1 Finding Mnemo : Hybrid Intelligence Memory in a Crowd-Powered Dialog System
semanticscholar(2018)
摘要
While dialog systems can provide a more powerful and natural way to interact with computational tools [Allen et al. 2001a; Allen et al. 2001b], robustly understanding discourse in natural language is beyond the scope of current automated approaches. By leveraging crowds of human workers to respond to end-user queries, crowd-powered dialog systems make it possible to create working systems today, while simultaneously generating data to help train future machine learning (ML) based approaches [Lasecki et al. 2013; Huang et al. 2015; Huang et al. 2016; Huang et al. 2018]. The crowds behind these systems are constantly changing, and no single worker can be relied on to be present between multiple conversational sessions. As a result, context can be lost when interactions span multiple sessions and take place over longer time scales. To provide effective replies, these crowd-powered methods need to maintain consistency over time through a shared conversational context, such as a chat history [Fono and Baecker 2006; Baecker et al. 2007]. However, having workers scroll through a long history log is costly (in terms of time) and may reduce workers’ ability to participate in the conversations in real-time. Moreover, crowd-powered systems fail to map information from past dialogs to present ones, leading to a lack of contextual memory about the user and unnecessary repetition of information across conversations [Lasecki and Bigham 2013]. There is currently no way to extract concise, conversational context from those dialogs. However, people naturally curate between-session context without thinking about it (e.g., memories about their conversations and conversational partners) and can recall information relevant to current topics with relative ease, even over long time spans [Clark et al. 1991]. Unfortunately, querying crowd workers to identify this context is diffcult because this is a subconsious process that does not require explicit effort. But, what if we could tease out these latent mental models of information-saving that we innately build and instantiate this storage in our dialog systems? We propose a methodology for note generation for conversational context maintenance by saving and aggregating human-generated notes from goal-oriented dialogs. We implement and evaluate our approach in Mnemo, a crowd-powered dialog plug-in that allows crowd workers to read dialogs and predict, curate, and save critical information into notes that will be relevant for future conversations, which is not a capability of existing crowdsourced summarization techniques. Our fndings show that combining worker-generated notes (which would be hard or impossible to do with automatically extracting summaries) with aggregation methods that act as tunable “knobs” allow collective responses to outperform individuals’ responses.
更多查看译文
AI 理解论文
溯源树
样例
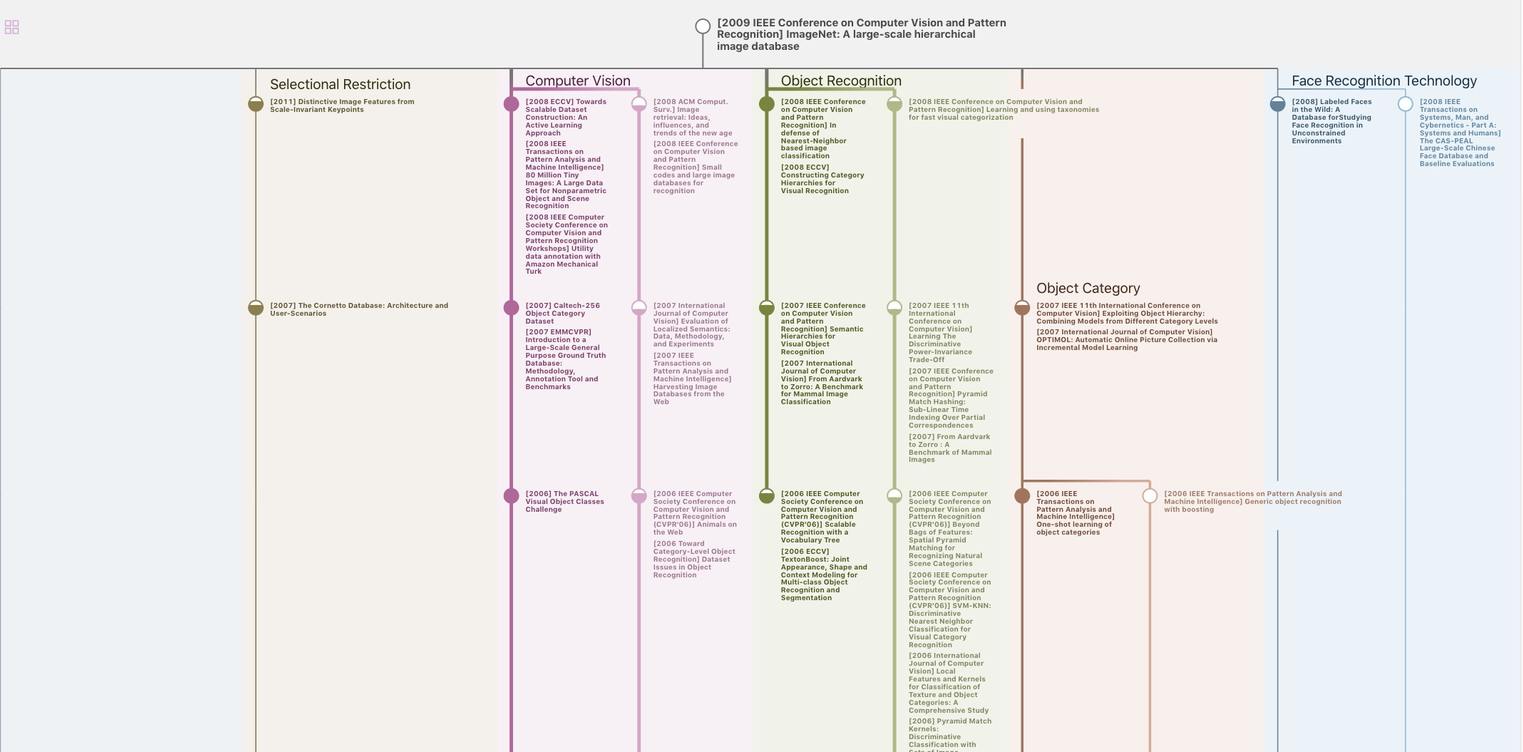
生成溯源树,研究论文发展脉络
Chat Paper
正在生成论文摘要