M L ] 2 3 N ov 2 01 7 Estimating Mixed Memberships with Sharp Eigenvector Deviations
semanticscholar(2017)
摘要
We consider the problem of estimating overlapping community memberships. Existing provable algorithms for this problem either make strong assumptions about the population [33, 16], or are too computationally expensive [3, 15]. We work under the popular Mixed Membership Stochastic Blockmodel (MMSB) [2]. Using the inherent geometry of this model, we link the inference of overlapping communities to the problem of finding corners in a noisy rotated and scaled simplex, for which consistent algorithms exist [12]. We use this as a building block for our algorithm to infer the community memberships of each node. Furthermore, we prove that each node’s soft membership vector converges to its population counterpart. To our knowledge, this is the first work to obtain rate of convergence for community membership vectors of each node, in contrast to previous work which obtain convergence results for memberships of all nodes as a whole. As a byproduct of our analysis, we derive sharp row-wise eigenvector deviation bounds, and provide a cleaning step that improves the performance significantly for sparse networks. We also propose both necessary and sufficient conditions for identifiability of the model, while existing methods typically present sufficient conditions. The empirical performance of our method is shown using simulated and real datasets scaling up to 100,000 nodes.
更多查看译文
AI 理解论文
溯源树
样例
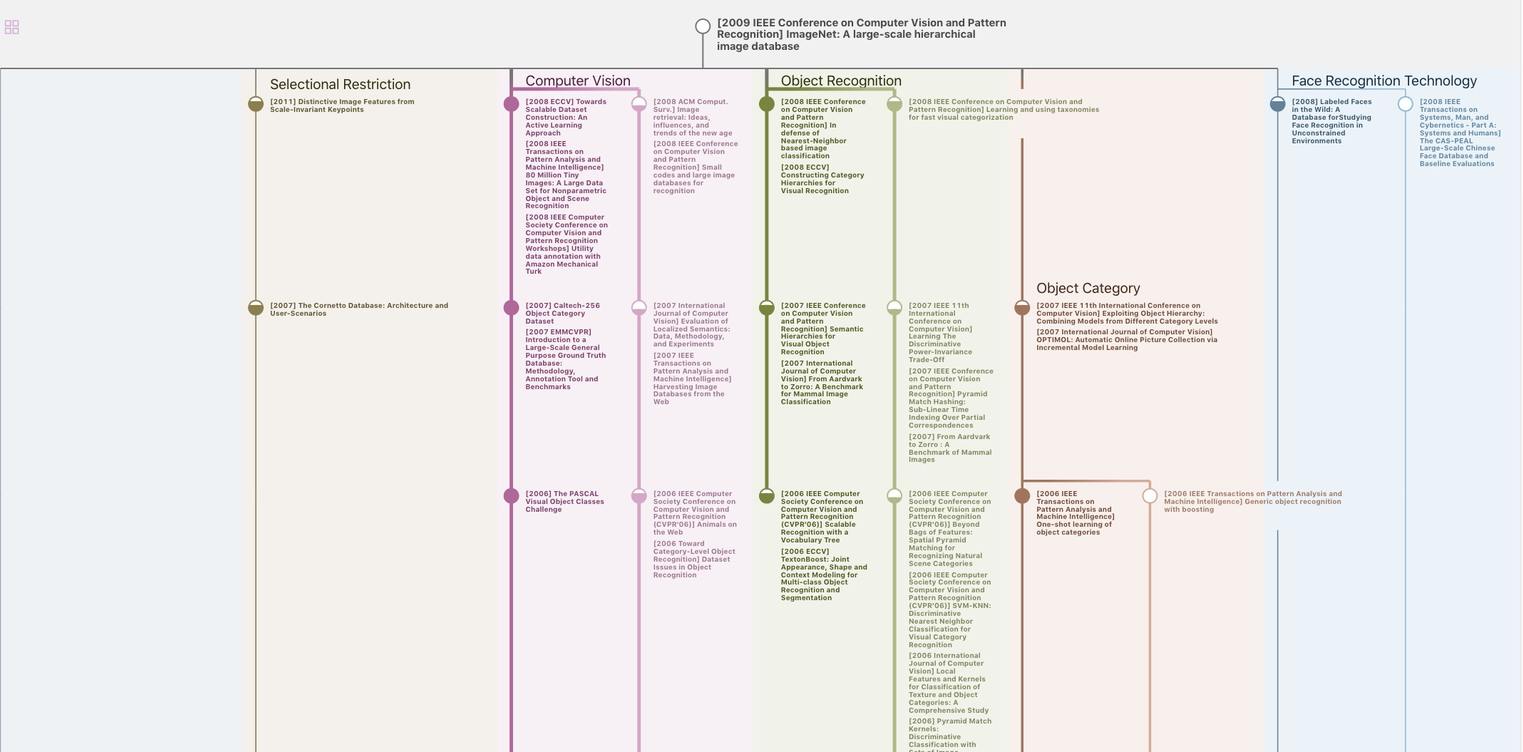
生成溯源树,研究论文发展脉络
Chat Paper
正在生成论文摘要