Graph-based compressed sensing MRI image reconstruction : View image patch as a vertex on graph
semanticscholar(2014)
摘要
INTRODUCTION: Compressed sensing (CS) accelerates magnetic resonance (MR) imaging 1 by under-sampling the k-space data. Although undersampling will introduce aliasing artifacts, high quality images can be reconstructed by enforcing a sparse regularity on them.The sparse representation plays a key role in CS. Previous methods are often based on pre-constructed bases , trained dictionaries 3 or optimized sparsifying transforms . In this work, an emerging graphical model is constructed to capture geometry and structure of a MR image which transform it more regular. Then, wavelet transform is applied on the re-ordered pixels to sparsify the image. METHOD: By breaking an image into overlapped patches and denoting the patches as vertexes and Euclidean distances between patches as edges, a patch-based graph can be constructed. Fig. 1 shows the graph of several patches. Smaller Euclidean distance implies more similarity between the centered pixels. To rearrange pixels to be a smooth signal, a traveling sales man problem is to be solved to find the optimal rearrangement . The corresponding permutation matrix P is applied to pixels before wavelet filtering, which can be expressed as T T Ψ P .The same reordering and filtering procedures can be applied to pixels of other pixels in a patch without increasing difficulty. When the size of an column-wise patch is J , we use 1, , , j j J = R L to denote the reordering operator. The l1 norm is adopted to
更多查看译文
AI 理解论文
溯源树
样例
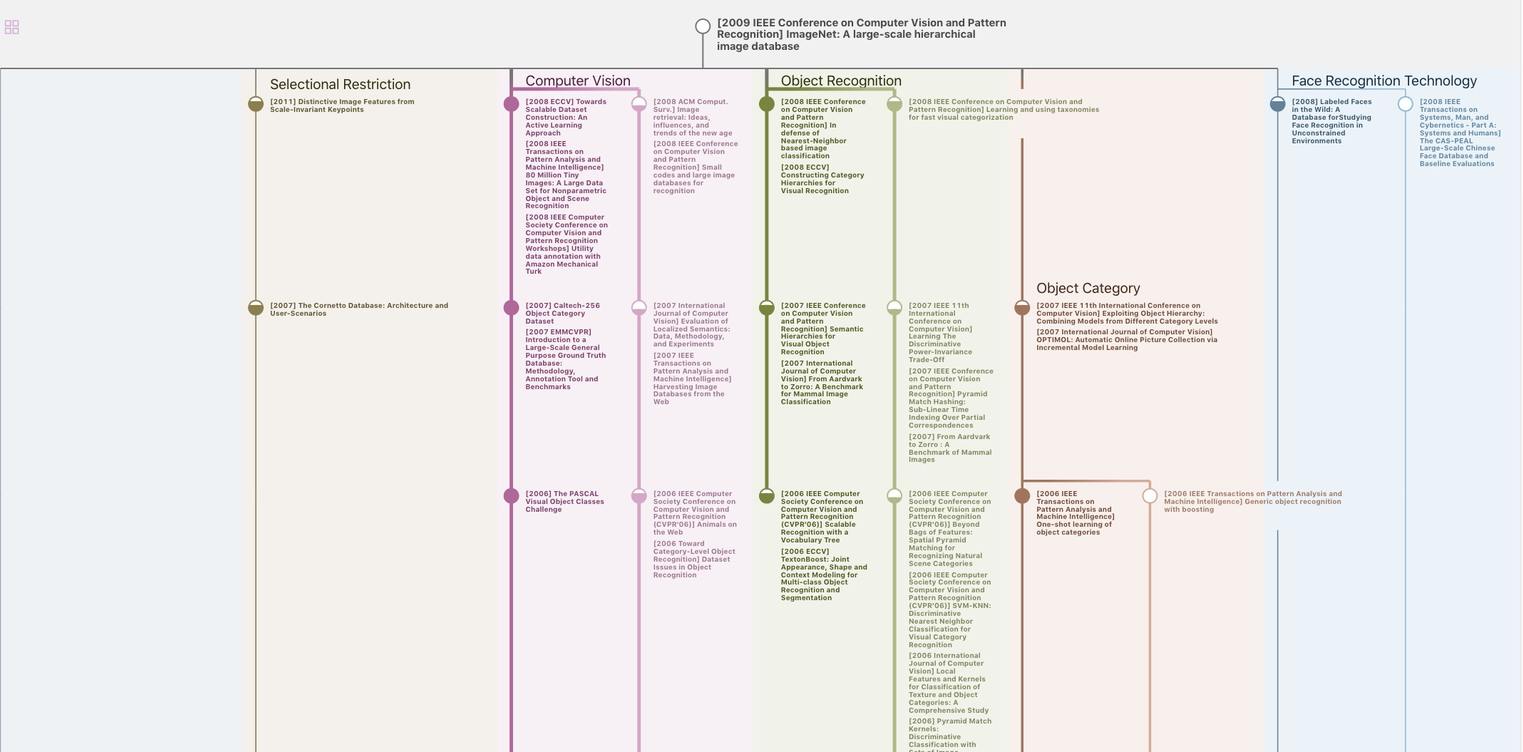
生成溯源树,研究论文发展脉络
Chat Paper
正在生成论文摘要