Translation-Invariant Modulation Spectrum with Application to Communication Signal Interception
semanticscholar(2006)
摘要
In many signal processing applications, such as communications signal interception, the signal’s modulation type is unknown. Accurately identifying the modulation type is therefore a critical first step in monitoring or other use of the communication channel. Past work on automatic identification of modulation type has mostly focused on using a combination of short-term spectral features and automatic classifiers. Systems which use these short-term features (for instance, short-term spectral estimates and their moments) rely upon dynamic classifiers, such as hidden Markov models, to model all longer-term dynamics. In this paper we take the view that time and frequency translation invariance, which is needed in this application, can be gained by also using longer-term signal features. In particular, the modulation spectrum, with an incorporated wavelet transform, can provide the needed invariance, and potentially improve the performance of a subsequent automatic classifier. We test this hypothesis on two common signal databases, one relatively clean and the other noisy, and compare automatic modulation classification systems with and without our proposed long-term features. In all cases, the addition of the long-term features substantially improves performance, as measured by classification error rate. In several cases, our proposed solution has error rates at least 20% better than solutions which only use conventional short-term features. Manuscript received March 9, 2006. This work was partly supported by the Air Force Research Laboratory. S. Sukittanon is with ShotSpotter Inc., Mountain View CA 94025 USA (e-mail: ssukitta@ieee.org) L.E. Atlas is with the Department of Electrical Engineering, University of Washington, Seattle WA 98195-2500 USA. J.W. Pitton and J. McLaughlin are with the Applied Physics Laboratory, University of Washington, Seattle WA 98105-6698 USA. K. Filali is with the Department of Computer Science and Engineering, University of Washington, Seattle WA 98195-2350 USA.
更多查看译文
AI 理解论文
溯源树
样例
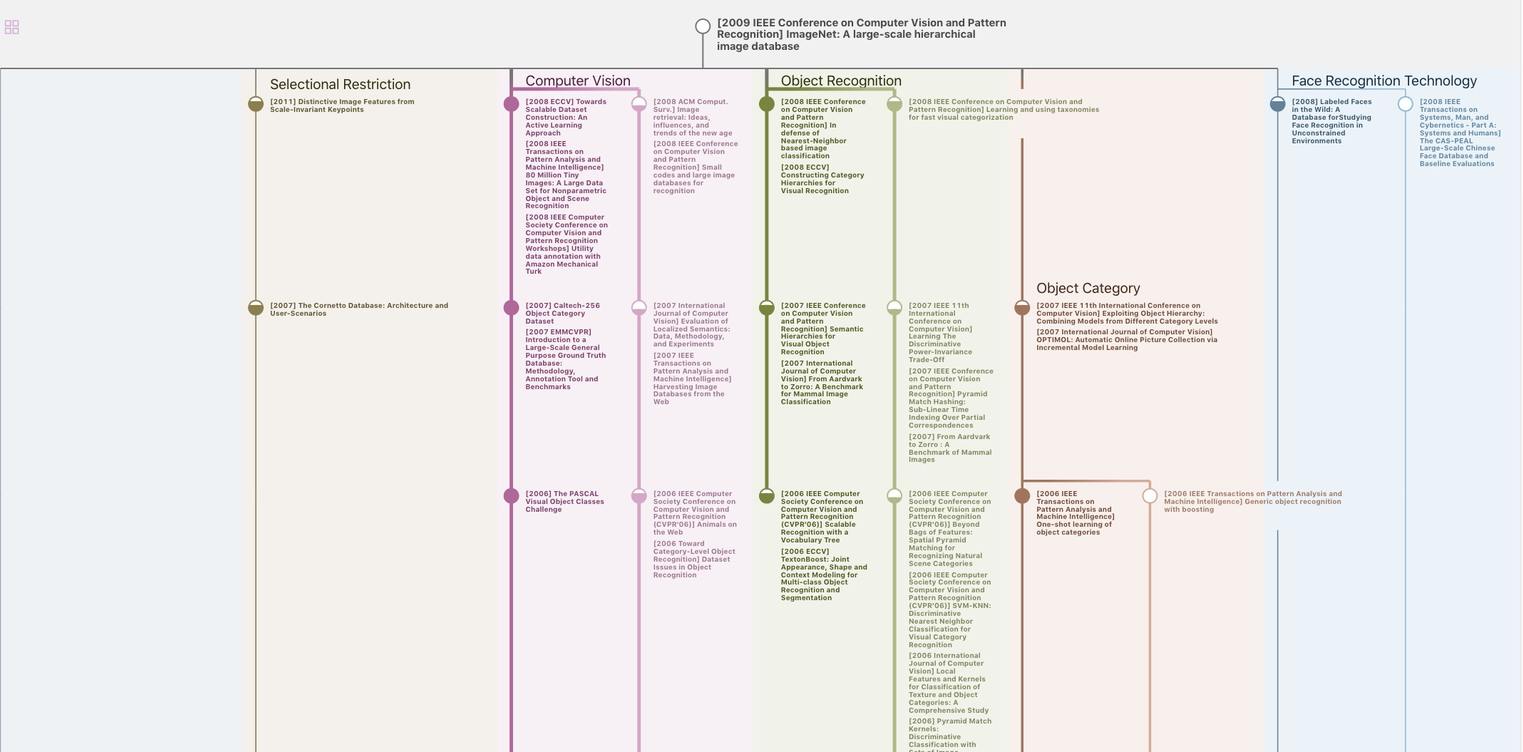
生成溯源树,研究论文发展脉络
Chat Paper
正在生成论文摘要