An integrated unsupervised change detection in multispectral images using svm and neural networks
semanticscholar(2013)
摘要
Importance: Satellite Remote sensed data and GIS for land cover, land use and its changes is a key to many diverse applications such as Environment, Forestry, Hydrology, Agriculture and Geology. Natural Resource Management, Planning and Monitoring programs depend on accurate information about the land cover and its changes along the time in a region. Methods for monitoring changes varies from intense sampling from field with plot inventories to vast analysis of remotely sensed data which has proven to be more cost effective for large areas, small site assessment and analysis. A number of change detection methods have been proposed in the past, among which are efficient based on model techniques such as the Generalized Likelihood Ratio (GLR) stochastic test. Introducing of the problem along with data used: Here we regard the case where not accurate nor can tractable model be found, by a model-free method, namely as the Kernel change detection (KCD). The reliability of support vector machines for classifying multispectral data in the remote sensing has been proven in various researches. Depending on the soft margin single-class Support Vector Machine (SVM); a un-similarity measure in feature space between those sets, without estimating densities as an intermediary step is imported. Method used: A method based on SVM and artificial neural network (ANN) was developed to detect newly countryside urbanized areas illustrated in satellite sensor data. This method uses a pair of Landsat Thematic Mapper (TM) images taken from a region and acquired on different dates as input and supervises the SVM system to classify the image data into 'from to to' classes and also implies a parameter adjustment using the ANN method. Furthermore, The Levenburg-Marquardt algorithm was used to accelerate the ANN's convergence. The high-dimensional feature vectors of multi spectral data often impose a high computational cost as well as the risk of “over fitting” when classification is performed. Therefore it is necessary to reduce the dimensionality through ways like feature selection. In this paper, we present a classification of multi spectral data using Support Vector Machine (SVM), a state-of-art classifier that has found success in a variety of areas along with the neural network (NN), which seeks to solve optimization problems using the methods of neuron connection, specifically implying hidden layers which was used to optimize both the feature subset, i.e. band subset, of multi spectral data and SVM kernel parameters simultaneously. Analysis and conclusion: An empirical result from a case study shows that the ANN-based method requires not so much training time but can be somehow 2030% much more accurate than post-classification comparisons. The outputs imply that the practical value of ANN-based change detection is efficient and optimized. Comparison of the optimized results and the non-optimized results implies that the Neuro-SVM approach could significantly reduce the computation time and cost while raising the classification accuracy. The number of bands used for classification was reduced, while the classification and detection accuracy increased considerably. In addition and conclusion, the proposed Neuro-SVM can optimize feature subsets change detection and SVM kernel parameters at the same time, therefore can be applied in feature selection of the hyper spectral data.
更多查看译文
AI 理解论文
溯源树
样例
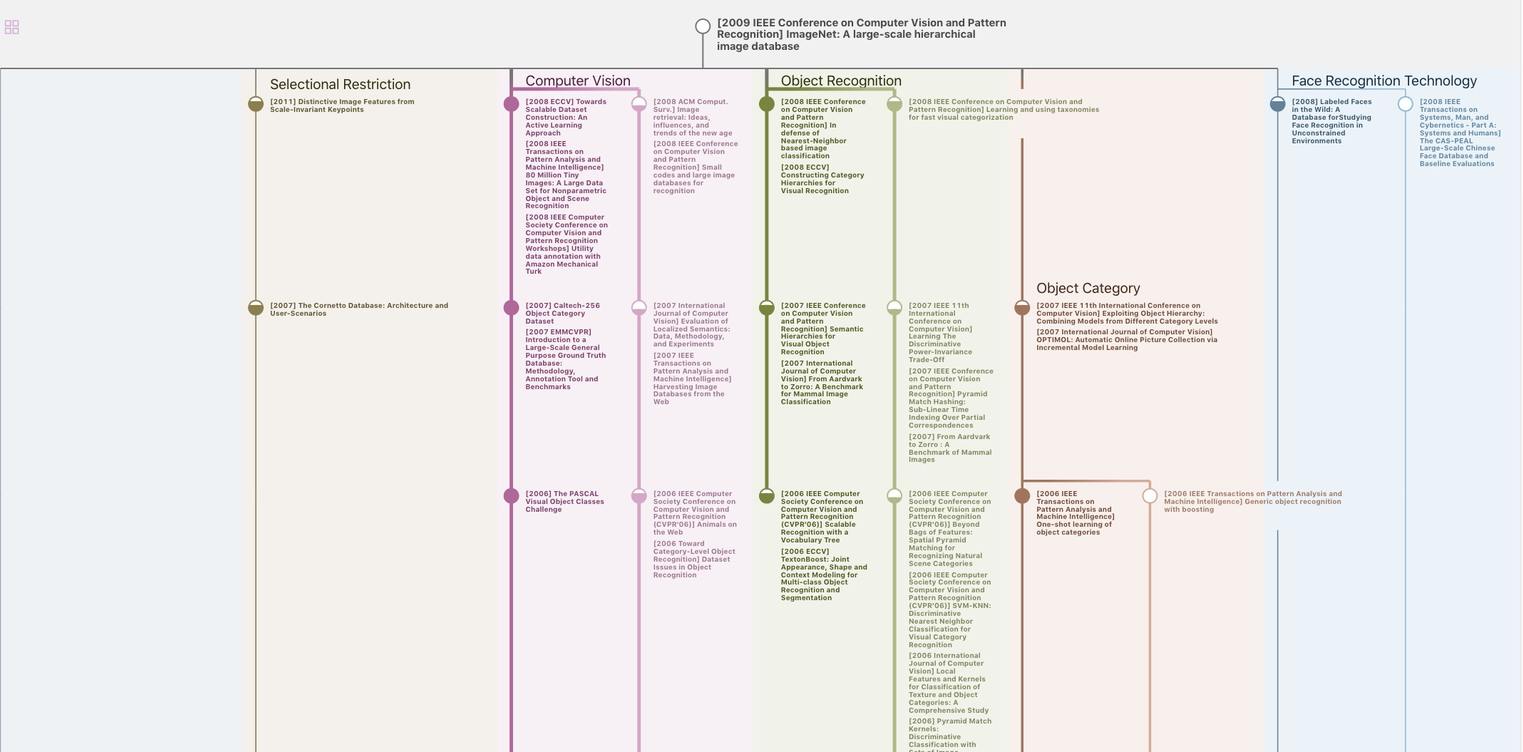
生成溯源树,研究论文发展脉络
Chat Paper
正在生成论文摘要