Evolving Foraging Behaviors
semanticscholar(2009)
摘要
Insects are particularly good at cooperatively solving multiple complex tasks. Some such tasks, such a foraging for food far away from the nest or clustering objects into piles, can be solved through relatively simple behaviors in combination with communication mechanisms using pheromones. As task complexity increases, however, it may become difficult to determine the proper simple rules which yield the desired emergent cooperative behavior; or to know if any such rules exist at all. For such tasks, machine learning techniques like evolutionary computation (EC) may prove a valuable approach to searching the space of possible rule combinations. As a first step towards this goal, we present a proof of concept experiment designed to show that learning techniques can successfully discover good performing foraging strategies which use only pheromone information. The significant contributions of this work are twofold. First, this paper presents a successful approach to learning both the pheromone-depositing and movement-decision-making aspects of an ant colony foraging strategy. Previous work has mainly described methods to learn only movement-decision-making. Second, this paper presents the first learning method which solely uses pheromone information rather than, as was done in previous work, providing hand-coded procedures for returning to the nest. We performed four experiments to demonstrate the efficacy of EC to learning multipheromone problems. Our experimental substrate was a toroidal grid environment with a single nest and point food source, and between 50 and 500 ants. For each approach, we drew a sample of ten runs, and applied a Welch two-sample statistical test at 95%. In the experiments we used the ECJ1 evolutionary computation system, and new multiagent simulation library, MASON, introduced in another paper submitted to this workshop. To evolve ant behaviors, we used strongly-typed genetic programming (GP) [Koza 1992]2. In the common form of genetic programming, which we adopted, evolutionary individuals (candidate solutions) use a parse tree structure representation. Leaf nodes in the parse tree return parameters representing external state values for the ant. Internal nodes in the tree return functions applied to the return values of their subtrees. Crossover swaps subtrees among individuals. In strongly-typed GP, type constraints are placed on which nodes may be added as children of other nodes: we used strong typing
更多查看译文
AI 理解论文
溯源树
样例
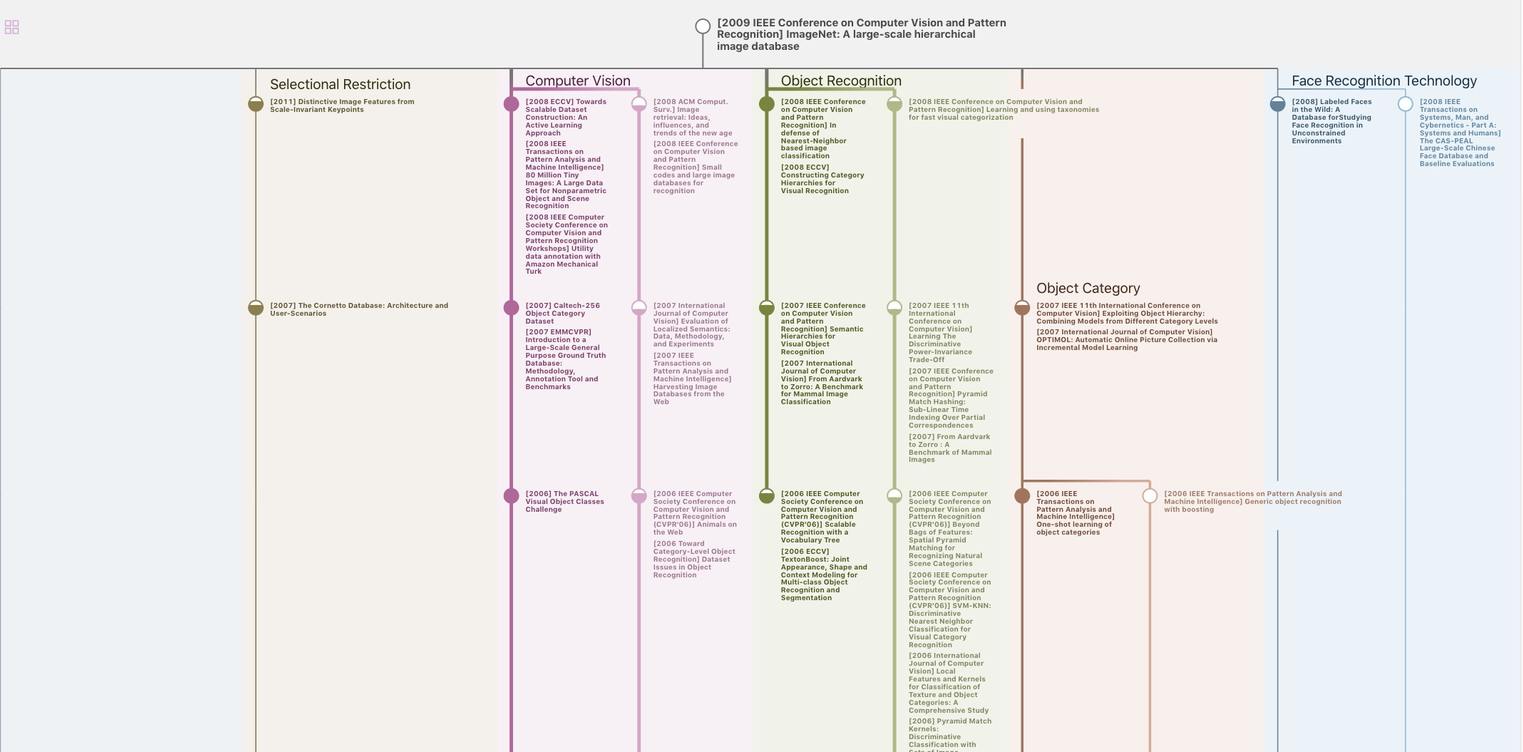
生成溯源树,研究论文发展脉络
Chat Paper
正在生成论文摘要