Methodological Challenges in Estimating Tone: Application to News Coverage of the U.S. Economy
semanticscholar(2016)
摘要
Machine learning methods have made possible the classification of large corpora of text by measures such as topic, tone, and ideology. However, even when using dictionary-based methods that require few inputs by the analyst beyond the text itself, many decisions must be made before a measure of any kind is produced from the text. When coding media the analyst must decide on the universe of media sources to sample from, as well as the criteria for selecting articles for coding from within that universe. If utilizing a supervised learning method, the method of generating training data presents many decisions: the unit of analysis to code, choice of coders, number of articles or units to code, number of coders per unit, and method of dealing with multiple codings of a single object. In this paper we consider the many decisions made by the analyst in using machine learning to classify media texts—using as a running example efforts to measure the tone (positive, negative, neutral) of newspaper coverage of the economy—and highlight our key findings throughout. In particular, we show that the decision of how to choose the corpus matters a great deal. We also introduce coder variance as a simple but novel measure of coder quality, and we demonstrate that this concept can be used to illustrate the varying returns to using multiple coders versus larger sample sizes in construction of a training dataset optimized for best classifier production. Finally, we introduce Classifer Training Using Multiple Codings, an improved method of utilizing multiple codings of individual objects, and demonstrate through simulation that it outperforms alternatives.
更多查看译文
AI 理解论文
溯源树
样例
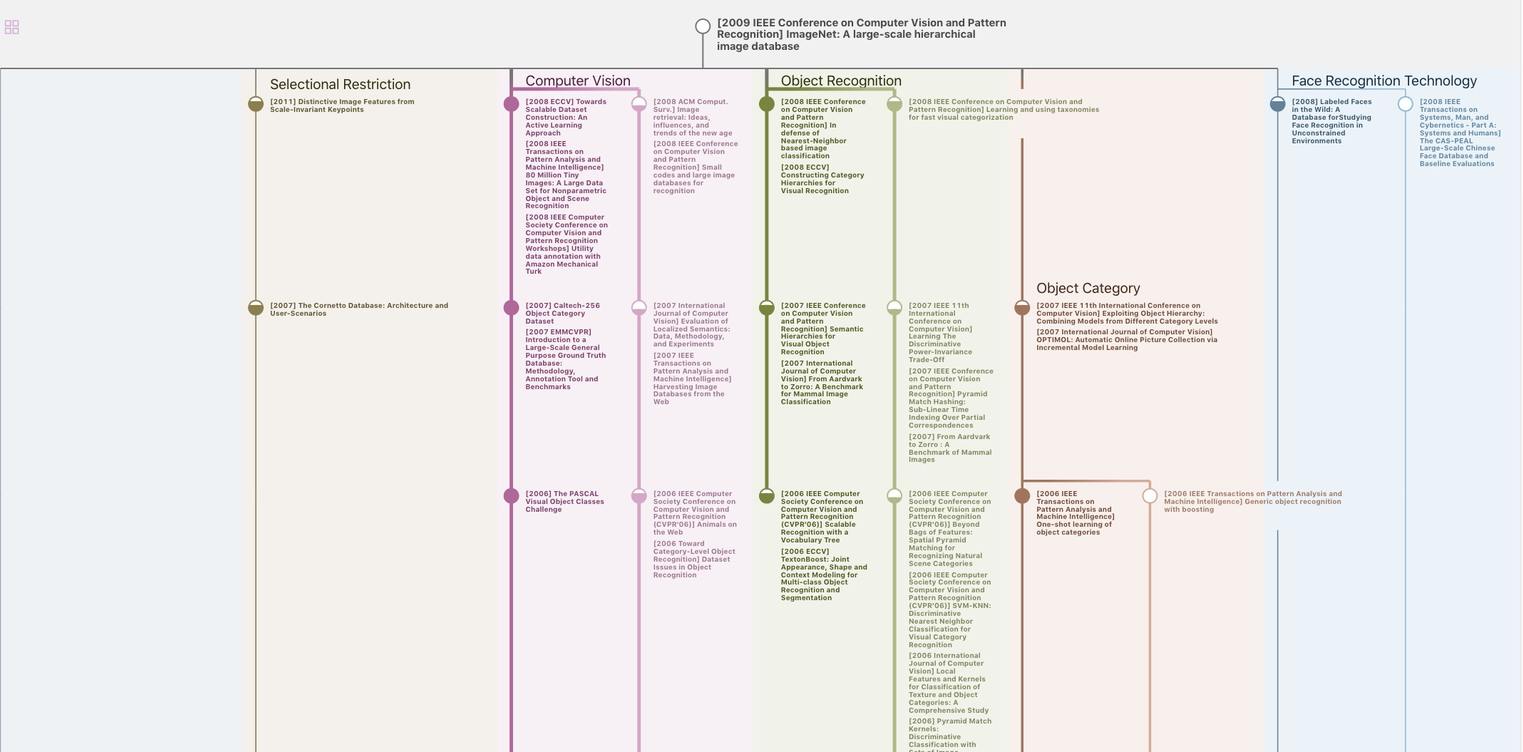
生成溯源树,研究论文发展脉络
Chat Paper
正在生成论文摘要