Layer-wise Performance Bottleneck Analysis of Deep Neural Networks
semanticscholar(2017)
摘要
Deep neural networks (DNNs) are becoming the inevitable part of a wide range of applications domains, such as visual and speech recognition. Recently, Graphics Processing Units (GPUs) are showing great success in helping meet the performance and energy efficiency demands of DNNs. In this paper, we identify GPU performance bottlenecks via characterizing the data access behaviors of AlexNet and VGG16 models in a layer-wise manner. The goal of this work is to find the performance bottlenecks of DNNs. We obtain following findings: (i) Backward propagation is more performance critical than forward propagation. (ii) The working set of convolutional interlayers does not fit in L1 cache, while convolutional input layer can exploit L1 cache sufficiently. (iii) Interconnect network can also be a performance bottleneck that substantially increase GPU memory bandwidth demand.
更多查看译文
AI 理解论文
溯源树
样例
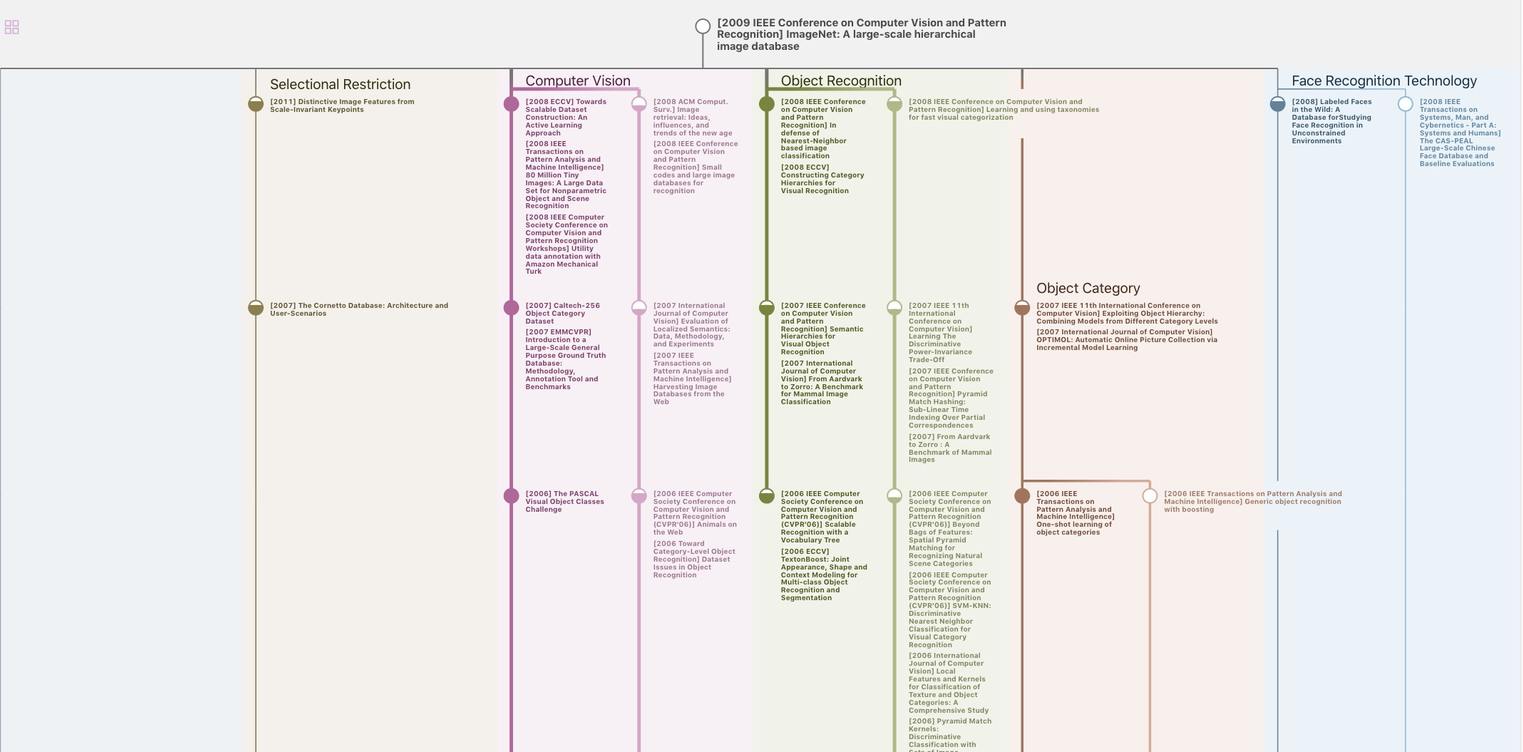
生成溯源树,研究论文发展脉络
Chat Paper
正在生成论文摘要