Robust Sparse Walsh-Hadamard Transform : The SPRIGHT Algorithm
semanticscholar(2015)
摘要
We consider the problem of stably computing the K-sparse N -point Walsh-Hadamard Transform (WHT) of a noisy input vector of length N , where K = O(N δ) scales sub-linearly in the signal dimension N for some δ ∈ (0, 1). The most efficient way known by far for computing the WHT of an arbitraryN -length signal is the Fast Walsh-Hadamard Transform (FWHT), which usesN samples and O(N logN) operations. However, given that the signal is K-sparse, can we perform the same task with much fewer samples and computations, even in the presence of noise? This paper answers the question affirmatively by presenting our SParse Robust Iterative Graphbased Hadamard Transform (SPRIGHT) algorithm, which computes the K-sparse N -point WHT in the presence of additive Gaussian noise, using a near-order-optimal number of samples O(K logN) and sub-linear computational complexity O(K logN). The SPRIGHT algorithm also admits the option of spending near-linear run-timeO(N logN) with an order-optimal sample costO(K logN), which maintains the same sample cost obtained in [1] for the noiseless scenario. ∗This work was supported by grants NSF CCF EAGER 1439725, and NSF CCF 1116404 and MURI CHASE Grant No. 556016.
更多查看译文
AI 理解论文
溯源树
样例
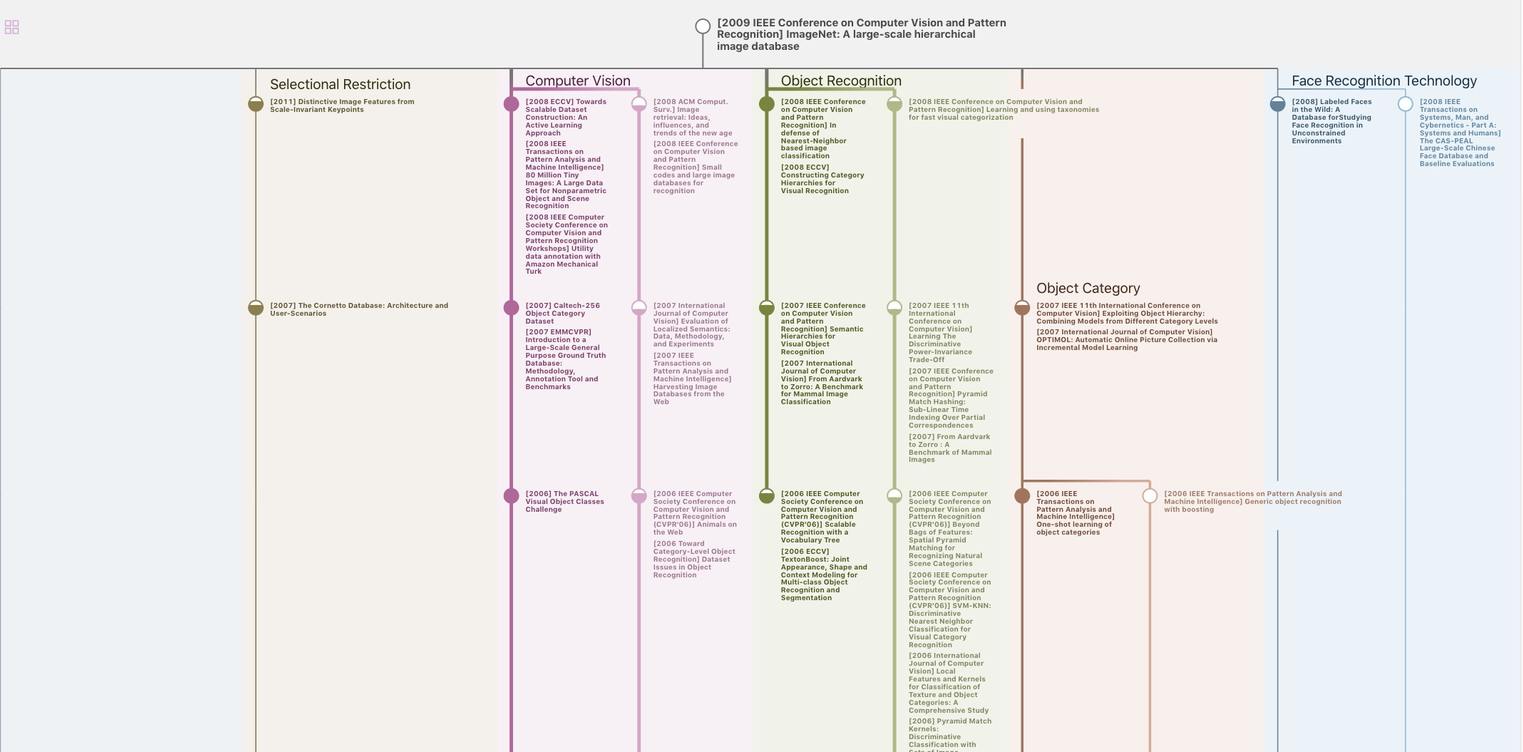
生成溯源树,研究论文发展脉络
Chat Paper
正在生成论文摘要