Towards Local Learning and MCMC Inference in Biologically Plausible Deep Generative Networks
semanticscholar(2018)
摘要
We propose an ansatz for approximate Markov Chain Monte Carlo (MCMC) inference and learning in multi-layered (deep) generative models that exhibit biologically plausible dynamics. In particular, our approach builds on a recently observed connection between the classical integrate-and-fire models of biological neurons and Bayesian inference based on Langevin dynamics. Utilizing this connection, we propose that a recurrent neural network model can be derived that learns to perform inference for a feed-forward generative model from local learning signals only. When combined with such a generative model – which can also be trained from information locally available at each neuron – our model provides new perspectives on how biologically plausible learning algorithms could be reconciled with statistical learning theory. We experimentally validate our model using synthetic images as well as the MNIST dataset and show that it approaches the level of performance of standard machine learning algorithms for training generative models on these simple tasks.
更多查看译文
AI 理解论文
溯源树
样例
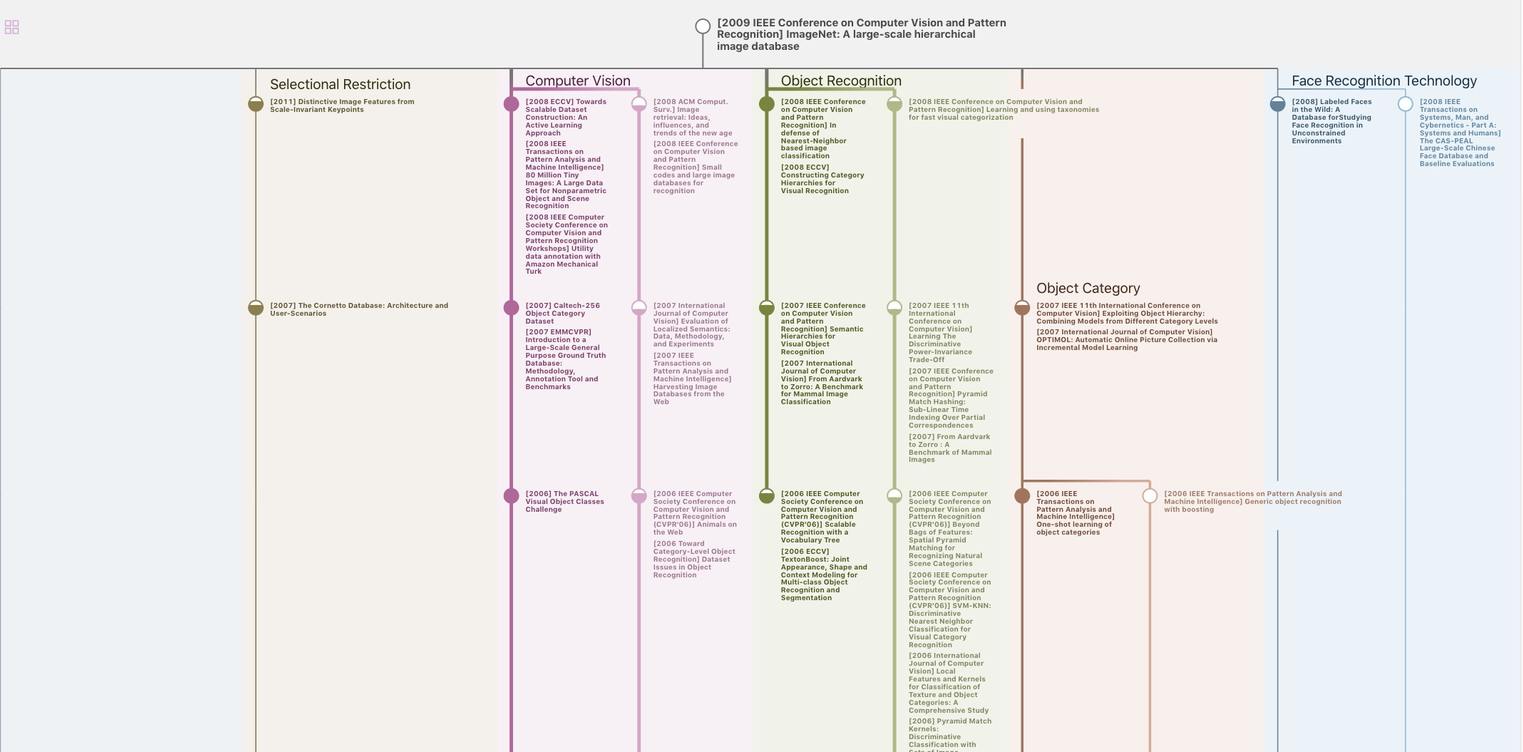
生成溯源树,研究论文发展脉络
Chat Paper
正在生成论文摘要