Privacy Aware Feature Selection : An Application to Protecting Motion Data
semanticscholar(2015)
摘要
Advances in machine learning provide the ability to predict personal data from seemingly unrelated sources. We focus on privacy leaks from providing motion data from a smartphone and seek to understand the risk to personal privacy. We collect a data set containing 74 statistical features from various motion sensors continuously collected from 88 subjects providing over 40 hours of data. An ideal privacy mechanism would allow the user to release motion data not capable of predicting sensitive private information while still being useful for other applications. We take the first steps toward such a mechanism by understanding which statistical features in the motion data predict private information using an expensive brute-force search. We evaluate the ability of inexpensive feature selection algorithms to choose the set of private features and demonstrate the variability of feature selection among fifteen feature selection methods. Some of this variation is caused by correlated features which can be clustered to decrease the search space of the brute-force search. We find that traditional feature selection gives different top features and is suboptimal at selecting the most privacy sensitive features. Our work motivates the need for further study to develop private feature selection that is scalable to large
更多查看译文
AI 理解论文
溯源树
样例
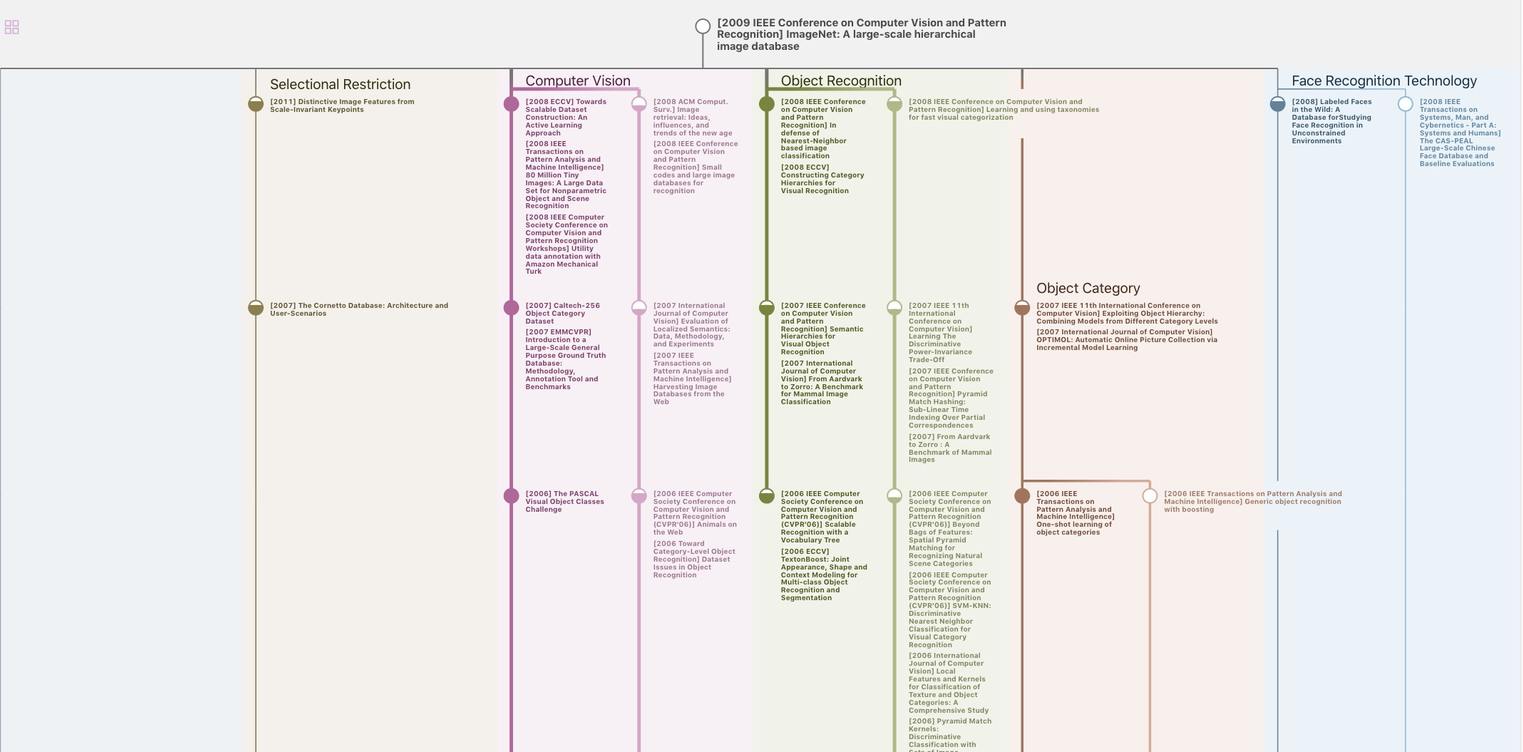
生成溯源树,研究论文发展脉络
Chat Paper
正在生成论文摘要