Machine learning and intelligent optimization in tourism and hospitality : “ Rocket Science ” without the need of scientists
Proceedings of the VLDB Endowment(2019)
摘要
Like most businesses, tourism and hospitality are undergoing a phase of disruptive innovation caused by the wider adoption of computers, networks and, above all, sophisticated and powerful algorithms. Algorithms automate business processes in a partial or total manner, by starting from repetitive and simple tasks but progressively reaching also more complex and “creative” tasks, traditionally associated with human decision making. The concepts of “automated creativity” or “automated business innovation” sound like contradictions. We like to think that only human people can discover truly innovative ways of solving problems and radically improving business performance. In this chapter we summarize two theoretical advancements in the past years which permit this disruptive innovation: machine learning and intelligent optimization. Managers and decision makers reach decisions by some level of anticipation (expectation, prediction) of the effects of different choices. Even if not explicit, these decisions are based on a series of “What if?” questions, with answers given by expertise, gut feeling, or some level of logical and mathematical modelling. Machine learning (ML), or learning from data, is a theory for deriving flexible models by starting only from the data produced by the business. The objective of these models is to generalize in a sound manner for cases not already encountered in the past. After a model is available, computers can simulate the effects of millions of possible decisions, by predicting the output (for example the total profit of the hotel), and by creating and selecting one among the best decisions. Intelligent optimization (IO) is this automated process of creating in an intelligent manner a large series of possible decisions, aiming at improving the current way of doing business. In many practical cases, the objectives to reach are not crystal-clear from the beginning. A standard case is that of multiple-objectives optimization (e.g., maximizing customer satisfaction and minimizing costs). Fine-tuning the objectives by properly balancing different desiderata and constraints and by learning about the possibilities is the sign of intelligent managers. Machine learning represents an additional possibility, this time by learning and fine-tuning a proper global and combined objective to be achieved from the decision maker feedback.
更多查看译文
AI 理解论文
溯源树
样例
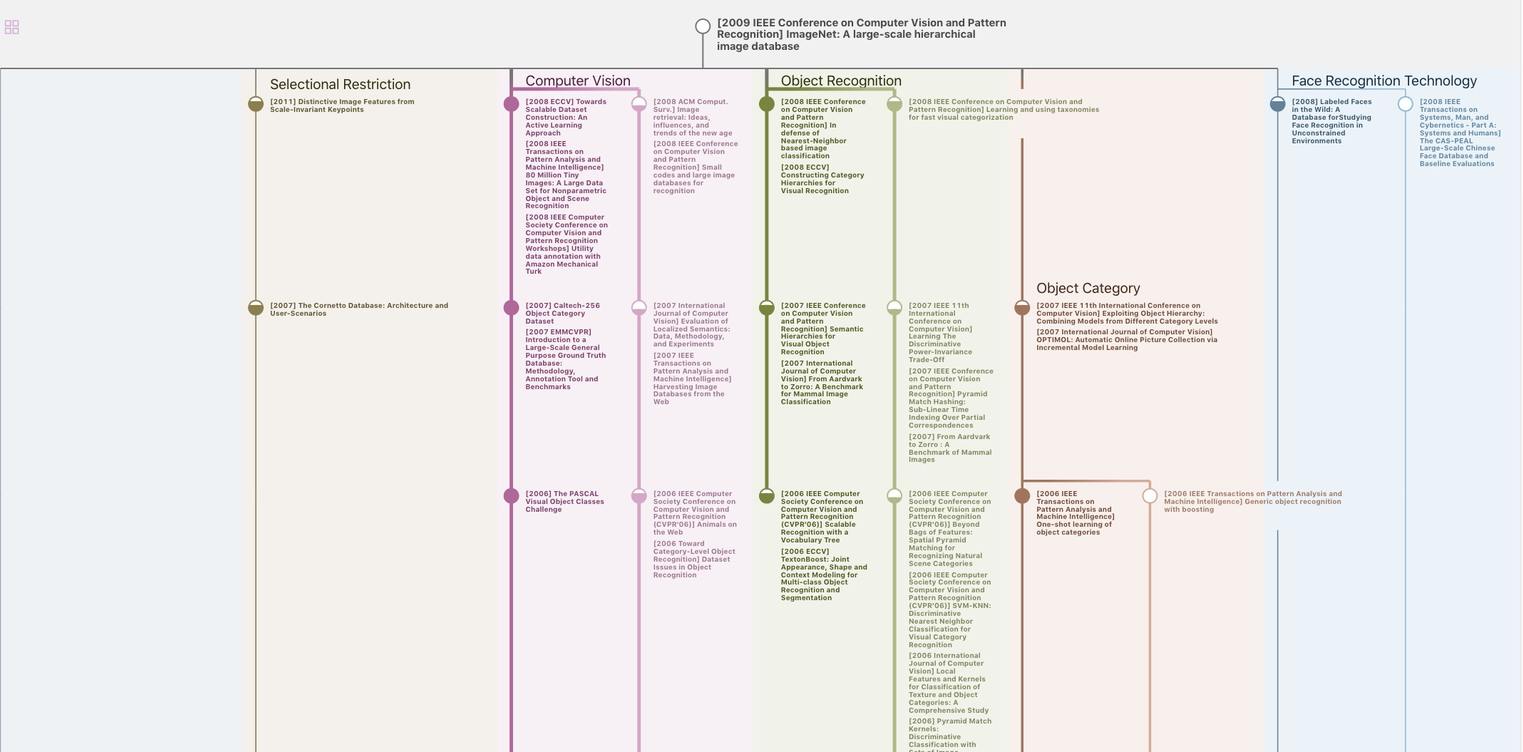
生成溯源树,研究论文发展脉络
Chat Paper
正在生成论文摘要