MATERIALS 3 , 093802 ( 2019 ) Atomic energy mapping of neural network potential
semanticscholar(2019)
摘要
We investigate the atomic energy mapping inferred by machine-learning potentials, in particular neural network potentials. We first show that the transferable atomic energy can be defined within the density functional theory, which means that the core of machine-learning potentials is to deduce a reference atomic-energy function from the given set of total energies. By utilizing invariant points in the feature space at which the atomic energy has a fixed reference value, we examine the atomic energy mapping of neural network potentials. Examples on Si consistently support that NNPs are capable of learning correct atomic energies. However, we also find that the neural network potential is vulnerable to ‘ad hoc’ mapping in which the total energy appears to be trained accurately while the atomic energy mapping is incorrect in spite of its capability. We show that the energy mapping can be improved by choosing the training set carefully and monitoring the atomic energy at the invariant points during the training procedure. The energy mapping in multicomponent systems is also discussed.
更多查看译文
AI 理解论文
溯源树
样例
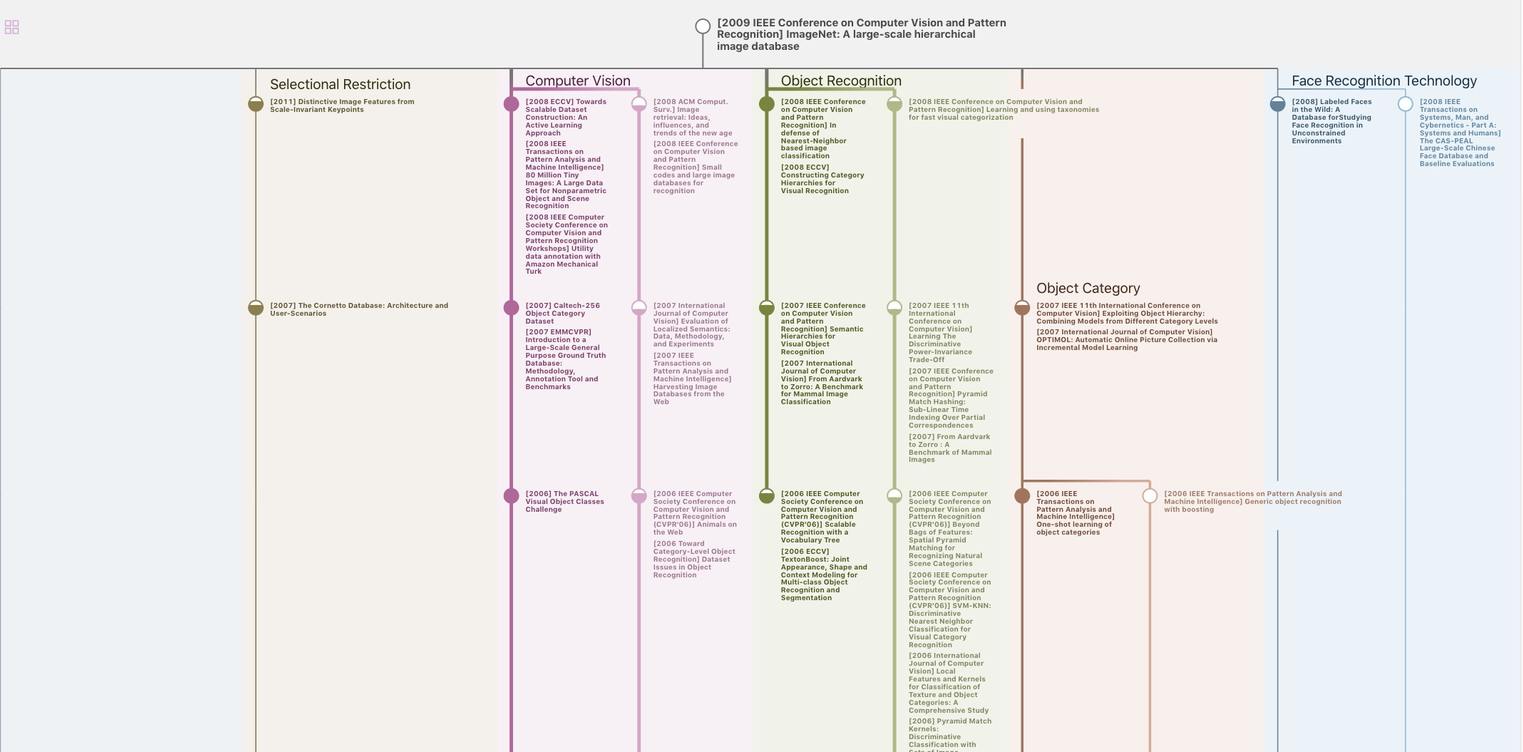
生成溯源树,研究论文发展脉络
Chat Paper
正在生成论文摘要