Predictive Maintenance of Technical Faults in Aircraft
semanticscholar
摘要
A key issue for handlers in the air cargo industry is arrival delays due to aircraft maintenance [1]. This maintenance can be planned or unplanned depending on the underlying cause : it can either be a routine check, which usually causes very little delay due to its periodicity and predictable nature, or it can also be an undetected technical failure which manifests itself during the preight check. The latter is formally known as technical delays. With the recent resurgence of arti cial intelligence techniques in decision making, especially in cases such as predictive maintenance [2], the approach followed in this work is to gather delay and maintenance data from a cargo handler in order to train a machine learning classi er that can predict if a ight will su er from an unexpected technical delay or not. In this study, a few typical machine learning techniques are explored and a novel one is also proposed. A new version of the proposed decision tree extension by Ho ait & Schyns [3], which will be referred to as con dence trees, is presented. Provided datasets by the handler are pre-processed for missing data; a subset of the available variables for each ight is selected and another subset of variables is generated based on the information available in the other datasets, such as the number of maintenances performed on the plane before the given ight. Selected machine learning models are then compared on this nal dataset comprised of 41,415 samples using a 75/25 holdout method. Each model's hyper-parameters are ne-tuned using a 5-fold cross-validation technique rst. A comparison of the regular decision trees and the proposed con dence trees highlights the advantages of the latter over the former. More speci cally, it is shown that using con dence trees signi cantly improves the interpretation of the results and thus the decision making process. The nal results of the study show that it is possible to predict aircraft technical delays with a recall of 69.9% using the aforementioned con dence trees model which is the model showing the best results in this context. Although the trained
更多查看译文
关键词
Fault Tree Analysis,Machine Mechanics,Metrological Assurance,Human Reliability Analysis,Uncertainty Handling
AI 理解论文
溯源树
样例
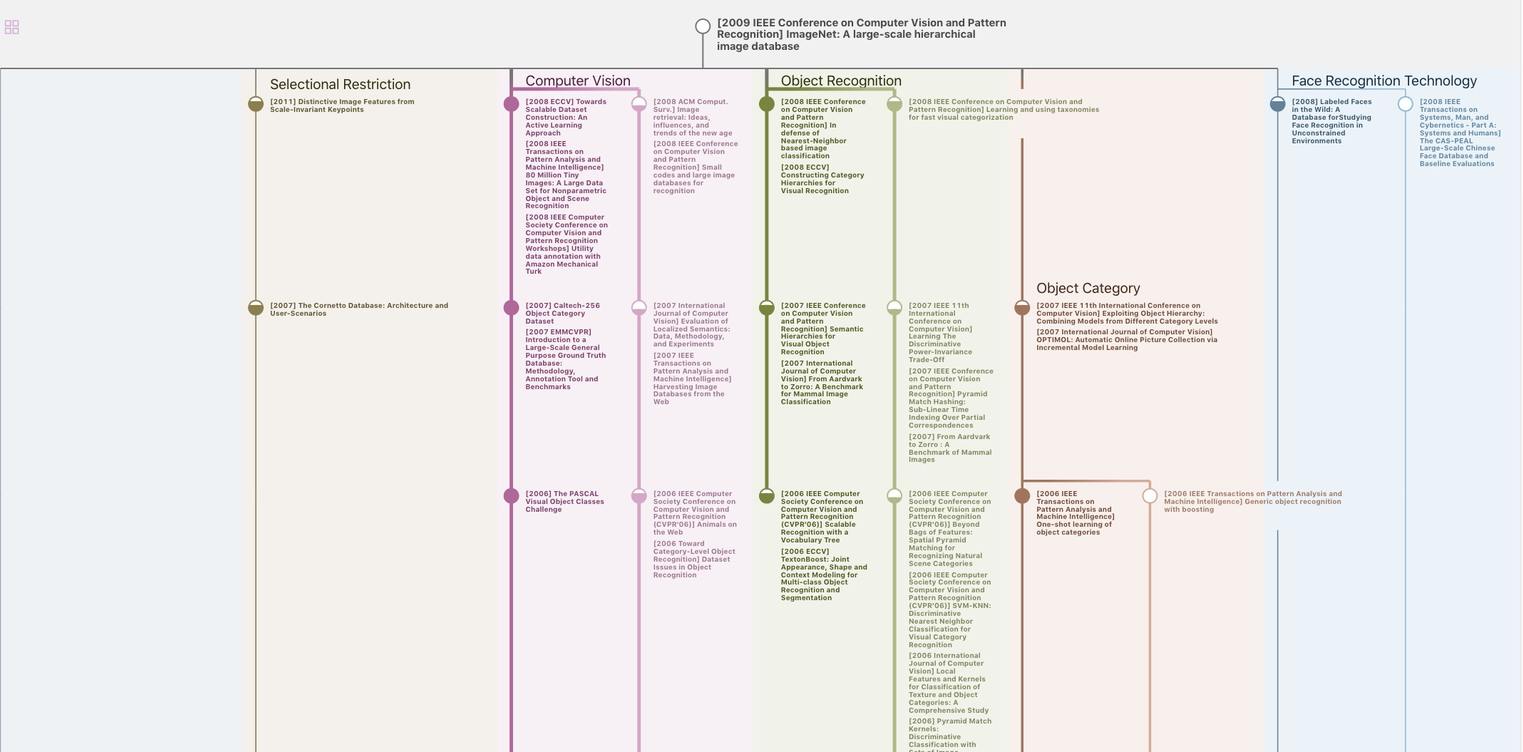
生成溯源树,研究论文发展脉络
Chat Paper
正在生成论文摘要