Extended Object Tracking With Transparency Mitigation
semanticscholar(2018)
摘要
Zusammenfassung: We propose an efficient approach to enhance the measurement likelihood of differentiable star-convex extended target models to mitigate unlikely association processes due to self-occlusion. Our approach allows us to retain an efficient computation of likelihoods and hence the correction step for extended targets while simultaneously penalizing unlikely measurement to target associations. In combination with a filter that is able to process multimodal distributions we find that this leads to a significant increase in tracking performance, in particular during the initialization of the filter. We demonstrate an implementation based on the recently proposed Gaussian process extended target model and show how our redefined likelihood improves real-world tracking performance using automotive LIDAR data.
更多查看译文
AI 理解论文
溯源树
样例
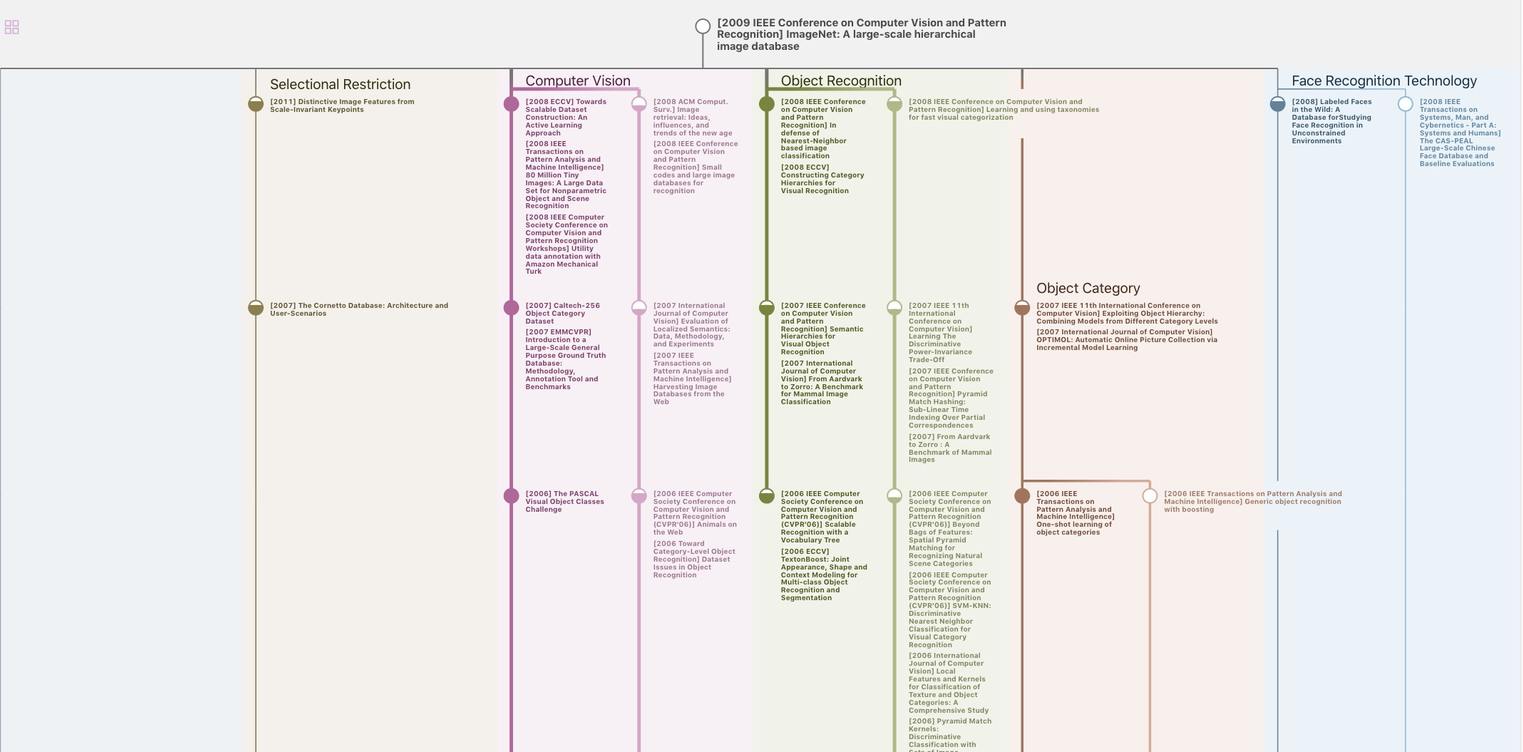
生成溯源树,研究论文发展脉络
Chat Paper
正在生成论文摘要