A User-aware Clicking Platform for Recommenders
semanticscholar(2016)
摘要
Recommenders are becoming one of the main ways to navigate the Internet. They recommend appropriate items to users based on their clicks, i.e., likes, ratings, purchases, etc. These clicks are key to providing relevant recommendations and, in this sense, have a significant utility. Since the user clicks reflect the preferences of users, they may raise privacy concerns. Intuitively, the effects on utility and privacy are not the same for all clicks. For the first time, we quantify the exact utility and privacy effects of each user click. Interestingly enough, we show that against the common belief of a trade-off between utility and privacy, they are not always antinomic regarding clicks. An appealing application of our measures is what we call a clickadvisor diagram, enabling users to decide whether it is worth clicking on an item or not. 1. METHODS 1.1 The utility of a Click We quantify the precise effect of a click on recommender utility by introducing the notion of commonality of a user profile i.e. how close the taste of a user is to that of other users (which helps a recommender suggest new items to that user). Intuitively, commonality captures the precision of recommenders. The difference between the commonality of a user profile before and after a click corresponds to the notion of the utility of that click. 1.2 The disclosure Risk of a Click To compute the privacy effect of a click, we introduce the concept of disclosure degree of a user profile, using an information-theoretic approach. The disclosure degree corresponds to the amount of information stored in a user profile, also known as entropy [3]. Roughly speaking, the larger the amount of information in a user profile, the higher the disclosure degree of the user. Intuitively, if the disclosure degree of a user profile is low, then the user is not easily distinguishable from others. We then capture the disclosure Figure 1: How does a click-advisor work? (a) A user gets the recommendations. (b) The user preclicks on the recommended items based on his real preferences. (c) Based on the zone of a click in the click-advisor diagram, the user decides to confirm, cancel or change the click. risk of a click, implying its privacy effect, as the difference between the disclosure degree of a user profile before and after the click. The click-advisor diagram We demonstrate that, the traditional belief of a trade-off between utility and privacy [5, 6], (i.e.) a user necessarily improves recommendation utility at the expense of compromising privacy (or vice versa), does not always hold. On the contrary, we show that there are some (safe) clicks that can improve both utility and privacy on the one hand and (dangerous and deleterious) clicks that do not improve utility, yet they hamper privacy on the other hand. The rest of the clicks are said to be in the trade-off zone. We precisely compute the conditions for a click to be in each zone. We show how to depict the zones of all clicks, at a click time, in what we call the click-advisor diagram. A click-advisor diagram can be viewed as a tool to help users decide whether or not to actually click on an item. The process is as follows: a user (a) pre-clicks on an item, (b) gets the feedback on the utility and privacy effects of all possible clicks for rating based on the real preference of the user shown in that pre-click and then (c) decides to confirm, change or cancel the pre-click.
更多查看译文
AI 理解论文
溯源树
样例
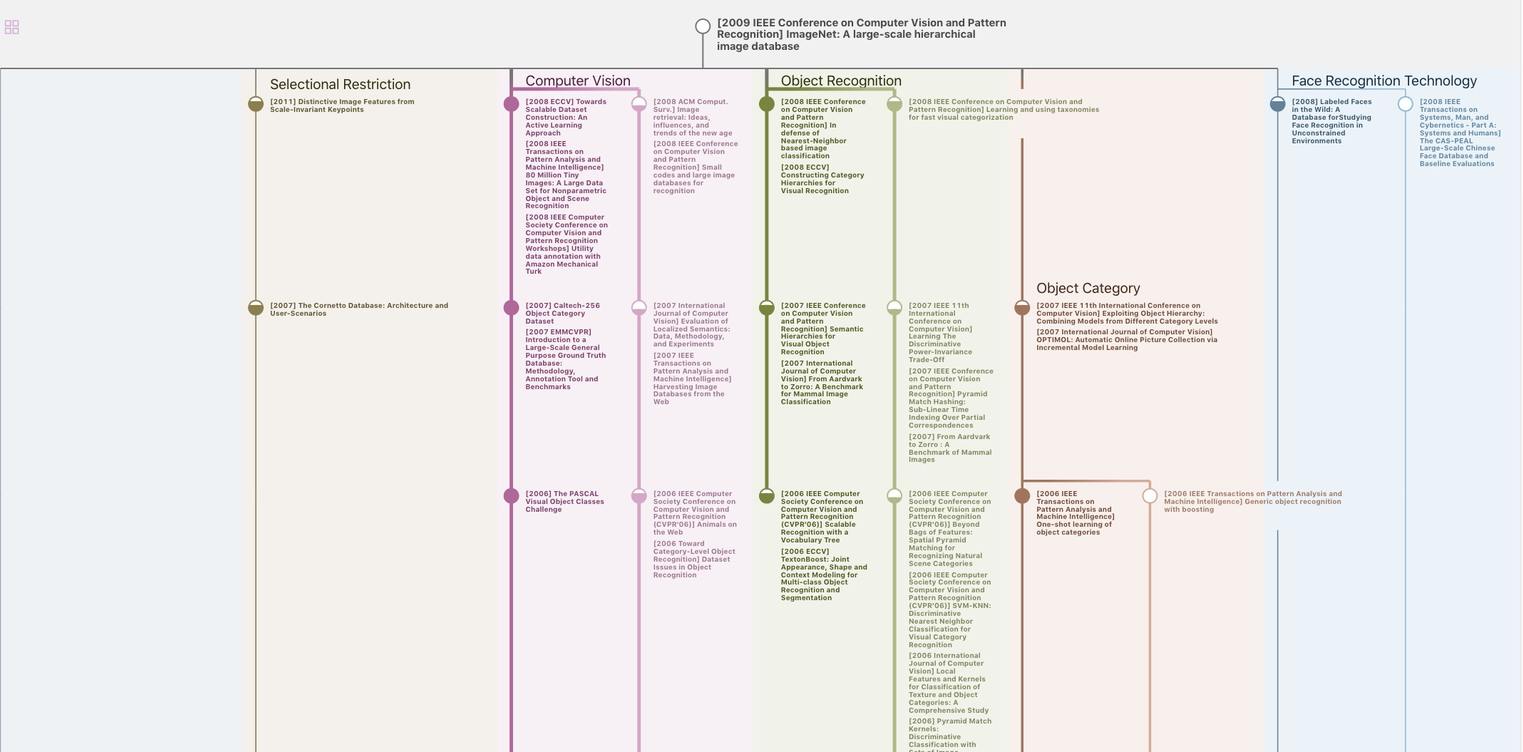
生成溯源树,研究论文发展脉络
Chat Paper
正在生成论文摘要