DEMO : XPlan – Experiment Planning for Synthetic Biology
semanticscholar(2018)
摘要
In the field of synthetic biology, as with other emerging fields of engineering, scientific exploration and engineering design are intimately entwined. Unlike established fields of engineering, synthetic biology has only highly uncertain and incomplete mechanistic models. As a result, engineering synthetic biological systems is an incremental process in which the production of designs is closely interleaved with execution of experiments to assess the success of those designs and data analysis to identify factors and mechanisms responsible for design successes and failures. In this demonstration, we show how a Hierarchical Task Network (HTN) planning system automates scientific discovery, closing the loop betweenmultiple machine learning analysis and biological design tools and wet labs to guide the discovery and design process. 1 Research Problem and Motivations Organization and planning of synthetic biology experiments is currently done almost entirely by hand. Several ongoing developments, however, are rapidly increasing the need for automation assistance in experiment planning. More and more laboratory automation is becoming available, increasing the scale and complexity of experiments that can be performed. Automation and information technology are supporting new business models with laboratory work done by technicians or outsourced to a “lab for hire.” Finally, new “multiplexing” protocols allow many tests to be conducted on a single experimental sample, and multiple experimental samples to be processed in parallel. In all of these cases, the growth in scale and complexity are rapidly outstripping the abilities of humans to create detailed experimental plans and to hand-curate the relationships between those plans and the large collections of data they generate. Furthermore, experiments are still costly both in money and time, and the space a researcher wishes to explore is often much larger than the ∗This work was supported by the Air Force Research Laboratory (AFRL) and DARPA under contract FA875017CO184. This document does not contain technology or technical data controlled under either U.S. International Traffic in Arms Regulation or U.S. Export Administration Regulations. Any opinions, findings and conclusions or recommendations expressed in this material are those of the author(s) and do not necessarily reflect the views of the AFRL and DARPA. Decision-Theoretic Hierarchical Planner
更多查看译文
AI 理解论文
溯源树
样例
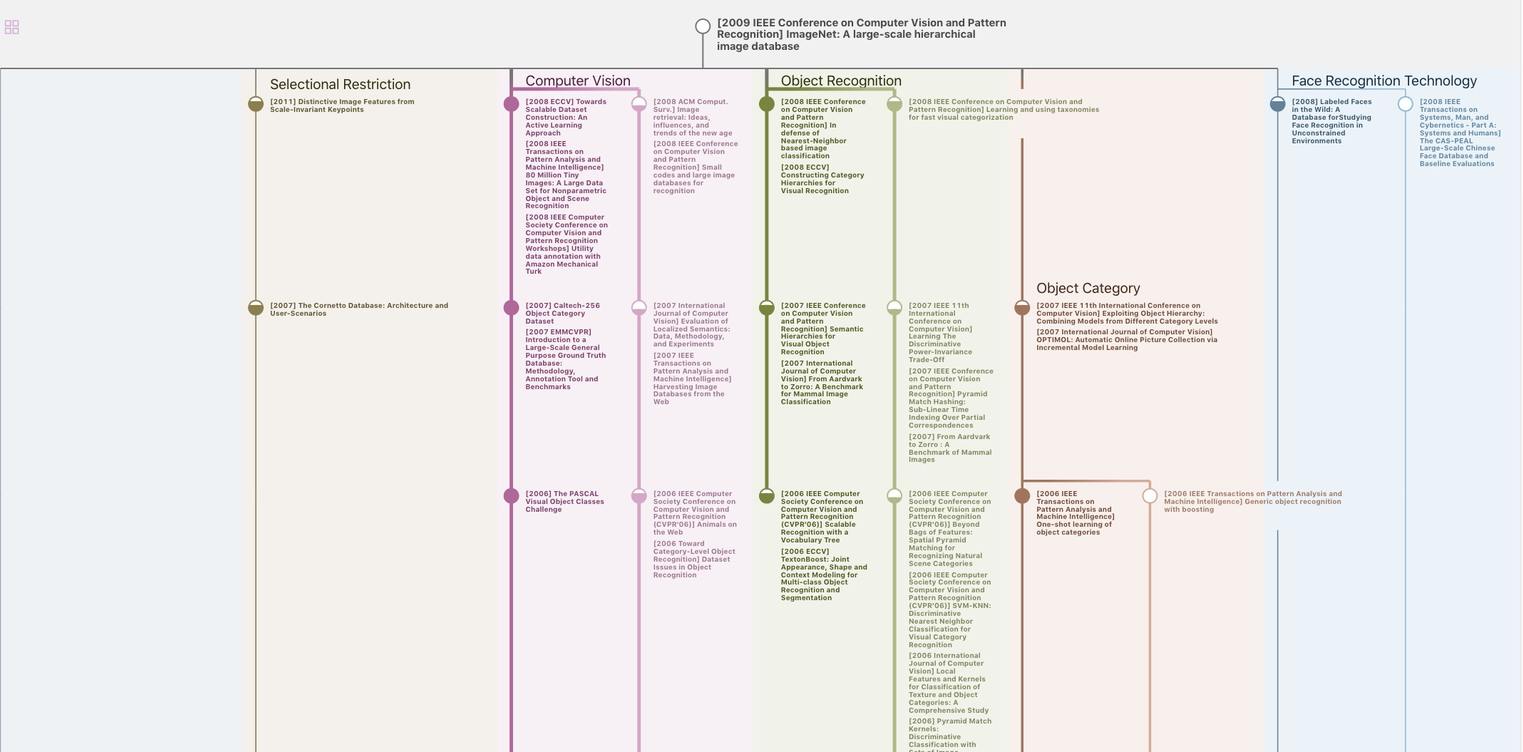
生成溯源树,研究论文发展脉络
Chat Paper
正在生成论文摘要