Multi-label Classification of Single and Clustered Cervical Cells Using Deep Convolutional Networks
semanticscholar(2018)
摘要
Cytology-based screening through the Papanicolaou test has dramatically decreased the incidence of cervical cancer. Convolutional neural networks (CNN) have been utilized to classify cancerous cervical cytology cells but primarily focused on pre-processed nuclear details for a single cell and binary classification of normal versus abnormal cells. In this study, we developed a novel system for multiple label classification with a focus on both nucleus and cytoplasm of single cells and cell clusters. In this retrospective study, we digitalized cervical cytology slides from 104 patients. Based upon the Bethesda system, the established criteria for diagnosing cervical cytology, cells of interest were categorized. With 10-fold cross validation, our CNN algorithm demonstrated 84.5% overall accuracy, 79.1% sensitivity, 89.5% specificity for normal versus abnormal. For 3level classification of normal, low-grade, and high-grade, CNN demonstrated 76.1% overall accuracy. Results show promise on the utility of CNNs to learn cervical cytology. Keywords—cervical cytology; cervical cancer; pap smear; Bethesda system; deep learning; convolutional neural network; digital pathology; whole-slide imaging
更多查看译文
AI 理解论文
溯源树
样例
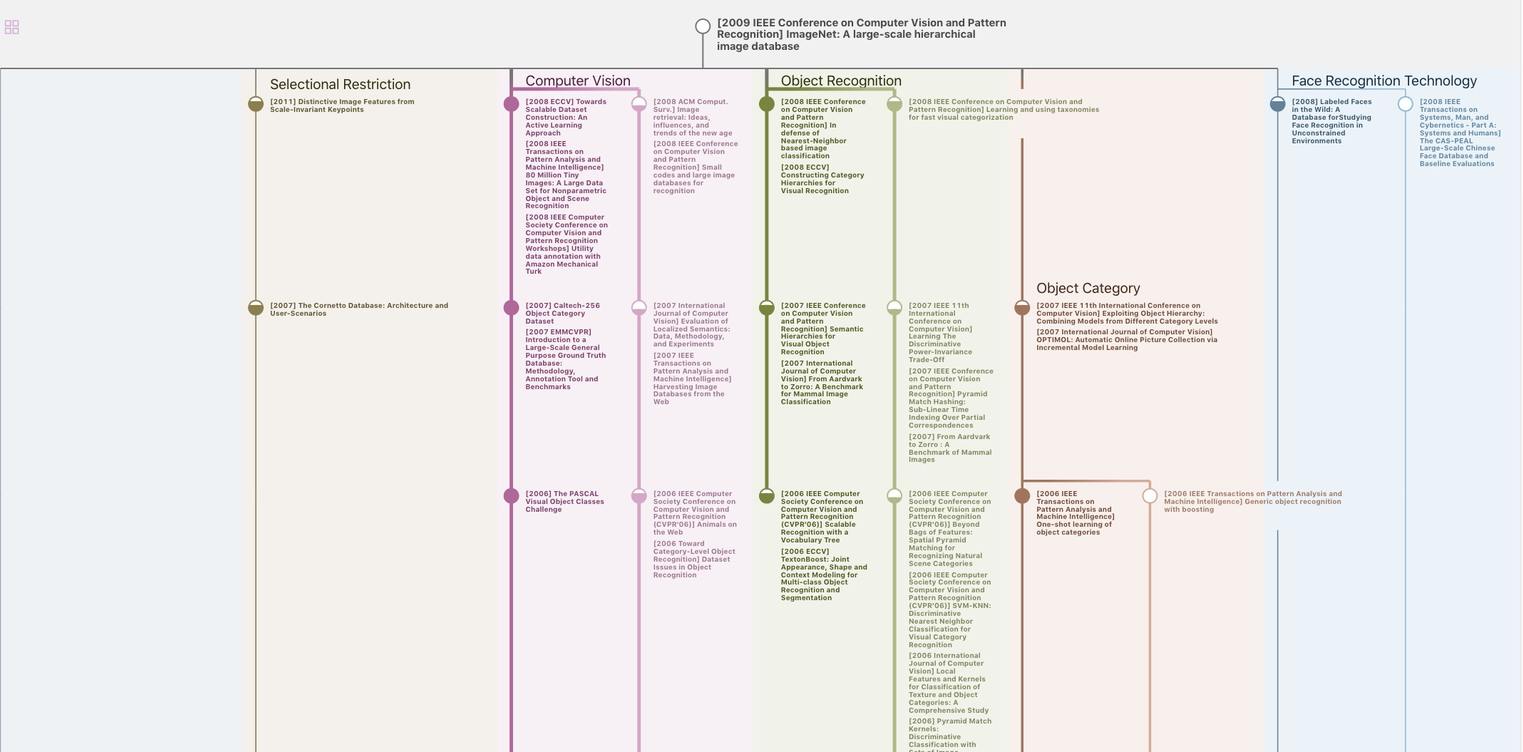
生成溯源树,研究论文发展脉络
Chat Paper
正在生成论文摘要