A Machine Learning Approach for Solving AC Optimal Power Flows
semanticscholar(2020)
摘要
The power grid is currently optimized using a MATLAB program that employs traditional methods for solving optimization problems with nonlinear constraints. These traditional methods are slow, require very close initial guesses, and cannot handle extreme deviations from the norm. We present two types of machine learning models for the IEEE’s predefined 30-bus power flow system in this paper. The first is a neural network algorithm with three hidden layers, 100 nodes in each layer, trained on over 20,000 samples with an accuracy rating of 99% that predicts valid generator configurations 80% of the time. The other model is a decision tree regression algorithm (XGBoost) that achieved an 88% fit to the data with 1000 decision trees and a depth of three levels per tree. Future work will include generalizing the models to higher nodal systems, investigating higher estimators for the regression tree model, employing optimality classifications for both models, and analysis of extreme deviations from the norm within the systems. 1Department of Mathematics, University of Houston 2Department of Mathematics, University of Kentucky 3Center for Computational Science, Tulane University 4Department of Mathematics, Kansas State University 5School of Mathematical Sciences, Rochester Institute of Technology 6Department of Civil, Arch. and Env. Engineering, University of Texas at Austin 7Operations Research Program, North Carolina State University 8Applied Statistics and Computational Modeling Group, Pacific Northwest National Lab 9Department of Mathematics, North Carolina State University
更多查看译文
AI 理解论文
溯源树
样例
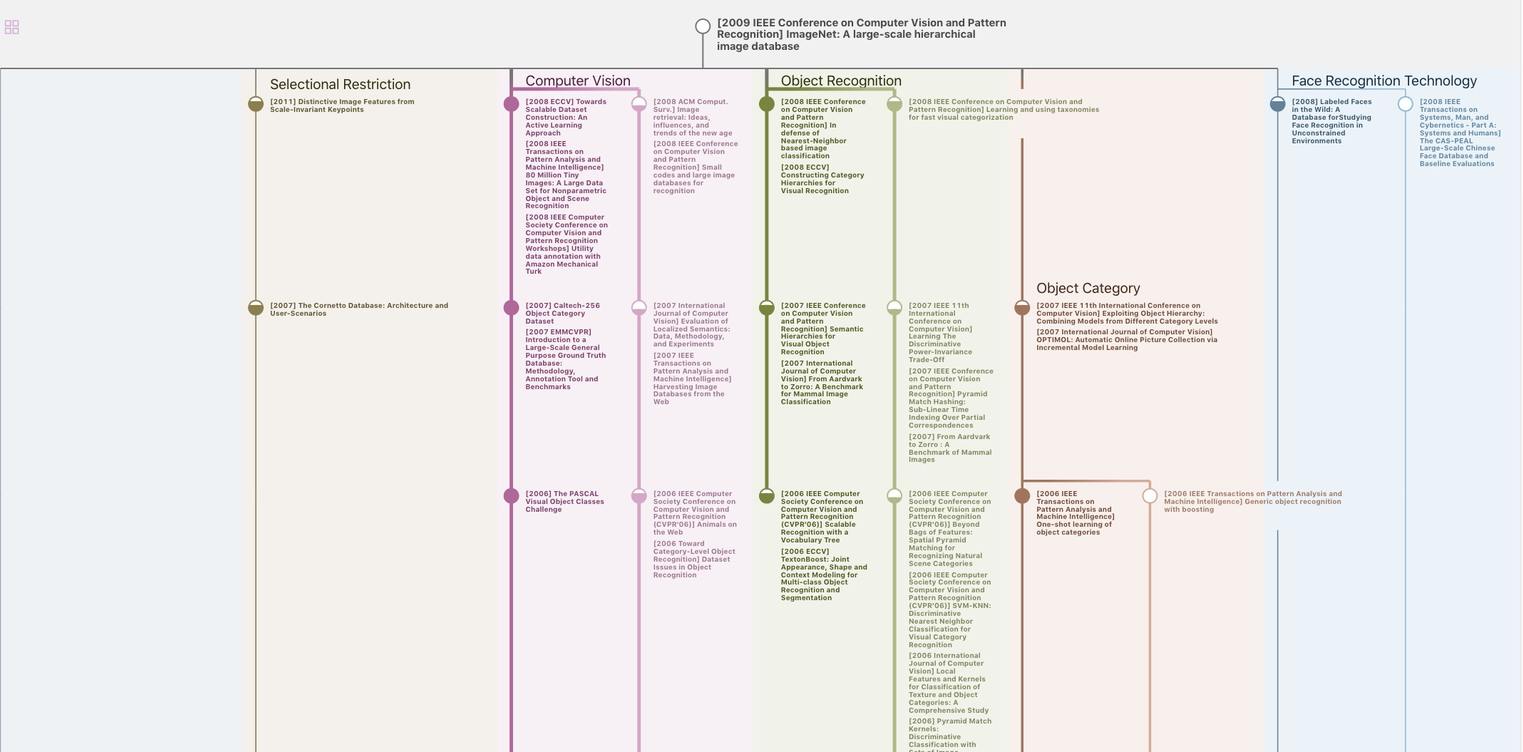
生成溯源树,研究论文发展脉络
Chat Paper
正在生成论文摘要