problem under functional uncertainties. Without loss of generality, the considered numerical simulator takes inputs that can be divided into two sets, the deterministic control variables and the functional random variables. Let f : X×V → R denote the output of the simulator where X ⊂ R is the search space of the control variables and the randomness of the simulator is derived from a random input defined in a functional space V. We assume that the probability distribution of the functional input is only known through a finite set of realizations Ξ = {v1, . . . ,vn} and each evaluation of f involves a time consuming computation. Let Xn = {x, . . . ,x} and Vn = {v, . . . ,v} denote the initial design points. The simulator responses at these design points are denoted by Z = {f(x,v), . . . , f(x,v)}. The objective of this study is to estimate the set Γ∗ = {x ∈ X , g(x) = EV[f(x,V)] ≤ c}, (1) where c ∈ R and EV is the expectation with respect to V. The estimation of Γ∗ based on computing function g at each grid point of discretized version of X requires far too many expensive simulations of f . Therefore, statistical methods based on a reduced number of evaluation points are widely used to overcome this latter difficulty by focusing the evaluations on the promising subregion of the control space. Among statistical models, Gaussian Process (GP) model has received increasing interest in recent years, due to many of its good properties, such as the existence of explicit formula of statistical moments and the easy computation of the uncertainties on predictions. However, in the literature the input variables involved in Gaussian Process models are often univariate or multivariate. The purpose of this talk is first to extend the use of Gaussian Process model to cases where the inputs contain infinite dimensional variables or functional data which are collected as curves. Then we define an infill sampling criterion based on the GP model in order to solve the stochastic inversion problem (1). After a dimension reduction of the functional space (V ∈ V −→ Karhunen-Loève U ∈ RKL), a GP model Z(x,u) is built in the joint space of control and uncertain variables (x,u). Then an averaged GP over the random variables is derived [2], Y(x) = EU[Z(x,u)]. Therefore the ’induced’ Gaussian process approximates the expected response involved in inversion problem (1). Driven by the Gaussian process conditioned on the n observations Y n (x), the proposed infill strategy consists of two steps: first we choose x by minimizing the Vorob’ev deviation [1]. Secondly we choose the uncertain point u that minimizes the variance of the process Y n (x) evaluated at the point x . By iterating this procedure, we expect a rapid estimation of the set Γ∗ with a limited number of calls to the simulator f . MascotNum Annual Conference, March 18-2
更多查看译文
AI 理解论文
溯源树
样例
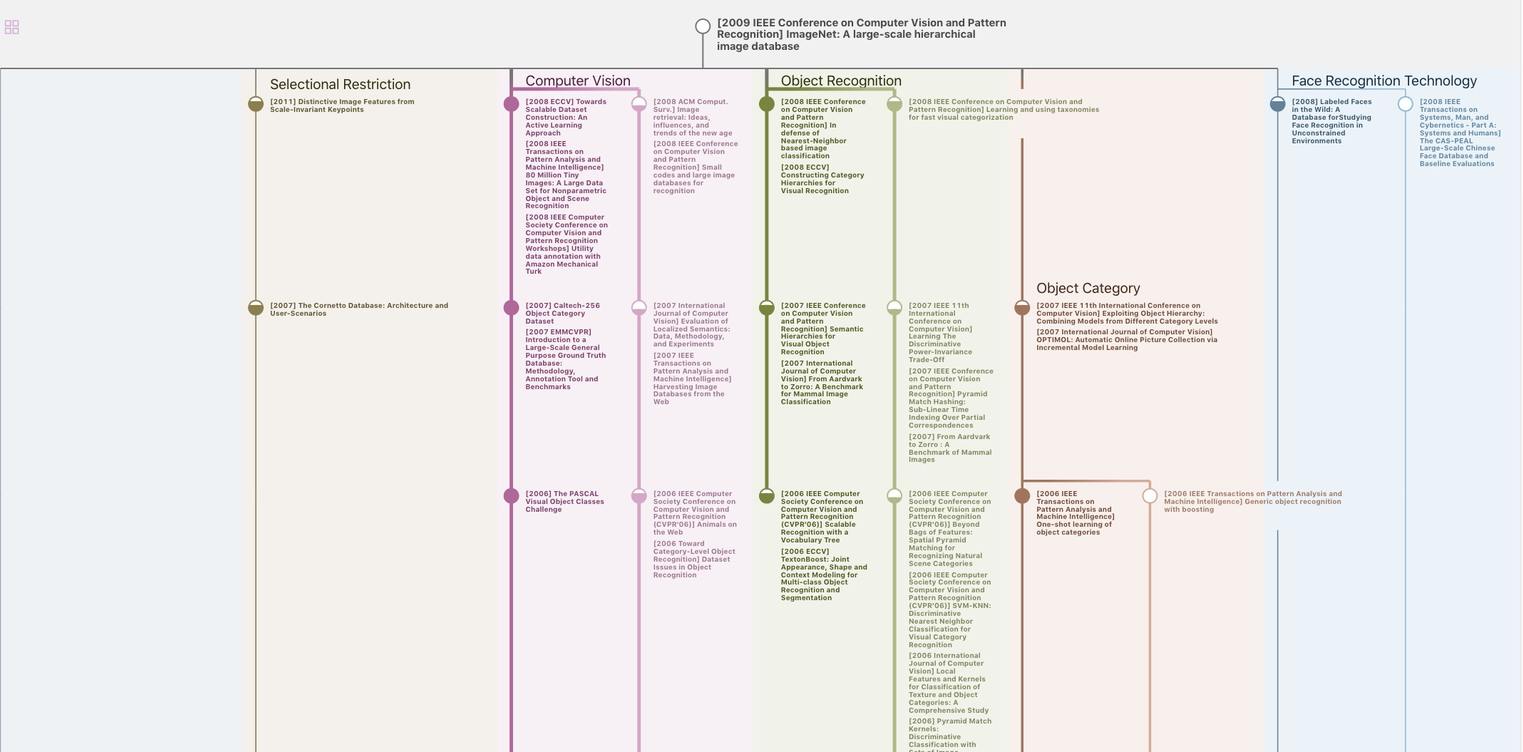
生成溯源树,研究论文发展脉络
Chat Paper
正在生成论文摘要