From Patches to Natural Images via Hierarchical Dirichlet Processes
semanticscholar(2016)
摘要
We propose a hierarchical generative model that captures the self-similar structure of image regions as well as how this structure is shared across image collections. Our model is based on a novel, variational interpretation of the popular expected patch log-likelihood (EPLL) method as a model for randomly positioned grids of image patches. While previous EPLL methods modeled the density of image patches with finite Gaussian mixtures, we use nonparametric Dirichlet process (DP) mixtures to create models whose complexity grows as additional images are observed. An extension based on the hierarchical DP then captures the repetitive and self-similar structure of image regions via image-specific variations in cluster frequencies. We derive a structured variational inference algorithm that uses birth and delete moves to create new patch clusters and thus more accurately model novel image textures. Our denoising performance on standard benchmarks is superior to EPLL and comparable to the state-of-the-art, while providing a novel statistical interpretation for many common image processing heuristics.
更多查看译文
AI 理解论文
溯源树
样例
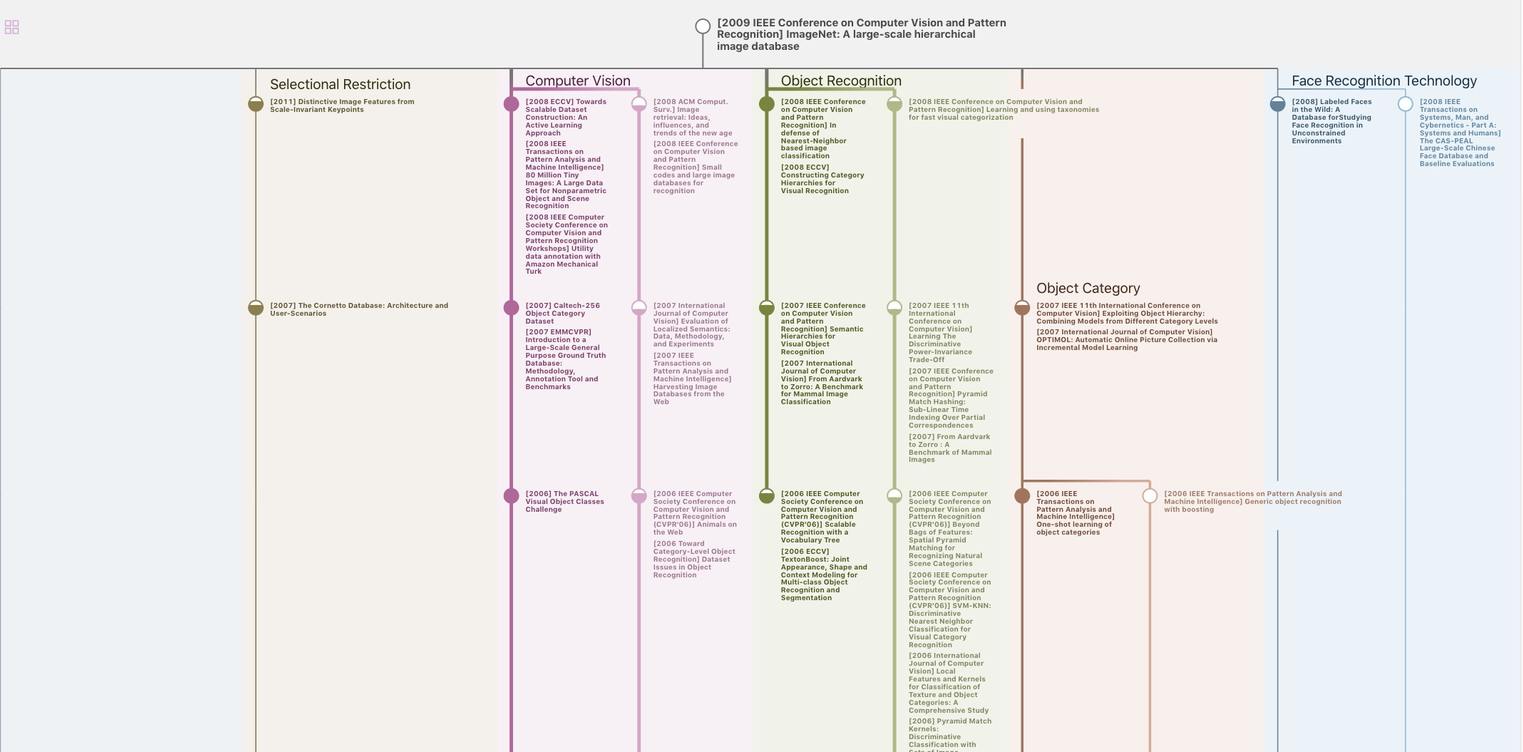
生成溯源树,研究论文发展脉络
Chat Paper
正在生成论文摘要