Cross-lingual Natural Language Modeling
semanticscholar(2019)
摘要
We focus on a particular type of language modeling which is known as cross-lingual language modeling because it suffers both from data sparsity and domain adaption making it very challenging and meaningful as a research topic. While the neural network brings about performance gains, it has not successfully addressed these two issues since the approach is essentially data-driven. In cross-lingual language modeling, we seek to find the common structures in both languages, so that learning one language also helps us in learning another language which could potentially be under-resourced. We focus our work on the study of code-switching (CS) language whereby the speaker is free to use either language, we seek to find the common structure or mapping space which help us to model both languages effectively. In both cases, the data is usually sparse, due to the lack of high-quality alignment or the low occurrence of such data under the natural circumstance. Code-switching or code-mixing is an increasingly common linguistic behavior among bilingual speakers [1]. Intra-sentential CS speech poses a significant challenge to ASR systems [2]. This is because CS introduces more vocabulary choices at each prediction step due to words from another language, at the same time, it occurs sparingly and freely without adhering to rigid syntactic or grammatical rules [3]. Speaker may choose when to and not to switch given the same preceding context. The challenge is further exacerbated because there are far less CS linguistic resources than monolingual ones. In general, we rely on large text corpora in written form, such as newspapers and books, for monolingual language modeling. As CS takes place mostly in spoken form, we cannot find as much documented CS text as monolingual text for language modeling.
更多查看译文
AI 理解论文
溯源树
样例
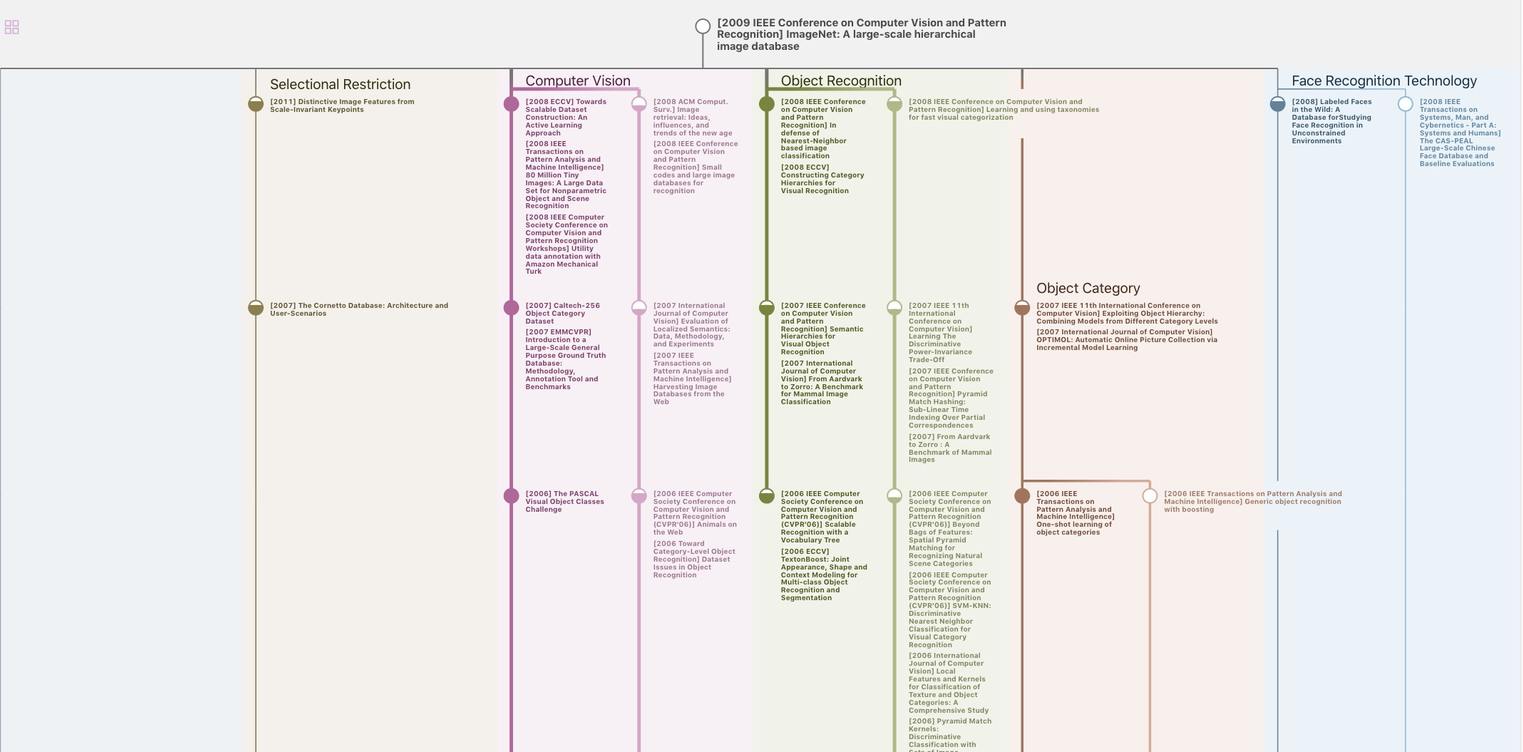
生成溯源树,研究论文发展脉络
Chat Paper
正在生成论文摘要