Actor-Critic PAC Robust Policy Search
semanticscholar(2019)
摘要
This work studies an approach for computing provably robust control laws for robotic systems operating in uncertain environments. We develop an actor-critic style policy search algorithm based on the idea of minimizing an upper confidence bound on the negative expected advantage of a control policy at each policy update iteration. This new algorithm is a reformulation of Probably-Approximately-Correct Robust Policy Search (PROPS) and, unlike PROPS, allows for both step-based evaluation and step-based sampling strategies in policy parameter space, enabled by the use of Generalized Advantage Estimation and Generalized Exploration. As a result, the new algorithm is more data efficient and is expected to compute higher quality policies faster. We empirically evaluate the algorithm in simulation on a challenging robot navigation task using a high-fidelity deep stochastic model of an agile ground vehicle and compare its performance to the original trajectory-based PROPS.
更多查看译文
AI 理解论文
溯源树
样例
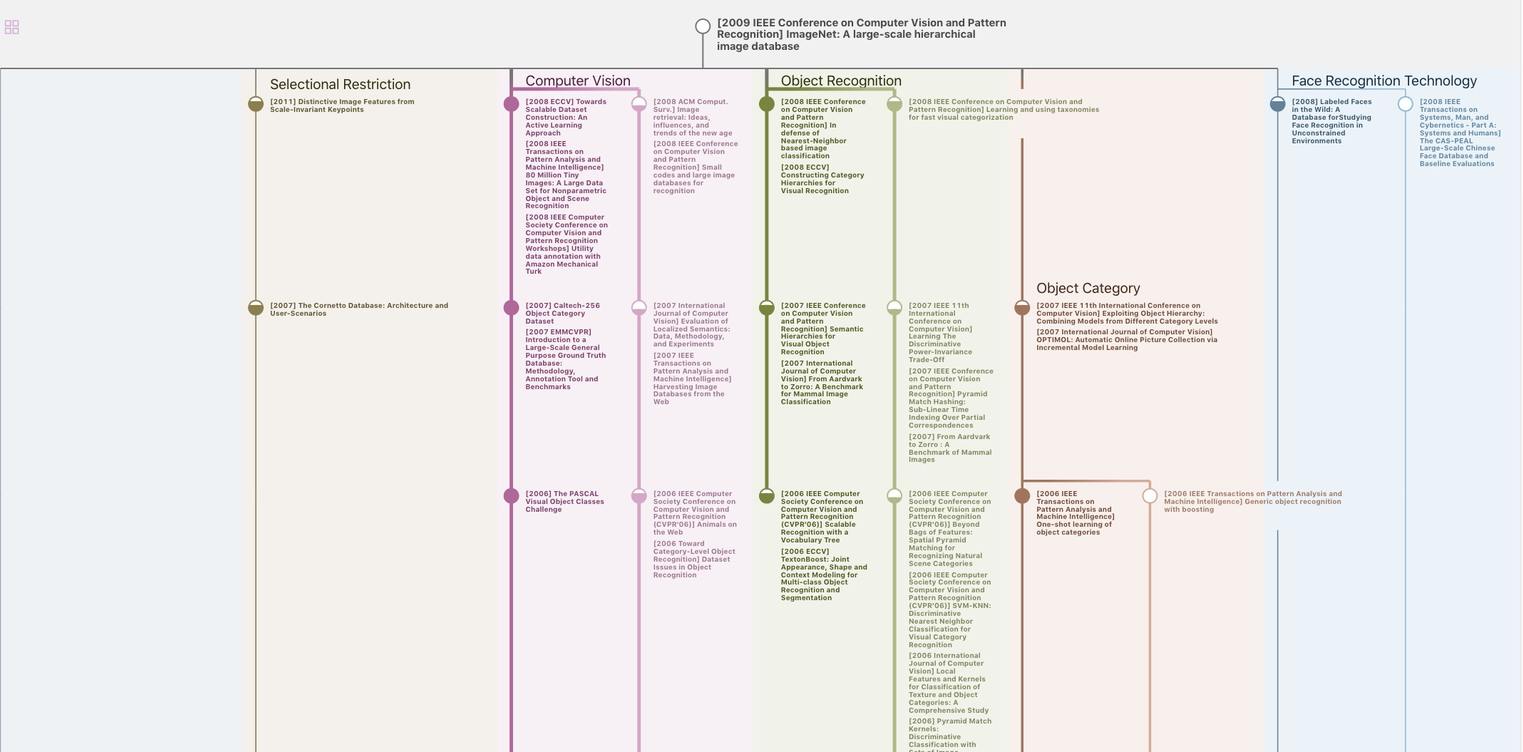
生成溯源树,研究论文发展脉络
Chat Paper
正在生成论文摘要