TRAFFIC SCENE ANOMALY DETECTION LUI CAI AN A project report submitted in partial fulfilment of the requirements for the award of Bachelor of Engineering (Hons.) Software Engineering
semanticscholar(2017)
摘要
Abstracting and inspecting meaningful activities from long hour video is very challenging. In the traditional approach in video analytic and anomaly detection, it applies ruled-based approach where a set of rules have been predefined as the pool of meaningful events that wanted to be detected. By using the ruled-based approach, it limits the detection performance, often triggers false alarm, require extensive configuration setup for each particular scenario and require heavy maintenance after setup. To overcome the limitation of traditional approach, we propose deep learning approach via data-driven approach using non-labelled data, training a spatiotemporal autoencoder model by feeding with normal traffic scene dataset. The autoencoder model consists of Convolutional Neural Network (CNN) and Long Short-Term Memory (LSTM) for learning spatial and temporal characteristics in video dataset. This unsupervised approach able to minimize and reduce dependency on human, where only limited human supervision is required. By using the Plus Highway dataset, the result shows the model able to perform anomaly detection.ing and inspecting meaningful activities from long hour video is very challenging. In the traditional approach in video analytic and anomaly detection, it applies ruled-based approach where a set of rules have been predefined as the pool of meaningful events that wanted to be detected. By using the ruled-based approach, it limits the detection performance, often triggers false alarm, require extensive configuration setup for each particular scenario and require heavy maintenance after setup. To overcome the limitation of traditional approach, we propose deep learning approach via data-driven approach using non-labelled data, training a spatiotemporal autoencoder model by feeding with normal traffic scene dataset. The autoencoder model consists of Convolutional Neural Network (CNN) and Long Short-Term Memory (LSTM) for learning spatial and temporal characteristics in video dataset. This unsupervised approach able to minimize and reduce dependency on human, where only limited human supervision is required. By using the Plus Highway dataset, the result shows the model able to perform anomaly detection.
更多查看译文
AI 理解论文
溯源树
样例
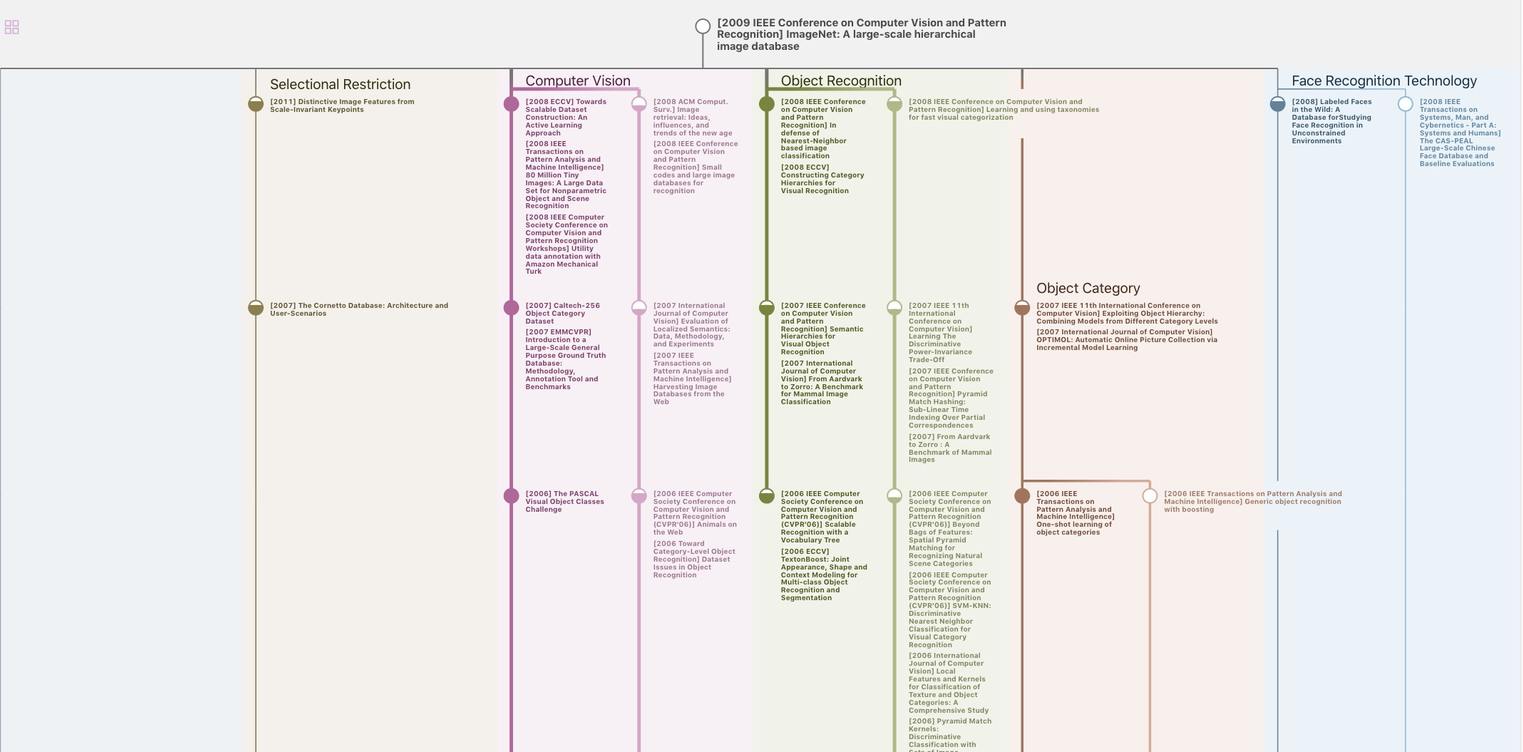
生成溯源树,研究论文发展脉络
Chat Paper
正在生成论文摘要