Privacy-Aware Malware Detection
semanticscholar(2016)
摘要
Overview Cloud service providers (e.g., Amazon Web Service, IBM Cloud, Microsoft Azure) are not allowed to access the content of customer virtual machines. In consequence, it’s harder for the providers to protect their infrastructure from malware infection. As virtual machines are generally created from a limited set of standard images and likely share many vulnerabilities, malware can propagate rapidly through cloudinfrastructure and cause damage on a larger scale. Thus, this project aims to introduce a malware detection technique on cloud-infrastructure: the idea is to use sub-semantic features from a virtual machine monitor without introspection of customer virtual machines. Previous works show the effectiveness of using hardware indicators as sub-semantic features [3, 7]. At them same time, the representative virtualization framework such as Xen [1] supports reading those indicators via built-in analysis tools [6, 5]. Our research consists of three steps: we design sub-semantic features on top of the indicators from a virtual machine monitor at first, we implement our malware detection frameworks using the features, and we evaluate effectiveness of our framework by using malware in the wild and comparing the result with previous works.
更多查看译文
AI 理解论文
溯源树
样例
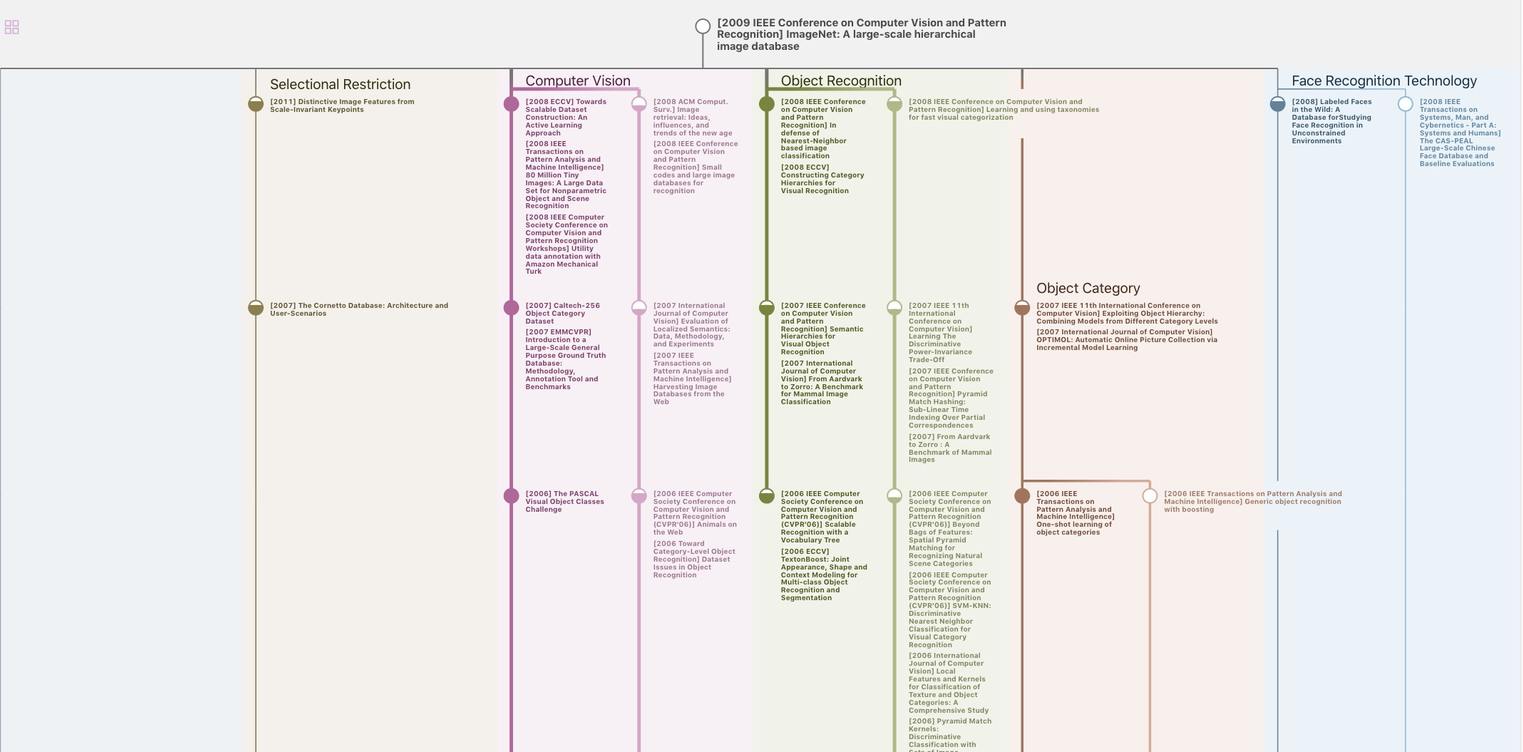
生成溯源树,研究论文发展脉络
Chat Paper
正在生成论文摘要