Simulation Study 2 . 1 Simulation Setup and Experimental Settings
semanticscholar(2017)
摘要
A good imputation model leverages relationships in the complete data to make predictions for missing values. However, there is some disagreement about how to handle imputed values in analyses when the imputation is driven by a single strong predictor, and that predictor will be included in the analysis model. One common situation is when subjects are assessed at two time points (y1 and y2), but some subjects are missing scores at one or both time points. Other auxiliary data are available for all subjects. The y2 score for each subject is typically the strongest predictor of the y1 score in the imputation model, but there is concern about “circularity” if the planned analyses then use the y1 score to predict the y2 score. Suggested approaches in the literature include a multiple imputation then deletion (MID) approach, where all missing values are imputed but observations with imputed outcomes are dropped from analyses; or using all observations (AO), including those with imputed outcomes, for analyses following imputation. This paper investigates the conditions under which circularity may be a concern, studies the performance of the MID and AO methods under different settings, and makes recommendations for practice.
更多查看译文
AI 理解论文
溯源树
样例
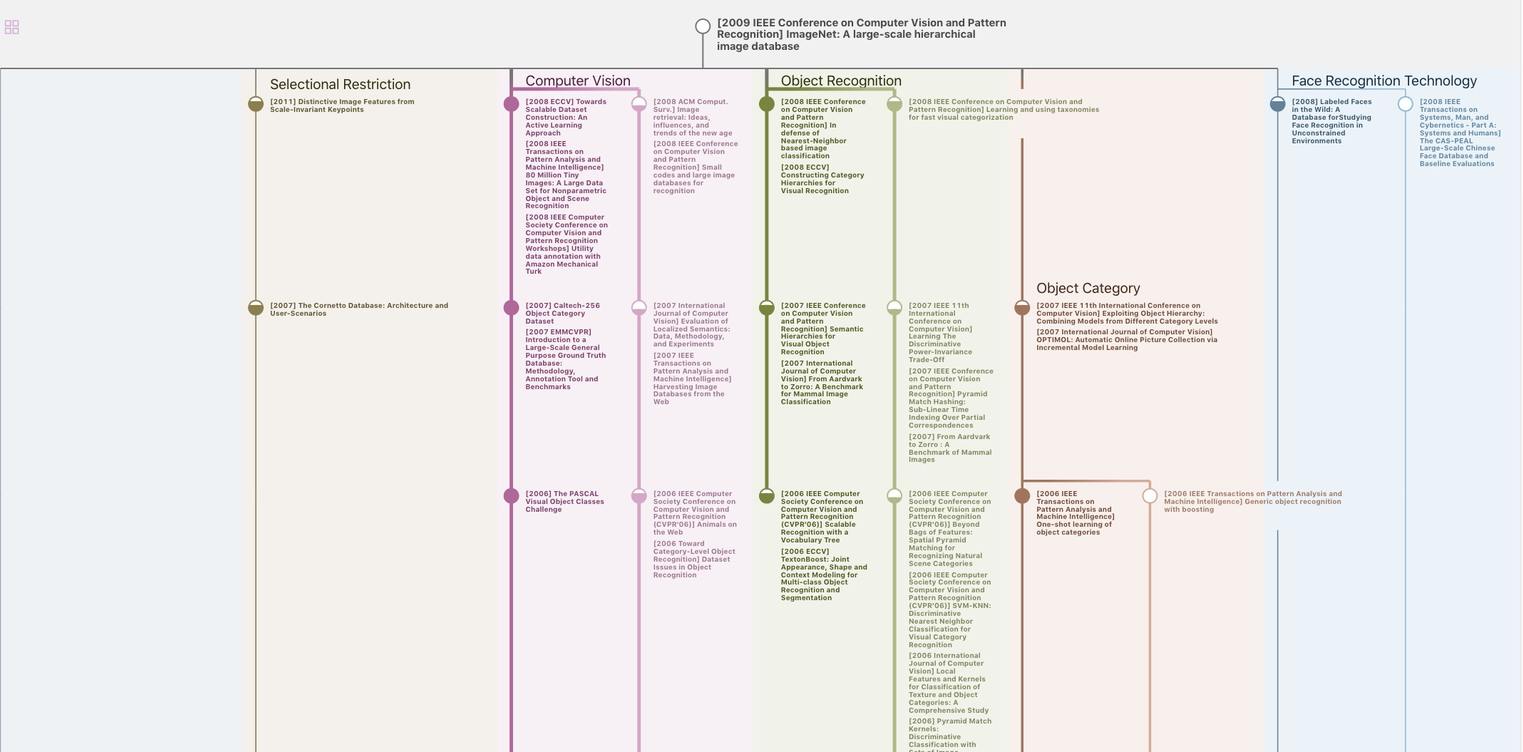
生成溯源树,研究论文发展脉络
Chat Paper
正在生成论文摘要