Training Iterative Collective Classifiers with BackPropagation
12th International workshop on mining and learning with graphs, San Francisco, USA(2016)
摘要
We propose a new method for training iterative collective classifiers for labeling nodes in network data. The iterative classification algorithm (ICA) is a canonical method for incorporating relational information into the classification process. Yet, existing methods for training ICA models rely on computing relational features using the true labels of the nodes. This method introduces a bias that is inconsistent with the actual prediction algorithm. In this paper, we introduce a variant of ICA, ICA with back-propagation (BPICA) as a procedure analogous to recurrent neural network prediction, which enables gradient-based strategies for optimizing over model parameters. We demonstrate that by training BPICA, we more directly optimize the training loss of collective classification, which translates to improved accuracy and robustness on real network data. This robustness enables effective collective classification in settings where local classification is very noisy, settings that previously were particularly challenging for ICA and variants.
更多查看译文
AI 理解论文
溯源树
样例
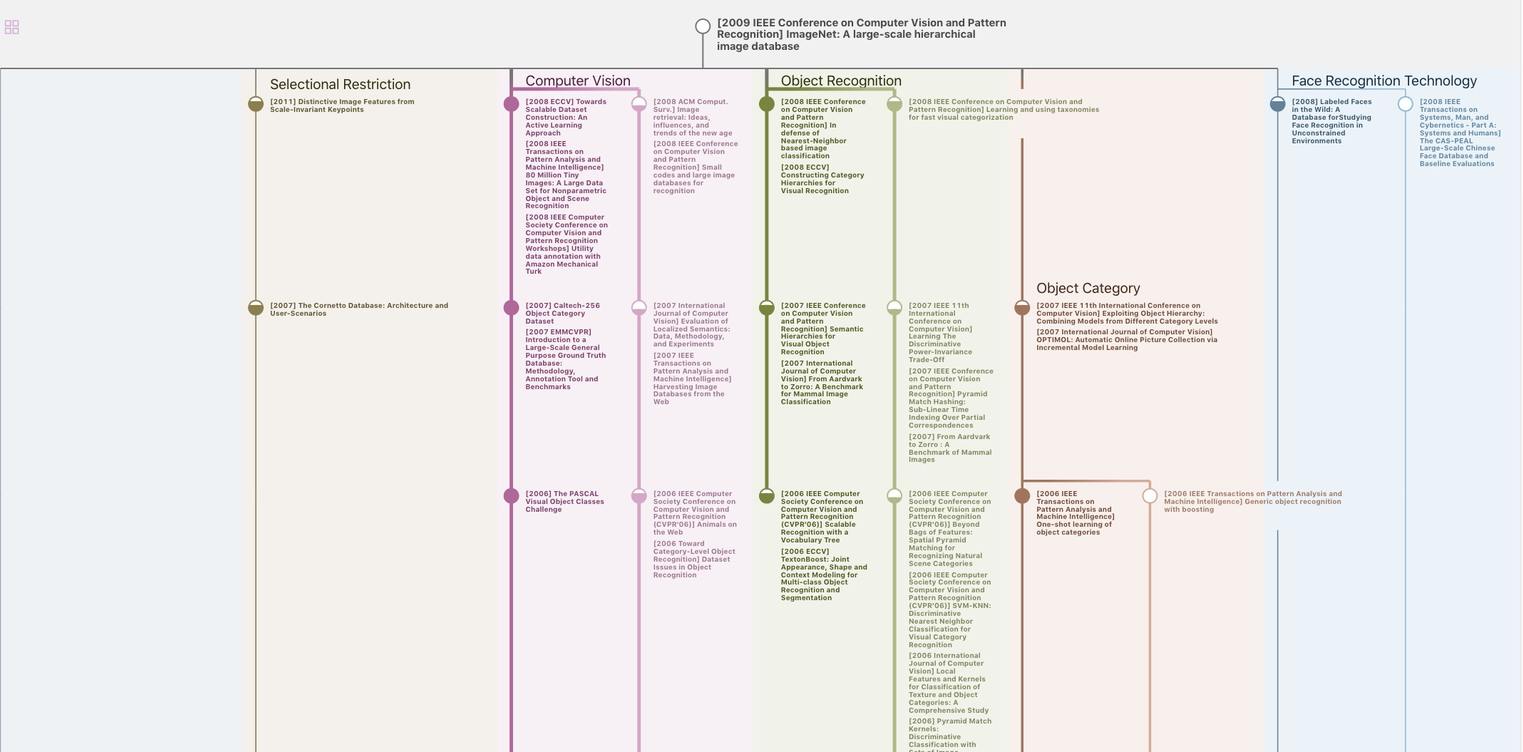
生成溯源树,研究论文发展脉络
Chat Paper
正在生成论文摘要