A mathematical approach to merging data from different 1 trace gas / particulate sensors having dissimilar ( T 90 ) 2 response times : Application to fire emission factor 3 determination 4 5
semanticscholar(2019)
摘要
13 Low cost atmospheric composition sensors are increasingly used in both air quality 14 research and in air pollution monitoring. Those focused on particulates have generally 15 rather rapid (T90) response times due to their reliance on light scattering methods. 16 However, those electrochemical and NDIR sensors targeting trace gases such as, 17 respectively, CO and CO2 typically respond very much slower, with T90 response 18 times that can be further lengthened by the rate at which sample exchange occurs in 19 the measurement cell. Data from these types of low cost sensors are often used to 20 derive emission ratios [ERs] of two simultaneously measured atmospheric species, yet 21 ER derivations made using data from sensors having dissimilar T90 values can be 22 problematic, because any rapid change in the pollutant concentration can mean one 23 sensor responding faster to the changing conditions than the other. Such situations are 24 typical within biomass burning plumes, yet where such ER assessments are routinely 25 required to generate the emissions factors (EFs) needed for biomass burning 26 emissions calculations. Here we confirm that the ERs coming from such analyses can 27 be strongly biased if differential sensor T90 effects remain unadjusted for, and we 28 demonstrate a simple mathematical approach for undertaking this adjustment. The 29 method is able to take data from a sensor taken with a particular T90 value and 30 simulate that which would be collected from a sensor targeting the same species but 31 with a different T90 value, and if the output T90 is selected to match that of the 32 companion sensor measuring the second species then the two measures with the same 33 effective T90 values can be used to generate unbiased emissions ratios and emissions 34 factors. We demonstrate our approach on simulated data with known T90 response 35 times, and then apply it to real data from low-cost sensors collected within biomass 36 burning plumes, including those installed in a sampling system that introduced further 37 – and unknown – additional measurement lag. In the latter case we find that our 38 mathematical approach to the post-measurement T90 adjustment is still able to derive 39 ERs from the low-cost sensor data that are comparable to those derived from data 40 collected with a very high precision laser absorption spectrometer that measures the 41
更多查看译文
AI 理解论文
溯源树
样例
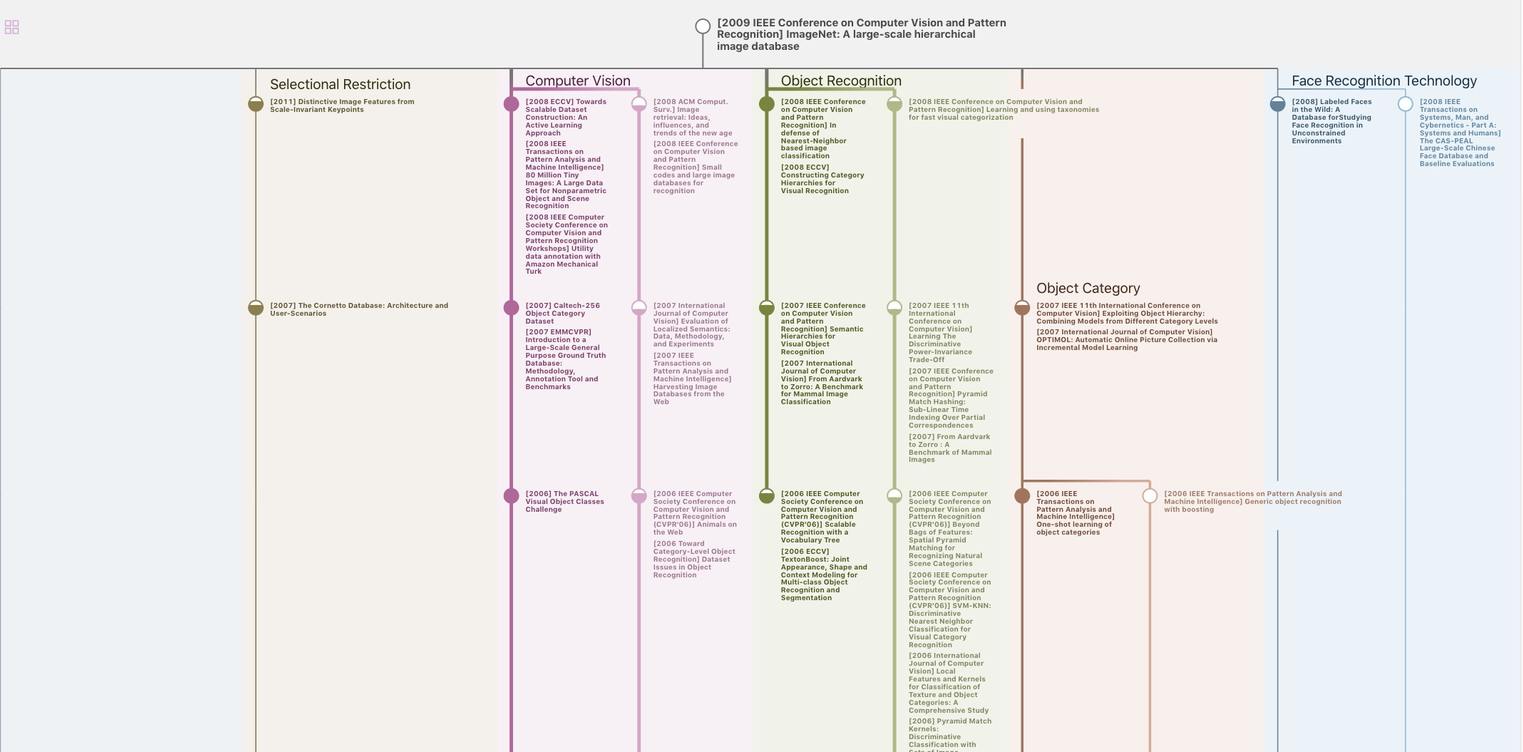
生成溯源树,研究论文发展脉络
Chat Paper
正在生成论文摘要