Identifying paths-to-purchase segments via Clustered Vector Autoregression
semanticscholar(2014)
摘要
Vector Autoregression Models (VAR) are widely used by researchers to capture the linear interdependencies among multiple time series. We propose a novel method called Clustered VAR (CVAR) to identify components of the data generated by a mixture of K VAR processes. By applying a CVAR model to a consumer-level time series dataset on shopping behavior at a retailer, we segment consumers based on their path-to-purchase. We estimate the CVAR model using the EM (expectation–maximization) algorithm that assigns each consumer into a segment that maximizes the likelihood and optimizes the VAR parameters for each segment given the membership assignments. We verify the effectiveness of the Clustered VAR model on a simulated dataset. Following successful evaluation, we apply the Clustered VAR model to a retail dataset from a major multi-channel, multi-brand North American Retailer. Our study could segment 2,000 randomly selected consumers into 4 clusters and offers insights on two issues: 1. Potential interdependencies among online marketing, offline marketing and their effects for each group, 2. Differences in the above effects across consumer segments. As a result, the consumer clusters in our study will guide managers in tailoring the marketing mix for different customer segments to help them move forward on the path-to-purchase.
更多查看译文
AI 理解论文
溯源树
样例
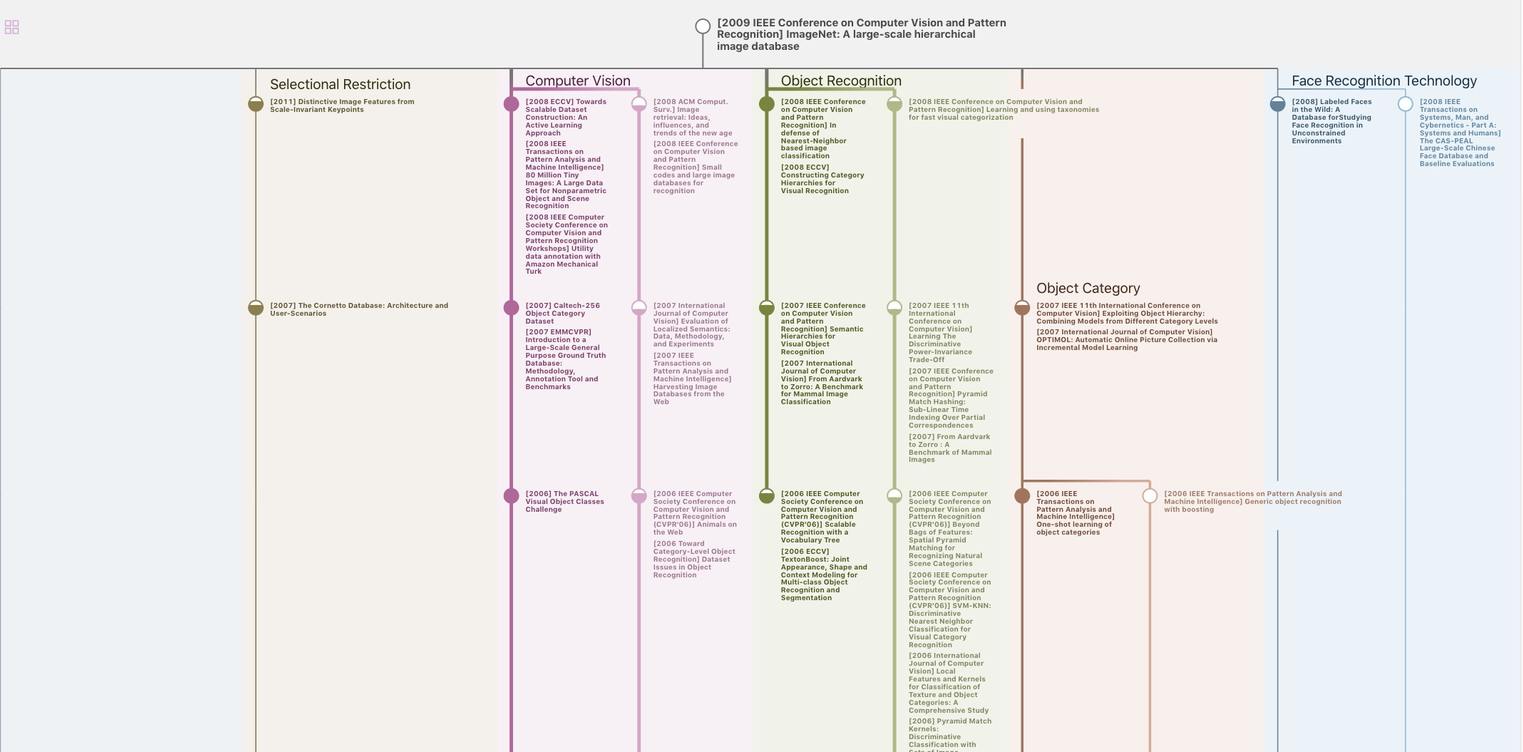
生成溯源树,研究论文发展脉络
Chat Paper
正在生成论文摘要