Deep Deformable Part Models
semanticscholar(2012)
摘要
The deformable parts model (DPM) [6] serves as a key component in most modern state-of-the-art object detection systems. At a high level, the DPM composes a single object model by learning to detect and assemble parts of an object. Most modern systems employing the DPM employ densely computed Histogram of Oriented Gradients [5] features at training time. Despite the success of HOG features in many applications, we believe that more sophisticated features derived from unsupervised feature learning processes can boost DPM performance. Recent work in unsupervised feature learning demonstrates that single neurons in a deep network can discriminate between object classes [10]. However, most published work on such deep learning approaches have succeeded only on simple image classification tasks (e.g. CIFAR, MNIST). In this project, we explore the empirical performance of the deep deformable parts model (D-DPM), which uses deep features in the DPM in lieu of HOG features. We evaluate the efficacy of the D-DPM on the PASCAL VOC 2007 object detection task. To the best of our knowledge, no other work has successfully employed deep features on detection tasks involving datasets with as much variation as PASCAL.
更多查看译文
AI 理解论文
溯源树
样例
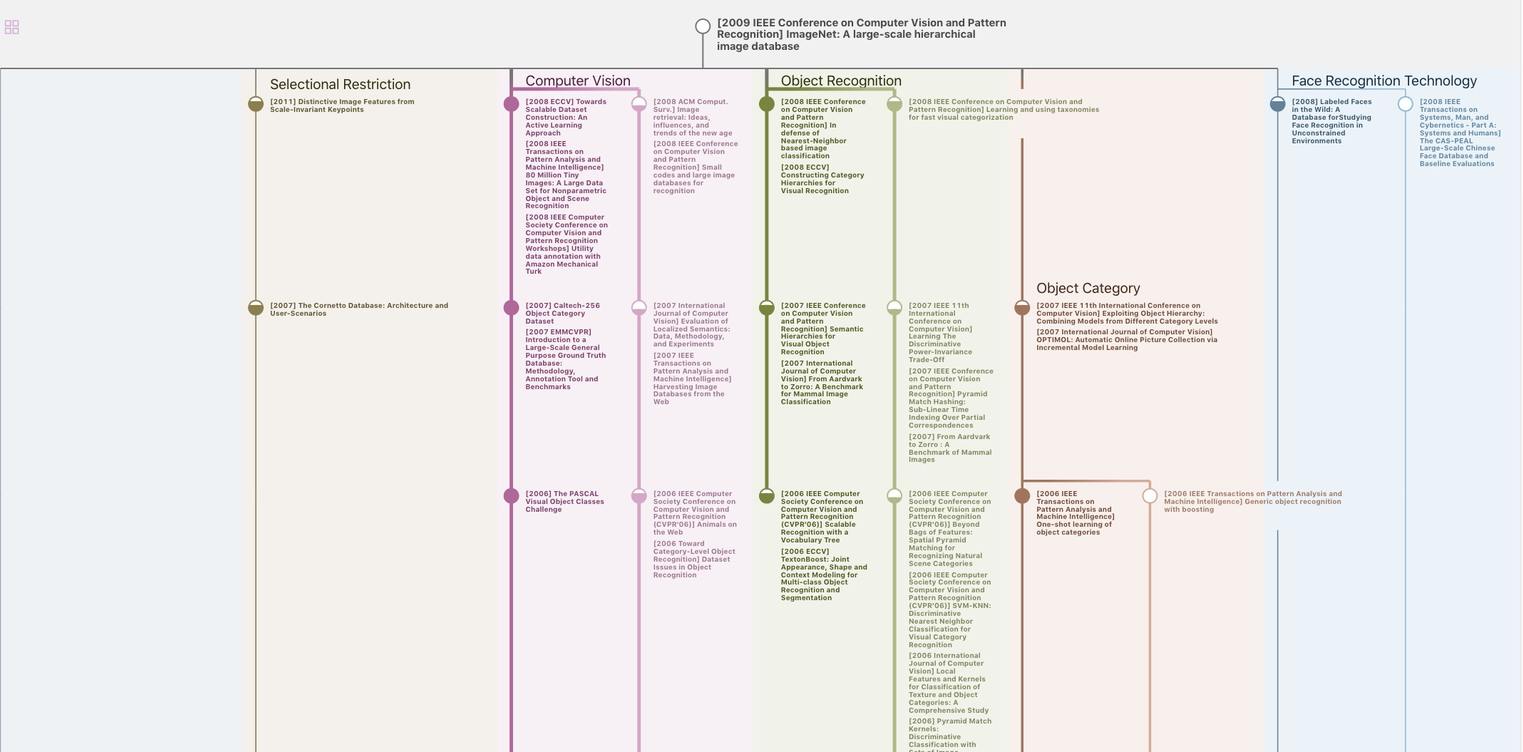
生成溯源树,研究论文发展脉络
Chat Paper
正在生成论文摘要