M ore systematic than claimed : i nsights on the scan tasks
semanticscholar(2018)
摘要
We show that some standard attention-based architectures widely used in Neural Machine Translation as well as a pointer-based variant achieve results on some of the compositional SCAN tasks that are far superior to those reported in Lake & Baroni (2018). We next show that there is high variance in the test accuracy across both random initialization and training duration. We show that ensembling can be used to take advantage of this variance and improve results but that, for many tasks, a large gap remains between ensemble performance and the performance of an oracularly selected single best model. Based on these insights, we suggest some possible directions for future research, emphasizing selection and regularization over the need for more compositional architectures.
更多查看译文
AI 理解论文
溯源树
样例
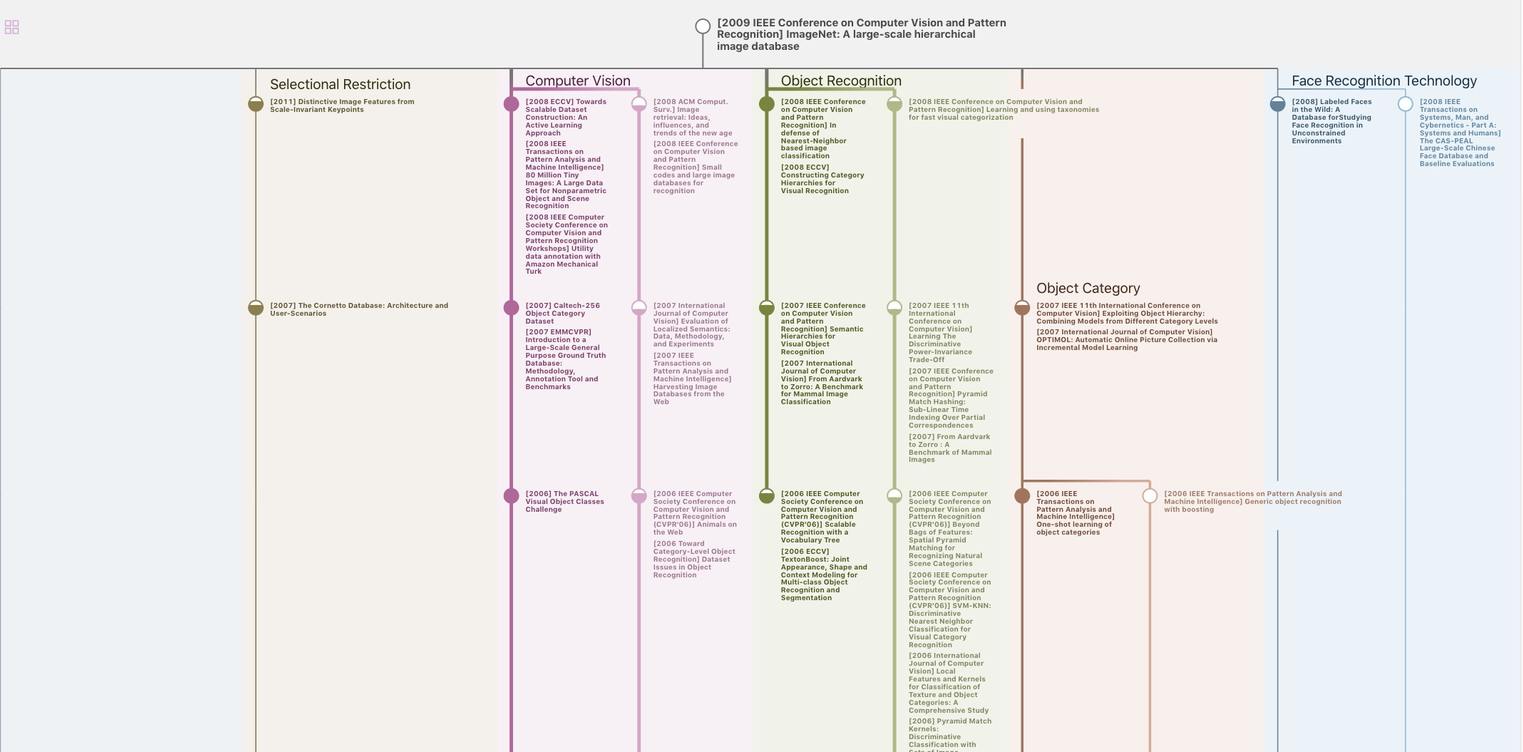
生成溯源树,研究论文发展脉络
Chat Paper
正在生成论文摘要