Touring Dataland ? Automated Recommendations for the Big Data Traveler
semanticscholar(2016)
摘要
In this paper, we develop features and models to predict three important aspects of big data transfers: location, throughput, and errors. We develop and evaluate our models using more than 4 million historical transfers conducted by Globus. Our work is the rst to study user-managed transfers in wide area networks comprised of user-owned endpoints, as opposed to previous work which focuses on experimental workloads, dedicated networks, and powerful computers. is real-world data presents several new challenges, including sparsity and lack of historical data, which we overcome by applying powerful ensemble machine learning algorithms and recurrent neural networks to summarize previous transfer information. Our approaches can predict the storage locations used with 78.2% and 95.5% accuracy for top-1 and top-3 recommendations, respectively. We model the throughput of transfers to within a factor of nearly two, and le transfer failures within approximately 1%. ACM Reference format: William Agnew, Kyle Chard (advisor), and Ian Foster (advisor). 2016. Tour-
更多查看译文
AI 理解论文
溯源树
样例
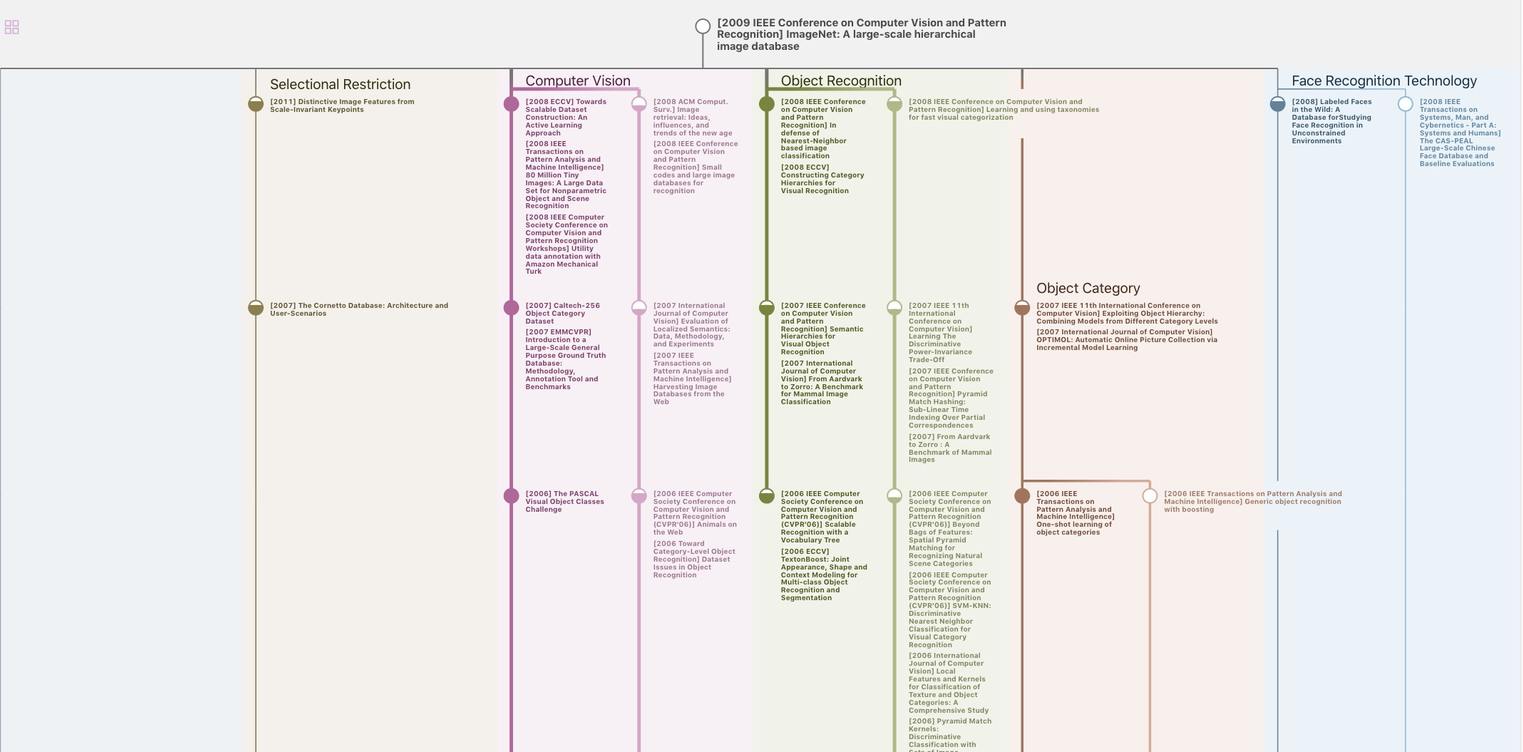
生成溯源树,研究论文发展脉络
Chat Paper
正在生成论文摘要