Data De-anonymization : From Mobility Traces to On-line Social Networks
semanticscholar(2014)
摘要
When people utilize social applications and services, their privacy suffers potential serious threat. In this paper, we present a novel, robust, and effective de-anonymization attack to mobility trace data and social data. First, we design a Unified Similarity (US) measurement which takes account of local and global structural characteristics of data, information obtained from auxiliary data, and knowledge inherited from on-going de-anonymization results. By analyzing the measurement on real data sets, we find that some data can potentially be de-anonymized accurately and the other can be de-anonymized in a coarse granularity. Utilizing this property, we present a US based De-Anonymization (DA) framework, which iteratively de-anonymizes data with accuracy guarantee. Then, to de-anonymize large scale data without the knowledge on the overlap size between the anonymized data and the auxiliary data, we generalize DA to an Adaptive De-Anonymization (ADA) framework. By smartly working on two core matching subgraphs, ADA achieves high de-anonymization accuracy and reduces computational overhead. Finally, we examine the presented de-anonymization attack on three well known mobility traces: St Andrews, Infocom06, and Smallblue, and three social data sets: ArnetMiner, Google+, and Facebook. The experimental results demonstrate that the presented de-anonymization framework is very effective and robust to noise. For instance, utilizing the knowledge of one seed mapping merely, 93.2% of the data in Smallblue can be successfully de-anonymized; utilizing the knowledge of ten seed mappings, August 13, 2014 DRAFT
更多查看译文
AI 理解论文
溯源树
样例
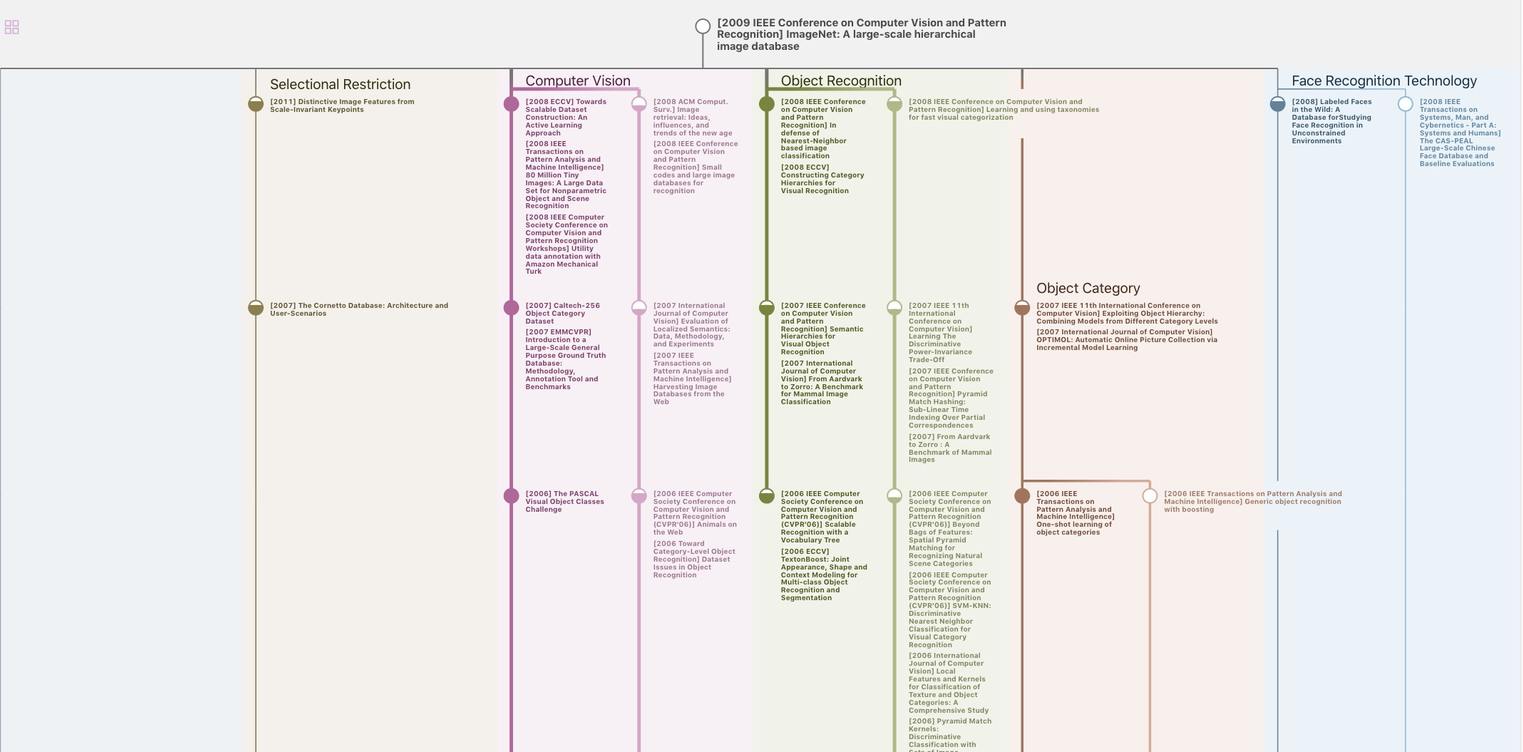
生成溯源树,研究论文发展脉络
Chat Paper
正在生成论文摘要