PROTOCOL: Effect of Early, Brief Computerized Interventions on Risky Alcohol Use and Risky Cannabis Use among Young People: Protocol for a Systematic Review
Campbell systematic reviews(2014)
摘要
Risky use of alcohol or recreational drugs among young people remains a prominent public health issue (United Nations, 2003; United Nations Office on Drugs and Crime, 2010b). The United Nations Office on Drugs and Crime (UNODC) has argued for a public health approach to prevent alcohol and recreational drug abuse, using interventions that provide assistance and counselling. This approach provides services at an early stage to drug and alcohol users who are at risk but who remain socially included (United Nations, 2003). Many countries have made substantial efforts at multiple levels ranging from government policy initiatives to primary health care services in an attempt to minimise the long-term consequences of alcohol and cannabis use. For example, Roche and Freeman (2012) have illustrated the advantages of implementing screening, and of brief, early interventions for young people with alcohol and drug problems. However, risky use of alcohol and recreational drugs remains a prominent health problem. Alcohol misuse presents a substantial societal burden due to the costs related to health care, prevention, crime, law enforcement and welfare assistance, as well as the costs resulting from reduced productivity and increased mortality (Thavorncharoensap, Teerawattananon, Yothasamut, Lertpitakpong, & Chaikledkaew, 2009). The WHO Global Survey on Alcohol and Health (2008) found a trend towards increased drinking among young people aged 18-25 years over the last five years, with an 80 percent increase in risky alcohol consumption in the participating countries (WHO, 2011). Risky and harmful drinking patterns such as binge drinking and drinking to intoxication have also increased over time among young people (Editorial Lancet, 2008; The National Center on Addiction and Substance Abuse at Columbia University, 2007). Small to moderate levels of alcohol might not be harmful, but high consumption of alcohol is directly related to risky behaviours such as intoxicated driving (Cherpitel, Ye, Bond, & Borges, 2003) and interpersonal violence (Foran & O'Leary, 2012). In addition, binge drinking can have both short- and long-term negative impacts on an individual's health. Lopez-Caneda et al. (2013), for instance, found an association between binge drinking and anomalous neural activity related to working memory processes, and college students who binge drink have been shown to have a higher risk of developing alcohol dependence in later years (Jennison, 2004). Cannabis is the most widely used and trafficked illicit drug worldwide (United Nations Office on Drugs and Crime, 2012). Cannabis is a general term to describe the psychoactive preparations of the plant Cannabis sativa. While marijuana refers to the cannabis leaves or other crude plant material, the term hashish describes the drug produced by drying the resin exuded by the marijuana plant (Brecher, 1972). Cannabis is commonly smoked, with or without being mixed with tobacco – but can also be consumed orally. The United Nations Office on Drugs and Crime (UNODC) has estimated that between 2.9 to 4.3 percent of the world population aged 15-64 (between 129 and 191 million people) used cannabis at least once in 2008 (United Nations Office on Drugs and Crime, 2010a). Whereas the use of inhalants (defined as substances producing chemical vapours such as found in beauty products like hairspray) is more commonly used among young people and might decline with age, the use of marijuana and hashish increases with age (Mosher, Rotolo, Phillips, Krupski, & Stark, 2004). Cannabis use has increased in the U.S. since 2007 in the 13 to 18 year age group and daily marijuana use reached a 30-year peak among high school students aged 17-18 years in 2011 (Johnston, O'Malley, Bachman, & Schulenberg, 2012). Regular cannabis use among young people presents a social burden due to costs arising from increased health care use and a higher risk for school-drop out. Although there appears to be no standard measure for ‘low’ or ‘high’ frequencies of cannabis use, we define ‘high users of cannabis’ according to the literature as users with at least weekly consumption of the drug (Lev-Ran, Le Foll, McKenzie, George, & Rehm, 2013). It has been reported that regular cannabis use among young people may have a negative impact on their cognitive functioning (Ramaekers, Berghaus, van Laar, & Drummer, 2004; Ramaekers et al., 2006), and on the developing brain (Pope, Grubera, Hudsona, Cohanea, & Yurgelun-Toddb, 2003). The current literature suggests a positive association between frequency of cannabis use and the risk of developing a mental illness, especially psychotic disorders (Løberg et al., 2012). On the other hand, there is some evidence that cannabis use can have a positive impact on creativity (Schafer et al., 2012), and is helpful in the treatment of certain ailments such as cancer (Robson, 2001) and multiple sclerosis (Iskedjiana, Berezaa, Gordonc, Piwkoa, & Einarsona, 2007). Other risk factors including unprotected sexual behaviour and risky driving behaviour have been found to be associated with increased use of alcohol (Jennison, 2004; Karam, Kypri, & Salamoun, 2007; J. Miller, Naimi, Brewer, & Jones, 2007) and of cannabis (Anderson & Stein, 2011; Hall & Degenhardt, 2009). In addition, the development of substance abuse disorders in later life has been found associated with the use of alcohol (Odgers et al., 2008) and cannabis (Behrendt, Wittchen, Hofler, Lieb, & Beesdo, 2009) during youth. Thus, there is a need for early interventions to reduce or eliminate the use of alcohol and cannabis among young people in order to prevent them from falling into a downward spiral that may lead to substance abuse related behaviours and ailments in adulthood. Brief interventions have the singular focus of targeting problematic behavior in a systematic and specific manner (O'Leary & Monti, 2004). For the purpose of this review, brief interventions are defined as follows: any preventive or therapeutic activity (delivered by a health worker, psychologist, social worker, or volunteer worker) given within a maximum of four structured therapy sessions, each of short duration (W. Miller, Zweben, DiClemente, & Rychtarik, 1992) that lasts between five and ten minutes with a maximum total time of one hour (Babor, 1994). Previous reviews suggest that ‘brief interventions’, so defined, can be effective in reducing the burden of alcohol (Rehm et al., 2004), and cannabis (Bernstein et al., 2009) use. The National Institute for Health and Clinical Excellence (NICE) differentiates between two main types of brief interventions, namely Structured Brief Advice and Extended Brief Interventions. Structured Brief Advice can be used with time constraints (e.g., 5 minutes) as a first step for adults (aged 18 and over) who have been classified as high-risk drinkers. In contrast, most Extended Brief Interventions can be classified as short versions of Motivational Interviewing (NICE, 2010). Examples are the ‘Motivational Enhancement Therapy’ originally developed as a four-session intervention in ‘Project MATCH’ in the US (W. Miller et al., 1992), and ‘Drinker's check-up’ (Hester, Squires, & Delaney, 2005; W. Miller, Sovereign, & Krege, 1988; NICE, 2010) consisting of assessment, feedback, and decision-making modules. Computerized brief interventions include both online and offline interventions (e.g., CD-Rom, software, websites and downloadable applications) delivered via electronic devices such as personal computers, tablets and smart phones. The main advantage of a computerized brief intervention is that it can reach large audiences at a low cost and simultaneously simulate an ‘interpersonal therapeutic component’ by targeting recipients' feedback. Moreover, computerized, brief intervention appeals to younger people who have been growing up with digital media. Many studies targeting young people such as high school and university students use computerized interventions (Carey, Carey, Henson, Maisto, & DeMartini, 2011; Carey, Scott-Sheldon, Elliott, Bolles, & Carey, 2009; Carney, Bronwyn, & Louw, 2011; White et al., 2010). As young people are underrepresented among users of standard face-to-face alcohol and other drug specialist services, the computer might be an effective medium to reach this population (White et al., 2010). In one study, 53 percent of Internet users aged 18-29 had searched online for information on a specific disease and medical problem (Fox & Duggan, 2013), and 14 percent had searched specifically for information on alcohol and drug problems (Fox, 2006). Computerized interventions often consist of two feedback components: targeted feedback and tailored feedback (W. Miller, 2002). Whereas the term ‘targeted feedback’ refers to feedback according to the needs of a whole group, for example, to students with risky alcohol and cannabis use, the term ‘tailored feedback’ refers to feedback that is individualized and tailored to a single-person's needs (Kreuter & Skinner, 2000). ‘Automated’ computerized interventions may be combined with a brief session of counseling given by a real time ‘counselor’ such as a psychologist or social worker at the other end of the electronic link (Kristjansdottir et al., 2013). In the case of early, brief computerized interventions, software programs can be used instead of health care professionals or other staff to screen effectively for substance use. This type of screening process is more anonymous and may thus encourage participants to give more honest information. Interventions that are consistent and of high quality can be provided via computers, tablets, or smart phones (including using the Internet) and can also give information tailored to the individual participant (Moyer & Finney, 2004/2005). The assessment module aims to classify the user as either a low, medium, high or very high risk drinker and provides recommendation on whether he or she might benefit from a more formal treatment program. The feedback module gives information on the user's score after each assessment and responds to the client's general reaction to such feedback. Initially, the decision-making module allows users to specify their level of readiness to change. Those who declare themselves ready to change are provided with a menu of goal options. After deciding which goal option to follow, users are lead through exercises to develop a plan of change, and are also provided with references to additional Web links, self-help groups and materials and a list of therapists. Those who do not show a readiness to change are offered the option of receiving some basic information before ending the program (Moyer & Finney, 2004/2005). Gender and education might influence the effect of brief, computerized interventions. For example, males and young adults with higher education use digital media more than females and young adults with lower education (OECD, 2008). These two groups may therefore be more likely to benefit from computerized interventions because their past experience is likely to have led to more efficient use of digital media. Brief interventions have been suggested as working through two main mechanisms: (1) by making the clients think differently about their alcohol/cannabis use, and (2) by providing them with skills to change their behavior if they are motivated to change. It has been suggested that the assessment component of brief interventions alone might lead to behavioral change (Bien, Miller, & Tonigan, 1993) particularly in emergency department settings (Longabaugh et al., 1995). In addition, studies drawing on time-line follow-back assessments have shown some reductions in the use of alcohol and other substances over time (LaBrie, Lamb, Pedersen, & Quinlan, 2006; Suffoletto, Callaway, Kristan, Kraemer, & Clark, 2012). Previous reviews and meta-analyses using “Motivational Interviewing” (MI) (Smedslund et al., 2011), internet-based interventions (Tait & Christensen, 2010) and online alcohol interventions (White et al., 2010) have studied the effects of computerized brief interventions delivered both as stand-alone or in combination with face-to-face interventions. First, Smedslund et al. (2011) focused on the effect of ‘motivational interviewing’ in general on substance abuse among persons who abused or were dependent upon substances, and included all individuals who met this criterion without limitation to age. Second, in examining the effect of fully automated Internet-based interventions, Tait and Christensen (2010) limited their review to studies targeting young people not older than 25 years with problematic substance use, and they did not explicitly differentiate between specific substances, and focused solely on brief computerized interventions. Third, White et al. (2010) included studies on the effect of online-alcohol interventions more generally, without limitation to age and time range. In general, these reviews have focused either on the universal prevention of problematic substance use, or on the treatment and rehabilitation of individuals who have established substance dependency. Most focus solely on computerized interventions, are limited to college students, and exclude other groups of young people who are not attending college. The current review investigates whether stand-alone early, brief computerized interventions prevent the development of established alcohol and/or cannabis problems in young people aged 15-25 years showing risky behavior. This has not been systematically studied before. The objective of this review is to assess the effectiveness of early, brief computerized interventions on alcohol and cannabis use by young people aged 15 to 25 years who are high or risky consumers of either one or both of these substances by synthesizing data from rigorous high-quality studies. We will include studies where units (e.g., persons, therapists, institutions) are allocated randomly or quasi-randomly to an early, brief computerized intervention and at least one other comparator condition. Both efficacy studies (where the treatment is studied under ideal conditions) and effectiveness studies (where the treatment is studied under real-world conditions) will be included. We will include studies where early, brief computerized interventions are used as a stand-alone treatment. Eligible comparators include no intervention, waiting list control or alternative brief intervention, which may be computerized or delivered face-to-face. We will exclude studies using non-randomised procedures for allocation (such as self-selection). Examples of eligible studies include that conducted by Voogt, Poelen, Kleinjan, Lemmers, and Engels (2011) suggesting the effectiveness of a web-based brief alcohol intervention in reducing heavy drinking among young people aged 15 to 20 years with a low educational background, the study by Voogt, Poelen, Lemmers, and Engels (2012) illustrating the effectiveness of a web-based brief alcohol intervention in reducing heavy drinking among college students, and the study conducted by Bingham et al. (2010) on the efficacy of a web-based, tailored, alcohol prevention/intervention program for college students. The review will include studies in which the participants are young people between 15 and 25 years of age who are high or risky consumers of alcohol or cannabis, or both. We will include studies of university students and of senior high school students even if no further information on age is provided. We will exclude studies that state only that the participants were young. High or risky consumption of alcohol is defined as either (a) consuming at least five (for males) or four (for females) drinks during any one drinking session, or (b) consuming more than fourteen (for males) or more than seven (for females) drinks a week (National Institute on Alcohol Abuse and Alcoholism, 2013). In the US, a standard drink is defined as one which contains about 0.6 fluid ounces or 14 grams of ‘pure’ alcohol. High or risky consumption of cannabis is defined in different ways; whereas some scholars view risky consumption of cannabis as daily or near-daily use (Fischer et al., 2011), others use a broader definition to include those who consume cannabis at least once a week (Webb, Ashton, Kelly, & Kamali, 1996). In this review, we define risky cannabis use among young people as the frequent consumption of cannabis at least once a week. Existing studies on the effect of brief computerized interventions on risky drug use have usually defined young people in a range 15 to 25 years (Bingham et al., 2010; Voogt et al., 2011; Voogt et al., 2012), and we have limited our target group accordingly. If we find studies comprising interventions to reduce the use of other types of substances simultaneously (e.g. cocaine), we will exclude them unless they analyse results on risky alcohol or cannabis use separately. If the study includes young people over 25 years of age, the mean age should be 25 years or less. If the study includes young people under 15, the mean age should be not be less than 15 years. The definition of ‘young people’ might vary in different countries and cultures. In addition, the debut age for using alcohol and cannabis is assumed to vary between different countries. For the purposes of this review, we define ‘early intervention’ as being delivered at an early stage of substance use (an ‘indicated prevention’). An ‘indicated preventive strategy’ targets individuals at high-risk who have been identified as having minimal but detectable signs foreshadowing alcohol and cannabis abuse (O'Connell, Boat, & Warner, 2009). Early, brief computerized interventions appear to be an important tool to stop the development of severe alcohol and cannabis use among young people at risk. This review will include all types of early, brief computerized interventions regardless of medium, provider or theoretical framework. These may be ‘automatic only’ or delivered with the involvement of trained real-time ‘counselors’ (e.g., social workers, psychologists, or other health care workers). Automatic interventions and interventions with trained real-time counselors will be analyzed separately. Studies with booster sessions will be included but analyzed separately. This review will only include ‘brief’ interventions defined as any preventive or therapeutic activity (such as is delivered by a health worker, psychologist, or volunteer worker) given within a maximum four structured therapy sessions, each of short duration (W. Miller et al., 1992) that typically lasts between five and ten minutes with a maximum total time in treatment of one hour (Babor, 1994). The comparator condition can be: an alternative early, brief intervention, no intervention or waiting list control. We will compare changes in use (e.g. frequency, quantity or peak consumption, occasions, drinking days) between intervention and comparators at baseline and at all follow-ups. Alcohol use and cannabis use will be analyzed separately. The exact duration of follow-up will be recorded for each study. We will search for on-going studies in ClinicalTrials.gov and will contact experts in the fields to identify unpublished reports, on-going studies and studies that were not retrieved in the bibliographic search. We will conduct a hand-search of the tables of contents of the Journal of Consulting & Clinical Psychology for the years 2012 to present and will search all conference proceedings from INEBRIA (International Network on Brief Interventions for Alcohol & Other Drugs). Finally, we will examine the reference lists of included studies and relevant systematic reviews in the field. If there are studies in which more than one eligible intervention group is compared with a single control group, we will only include one intervention to avoid using the control group more than once in the same meta-analysis. If two types of early, brief computerized interventions are compared with an inactive control intervention, we will include the most comprehensive intervention. If one type of early, brief computerized intervention is compared with, both, a non-computerized early, brief intervention and an inactive control intervention, we will select the inactive control intervention. If there are several follow-up times, we will analyse the data from each separately. If multiple measures are used to represent a primary outcome in the same study, we will either use standardized mean difference (Hedges' g) in order to obtain a common metric. The screening of studies will proceed in two phases. At Level 1, two review authors will scan the title and abstract of each reference and score either “promote to next level”, “exclude”, or “can't tell”. Only if both review authors score “exclude” will the reference be excluded at this Level. If at least one review author scores ”can't tell” or “include”, the reference will be promoted to Level 2. References promoted to Level 2 will be ordered in full text and the same screening criteria will then be applied. Two review authors will read the full texts and score “include” or ”exclude”. If there is disagreement, a third review author will decide whether to include the study. We will not calculate the inter-rater reliability of individual coders' ratings, but we will pilot a small number of references and discuss the screening practices as a group in order to develop high agreement between raters before we screen the rest of the studies. Data from each study will be extracted by two review authors using a specifically developed data extraction form (see Appendix 8.2) to record detailed information about authors, institutions, journal, participants, intervention, control conditions, research design, sample size, outcomes and results. We will apply the same rules for dealing with disagreement as described under 3.3.2. In a properly randomized study, all initial differences between groups will arise by chance. This applies to all prognostic variables, both known and unknown. In non-randomized designs, however, there may be important initial differences between groups. These differences can be systematic, and can appear in unmeasured variables as well as in the measured ones. It is generally possible to control for measured variables but not for unmeasured ones. Regression methods can be used after the intervention to control for initial differences, but such methods may introduce bias in the results (Deeks et al., 2003). We will compare the outcomes of treatment and control groups at post-test and at short-, medium- and long-term follow-up. For dichotomous data, we will report relative risks (risk ratios), and for continuous data, we will report standardised mean differences. We will use 95 percent confidence intervals as measures of the random error influencing the outcome estimations. We will use the optimal information size (OIS; Poque and Yusuf (1997)) to assess whether the sample size is sufficient for concluding that there is a statistically significant overall effect in a meta-analysis. Using a two-sided alpha of 0.05 and power of 0.95 we calculate that a total sample size of 1,302 is necessary for detecting a small standardised mean difference (SMD) of 0.2. For SMDs of 0.5 (medium) and 0.8 (large), the OIS are 212 and 84, respectively. In cluster-randomized trials one has to be careful to avoid unit-of analysis errors. The following may serve as an example: If the population of the study consists of a total of 100 risky alcohol users, distributed in four schools with 25 in each school, and two schools are randomized to the intervention and the other two to the control, the correct N to use in the analyses is not 100, but smaller. The effective sample size of a single intervention group in a cluster randomized trial is its original sample size divided by a quantity called the design effect. A common design effect is usually assumed across intervention groups. The design effect is 1 + (m - 1)r, where m is the average cluster size and r is the intra-cluster correlation coefficient (ICC). If we include any cluster randomized controlled trials in this review, we will attempt to measure the intra-cluster correlation. The total variance in the outcome can be partitioned into variance between groups (VBG) and variance within groups (VWG). The intra-cluster correlation is calculated as VBG/ (VBG+VWG). The number of participants can be used in the analyses if the ICC is used as a correcting factor; however, the ICC is seldom reported in primary studies. For dichotomous data both the number of participants and the number experiencing the event can be divided by the same design effect (Higgins & Green, 2011). If the ICC is not reported in primary cluster randomised studies we will consult a statistician for advice. Where not available from the published reports, we will contact authors by email to request any missing data or the reasons for missing data to allow a judgement to be made on whether these are ‘missing at random’ or ‘not missing at random’. Data will be considered ‘missing at random’ if the reason for absence is judged as unrelated to actual values of the missing data. In contrast, data will be considered ‘not missing at random’ if the reason for absence is judged as related to the actual missing data. Where data are not missing at random, we will analyse only the available data. Where data are missing at random, we will seek the advice of a statistician and will impute the missing data with replacement values, and treat these, as if they were observed. The method of imputation will be clarified after consulting a statistician. Effect sizes will be calculated from means, standard deviations and N. Where these are not reported in sufficient detail, we will contact the authors of the primary studies. If this is unsuccessful, we will attempt to retrieve effect size data from published meta-analyses, or to calculate effect sizes using RevMan or CMA software from information such as t-values. If these strategies are unsuccessful, we will attempt to use the method described in Section 16.1.3 of the Cochrane Handbook (Higgins & Green, 2011). If means but not SDs are provided, we will consider imputing SDs from other similar studies. We will assess for heterogeneity among primary outcome studies using the I2 and τ2 statistics (Higgins & Green, 2011) and will discuss any observed heterogeneity and its magnitude. We will use funnel plots to provide information about possible publication bias if there are more than ten included studies. We are aware, however, that asymmetric funnel plots are not necessarily caused by publication bias (and publication bias does not necessarily cause asymmetry in a funnel plot). If asymmetry is present, likely reasons will be explored. Further, we will search for pre-published trial protocols and will compare the published report with its corresponding protocol where this is available. Meta-analysis will be considered appropriate if the same treatments are compared to the same comparators and if the studies do not have high risk of bias. We will discuss in each case whether meta-analysis is appropriate until we reach consensus. If meta-analyses are performed, we will report results using random-effects models because we expect that the studies will be clinically heterogeneous regarding participants, settings, interventions and outcomes. If meta-analyses are not judged to be appropriate, we will report the results for each individual study by narratives. All analyses comparing a brief intervention with an alternative brief intervention (two active interventions) will be presented separately. We will consider conducting separate analyses by control group type for comparisons between different passive interventions, and will use moderator analysis to explore differences in mean effects across control group type. We will also investigate the effect of baseline frequency of use/dose. We will analyse effects separately for studies including targeted feedback only, and for studies, including both, targeted and tailored feedback. The studies will be analysed separately for the different time points. If there are sufficient primary studies, we will classify them according to these variables in attempt to identify possible sources of heterogeneity. We will consider performing moderator analyses (e.g., stratification on subgroups, meta-analysis analogue to ANOVA, meta-regression) to explore how observed variables are related to heterogeneity. We will check for the robustness of the observed findings by examining the effect of limiting the studies to be included to those with low RoB. Meta-analyses will be performed in RevMan, but we will consider conducting meta-regression in Comprehensive Meta-Analysis if the number of studies is sufficient. The systematic review was supported by the Norwegian Knowledge Centre and the University of Toronto, Factor-Inwentash Faculty of Social Work. All the authors (Sabine Wollscheid, Lin Fang, Wendy Nilsen, Geir Smedslund, Asbjørn Steiro, Karianne Thune Hammerstrøm and Lillebeth Larun) designed the review question and assisted in writing the protocol. Wendy Nilsen and Sabine Wollscheid wrote the background of the protocol. Asbjørn Steiro and Lillebeth Larun operationalized the inclusion criteria; Lillebeth Larun worked in particular on overall revisions of the manuscript. Geir Smedslund and Sabine Wollscheid wrote the methods section. Lin Fang provided expert input in on web-based and brief interventions. Karianne Thune Hammerstrøm developed the research strategy. No conflict of interests known. Screening level one (on the basis of title and abstract) Reference id.no: Study id.no: Reviewer's initials: Year of publication: We will exclude the study if one of the answers to the questions 1-4 is negative. If the answers are affirmative or uncertain, we will retrieve the full report for second level screening. When reading the full text article, we will consider again any questions where the answer was recorded as uncertain during the first level screen. In cases where the published information is insufficient or where the reporting is unclear, we will contact the author of the study. Primary author (publication year): Reviewer's initials, date (year/month/day) Study design Randomized controlled trial (RCT) Cluster RCT Quasi-RCT Link to protocol Methodological Quality (Risk of bias assessment). Risk of Bias (assess as low, high, unclear) Sequence generation Allocation sequence concealment Blinding of participants Blinding of personnel Incomplete outcome data Selective outcome reporting Other potential threats to validity http://handbook.cochrane.org/chapter_8/8_assessing_risk_of_bias_in_included_studies.htm Study Characteristics Country Setting Methods of recruitment to the trial Recruitment follow-up Criteria for identifying eligible participants Inclusion criteria Exclusion criteria First year of data collection Last year of data collection Data collection method Participants Number randomized Gender Age Education Employment Socio-economic status (parents' education) Consumption of cannabis Consumption of alcohol Other Intervention Delivery method Aim Explanation Theoretical framework/Background Feedback: tailored/targeted Number of sessions Duration of sessions Time between sessions Control Other brief intervention No intervention Placebo Waiting list Treatment fidelity Treatment fidelity will be coded by using an adapted version of the Oxford Implementation Index (Montgomery, Underhill, Gardner, Operario, & Mayo-Wilson, 2013) Outcome measurements Primary outcomes Alcohol use Cannabis use Secondary outcomes Motivation for change Depression and anxiety Adverse outcomes: Any reported adverse outcomes Results State where found in the article
更多查看译文
AI 理解论文
溯源树
样例
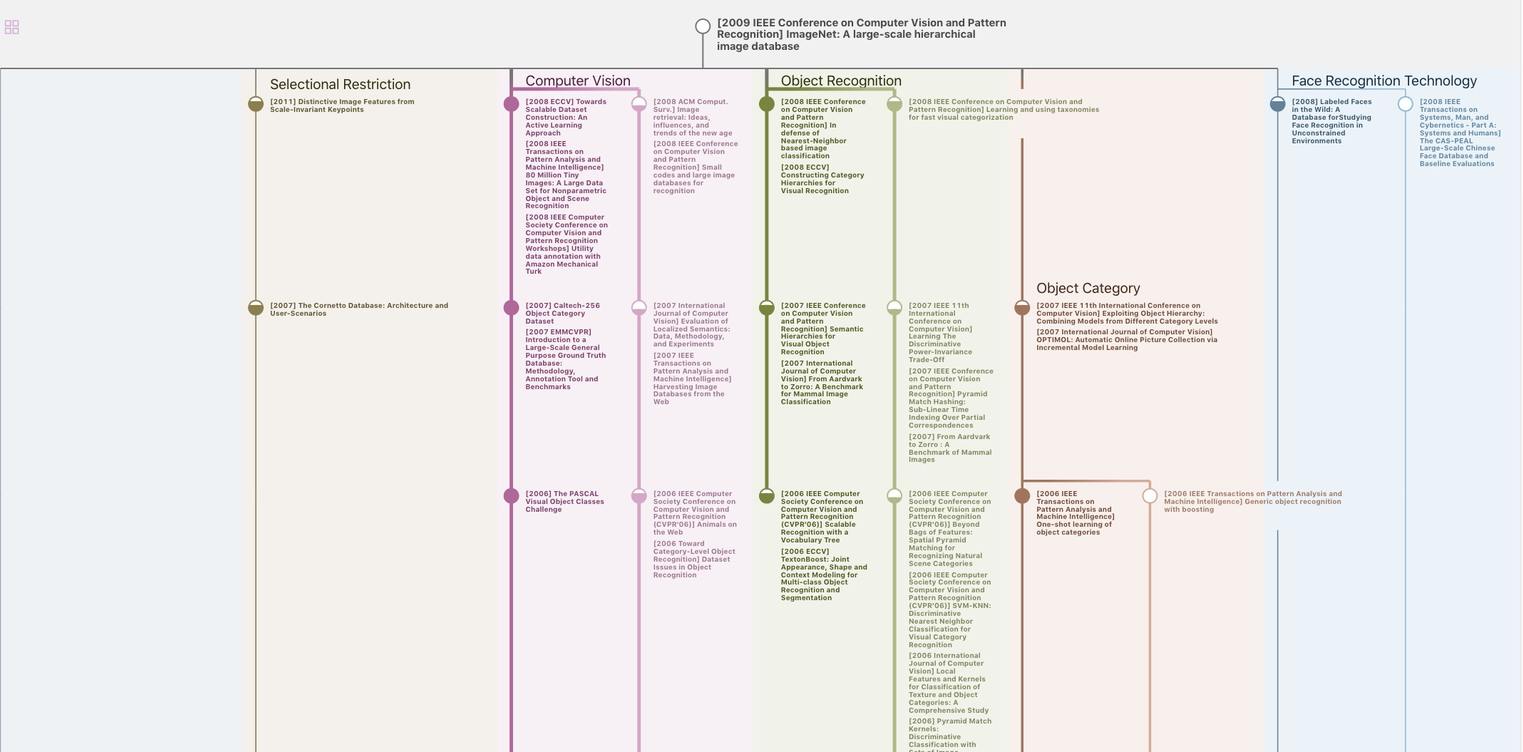
生成溯源树,研究论文发展脉络
Chat Paper
正在生成论文摘要