It ’ s QuizTime : A Study of Online Verification Practices on Twitter
semanticscholar(2019)
摘要
Misinformation poses a threat to public health, safety, and democracy. Training novices to debunk visual misinformation with image verification techniques has shown promise, yet little is known about how novices do so in the wild, and what methods prove effective. Thus, we studied 225 verification challenges posted by experts on Twitter over one year with the aim of improving novices’ skills. We collected, annotated, and analyzed these challenges and over 3,100 replies by 304 unique participants. We find that novices employ multiple tools and approaches, and techniques like collaboration and reverse image search significantly improve performance. Introduction and Related Work Today’s society is awash with misinformation. Governments and extremist groups spread false propaganda in the form of images and video, which are unwittingly shared by the public on social media. One approach towards combating these misinformation campaigns is image verification, a technique employed by expert investigators in domains like journalism and human rights advocacy (Barot, 2014). However, verification is a difficult, time-consuming task. With millions of images shared on social media every day, experts, faced with limited time and attention, are overwhelmed. Novices, too, attempt to debunk visual misinformation and help investigators (Nhan, Huey, and Broll, 2015; Europol, 2019), but are often hindered by their lack of verification and media literacy skills. Such attempts by crowds of novices can prove erroneous, leading to cases of vigilantism, such as when users on Reddit misidentified a perpetrator of the Boston Marathon Bombing (Nhan, Huey, and Broll, 2015). Researchers have studied ways to better understand expert verification practices (Brandtzaeg et al., 2016). Other work seeks to provide software tools to help novice crowds support expert investigators on verification tasks, such as image geolocation, the goal of which is to find the exact location on earth where a photo or video was taken (Kohler, Purviance, and Luther, 2017; Venkatagiri et al., 2019). Prior work (e.g., Caulfield, 2017) has studied ways to improve novices’ media literacy and verification skills. However, they were primarily controlled lab experiments, and focused on outcomes and not process (Venkatagiri and Zhang, 2018). Little is known Figure 1: A representative example of a verification quiz. about how novices learn verification skills in the wild, what tools and approaches they use, how successful they are in verifying media, and what factors affect success. Here we attempt to answer these questions by studying real-world, collaborative verification challenges (quizzes) posted on Twitter over the span of one year. We scraped all quizzes and associated conversation threads, and annotated each quiz for descriptive data and participants’ performance. We also calculated engagement metrics, such as the total number of replies and total number of unique users. Our preliminary analysis found that (1) novices employ a variety of tools and creative techniques when performing image verification; (2) a majority of participants were successful in doing so; and (3) whether they collaborated on a solution, or used certain tools, meant they were statistically significantly more successful. We conclude with a discussion of our results and avenues for future work.
更多查看译文
AI 理解论文
溯源树
样例
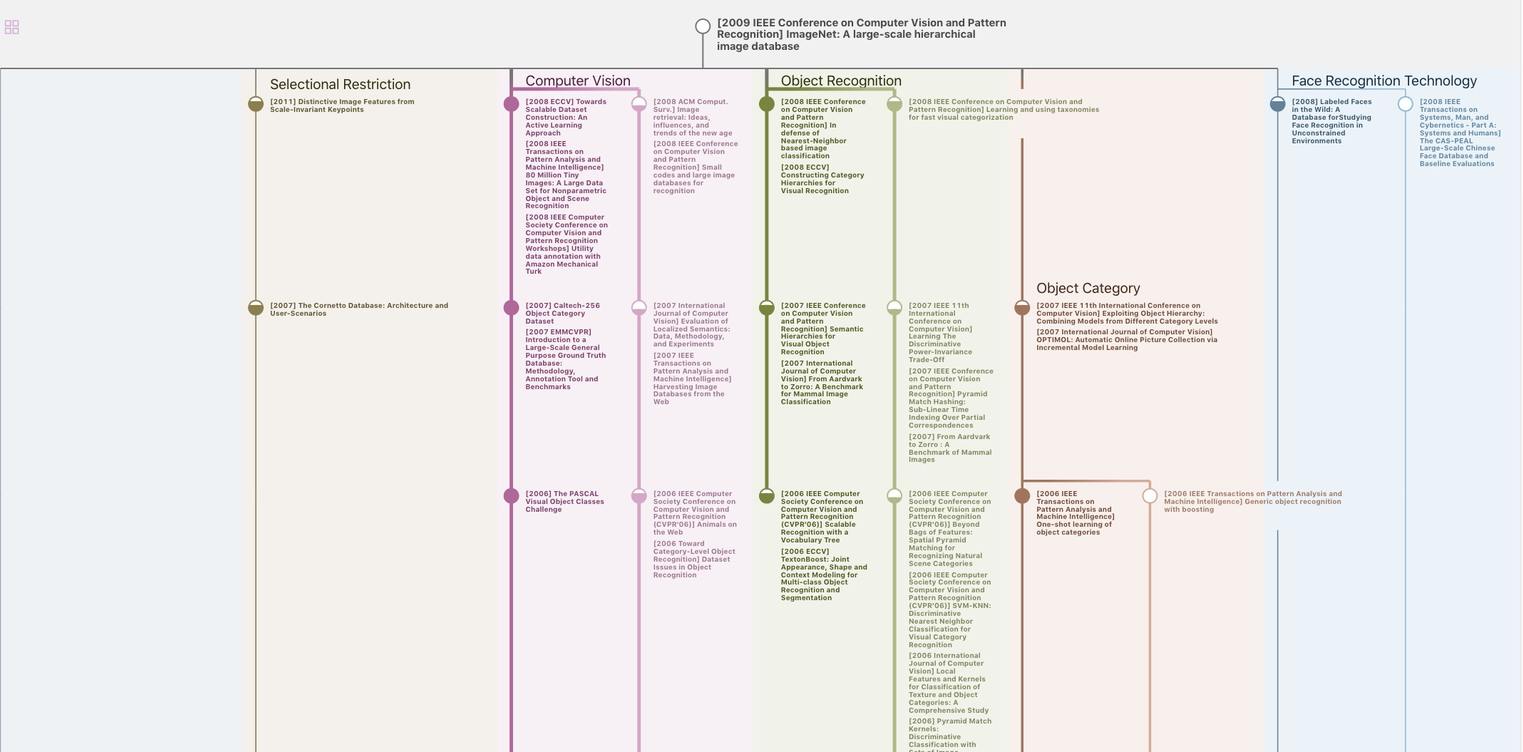
生成溯源树,研究论文发展脉络
Chat Paper
正在生成论文摘要