A Cellular Similarity Metric Induced by Siamese Convolutional Neural Networks
semanticscholar(2016)
摘要
High-throughput microscopy imaging holds great promise for elucidating complex behavior of cells over time. However with increased throughput comes increased difficulty in managing the identity of large numbers of cells over timecourses. This project presents a neural network method based on visual similarity for determining cell identity across frames, which can work in concert with or instead of existing distance-based methods [7]. Using a siamese neural net architecture, we produce a visual similarity distance metric between pairs of input cells. This visual similarity score can be used to assign the identities of cells between frames of a microscopy time course. Training of the siamese neural net is guided by a discriminative loss function developed by Chopra et al [5], which maximizes the energy score between cell pairs labeled as different cells, and minimizes the energy score between cell pairs labeled as the same cell at different time points. Training and test data are selected from labeled timecourses accounting for in total 16.2 million labeled cellpairs. The test accuracy of the model is 97.5% on cells one frame apart, 94.5% on cells separated two frames apart, and 95.0% on mixtures of oneand two-frame separated cells.
更多查看译文
AI 理解论文
溯源树
样例
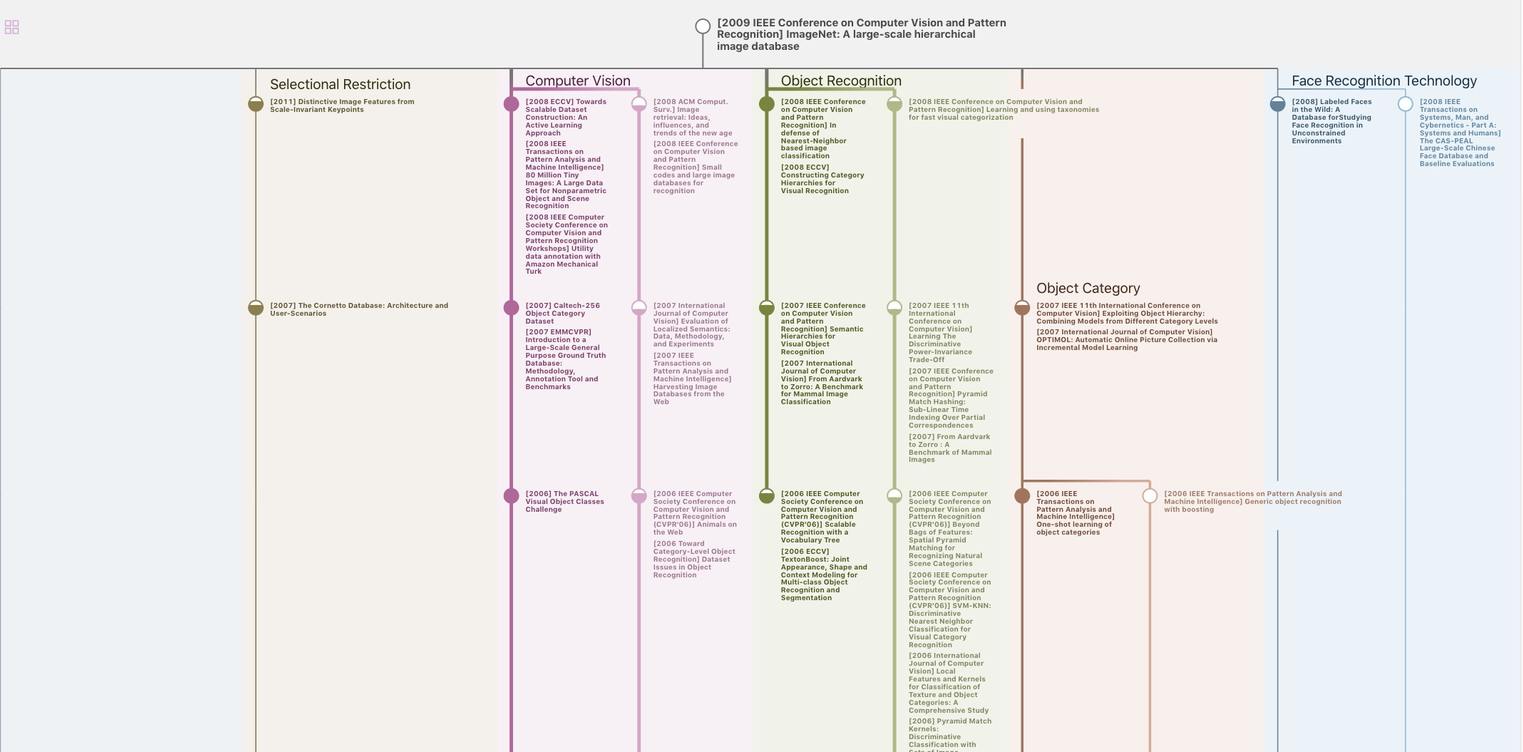
生成溯源树,研究论文发展脉络
Chat Paper
正在生成论文摘要