Supplementary material : Curriculum Learning of Multiple Tasks
semanticscholar(2016)
摘要
Assume that the learner observes a sequence of tasks in a fixed order, t1, ..., tn, with corresponding training sets, S1, ..., Sn, where Si = {(x1, y 1), ..., (ximi , y i mi)} consists of mi i.i.d. samples from a task-specific data distribution Di. We assume that all tasks share the same input space X and output space Y and that the learner uses the same loss function l : Y × Y → [0, 1] and hypothesis set H ⊂ {h : X → Y} for solving these tasks. The learner solves only one task at a time by using some arbitrary but fixed deterministic algorithm A that produces a posterior distribution Qi over H based on training data Si and some prior knowledge Pi, which is also expressed in form of probability distribution over the hypothesis set. Moreover, we assume that the solution Qi plays the role of a prior for the next task, i.e. Pi+1 = Qi (P1 is just some fixed distribution, Q0). For making predictions for task ti the learner uses the Gibbs predictor, associated with the corresponding posterior distribution Qi. For an input x ∈ X this randomized predictor samples h ∈ H according to Qi and returns h(x). The goal of the learner is to perform well on all tasks, t1, ..., tn, i.e. to minimize the average expected error of the Gibbs classifiers defined by Q1, . . . , Qn:
更多查看译文
AI 理解论文
溯源树
样例
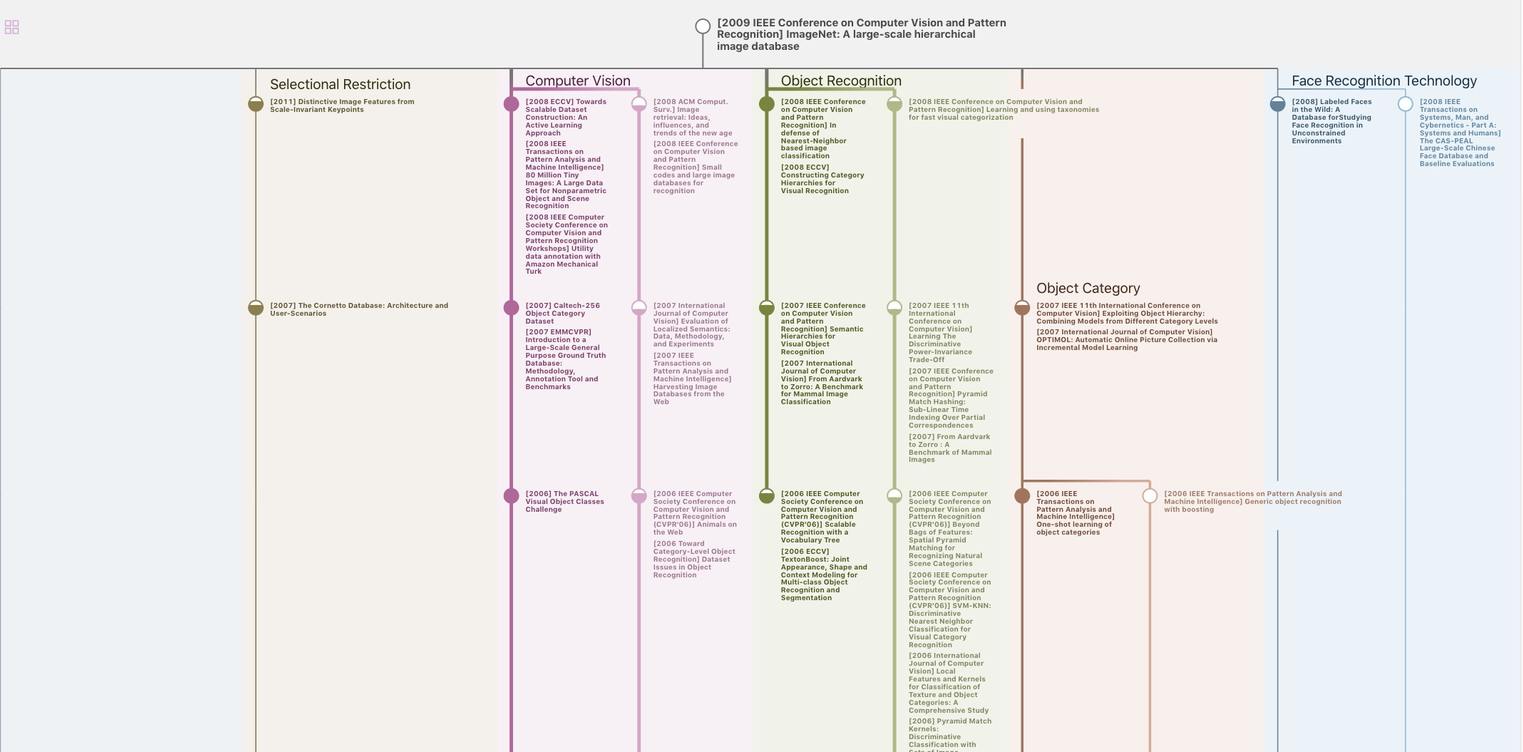
生成溯源树,研究论文发展脉络
Chat Paper
正在生成论文摘要