ReFrESH : Dynamic Decision Making in the Presence of Faults and Uncertainty in Self-Adaptive Multi-Robot Systems
semanticscholar(2014)
摘要
This paper presents an infrastructure for dynamic monitoring, reasoning and maintenance of the performance of a task solution for resource constrained multi-robot systems in the presence of faults and uncertainty. We call it ReFrESH. The goal of ReFrESH is to provide diagnosability and maintainability infrastructure support, built into a real-time operating system, to manage task performance in the presence of unexpected uncertainties. Specifically, the advantages of ReFrESH for the support of dynamic decision making are that it: (1) automatically detects and triggers self adaptation based on fault diagnosis; (2) enables the synthesis of new task solution candidates dynamically; (3) supports online estimation for each task solution candidate and selects the optimal one as the new task solution. We illustrate the feasibility of ReFrESH through a target tracking task deployed on a multi-robot system.
更多查看译文
AI 理解论文
溯源树
样例
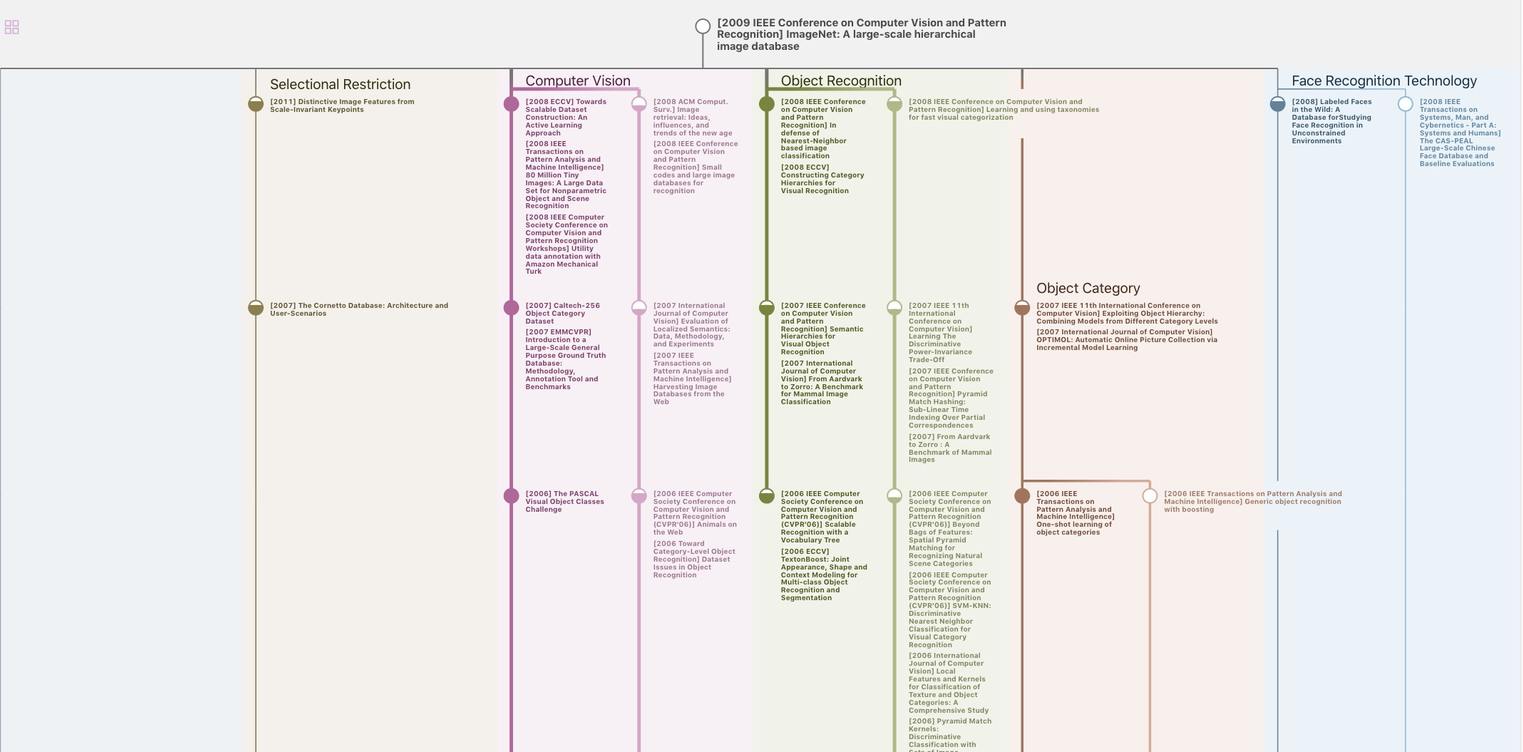
生成溯源树,研究论文发展脉络
Chat Paper
正在生成论文摘要